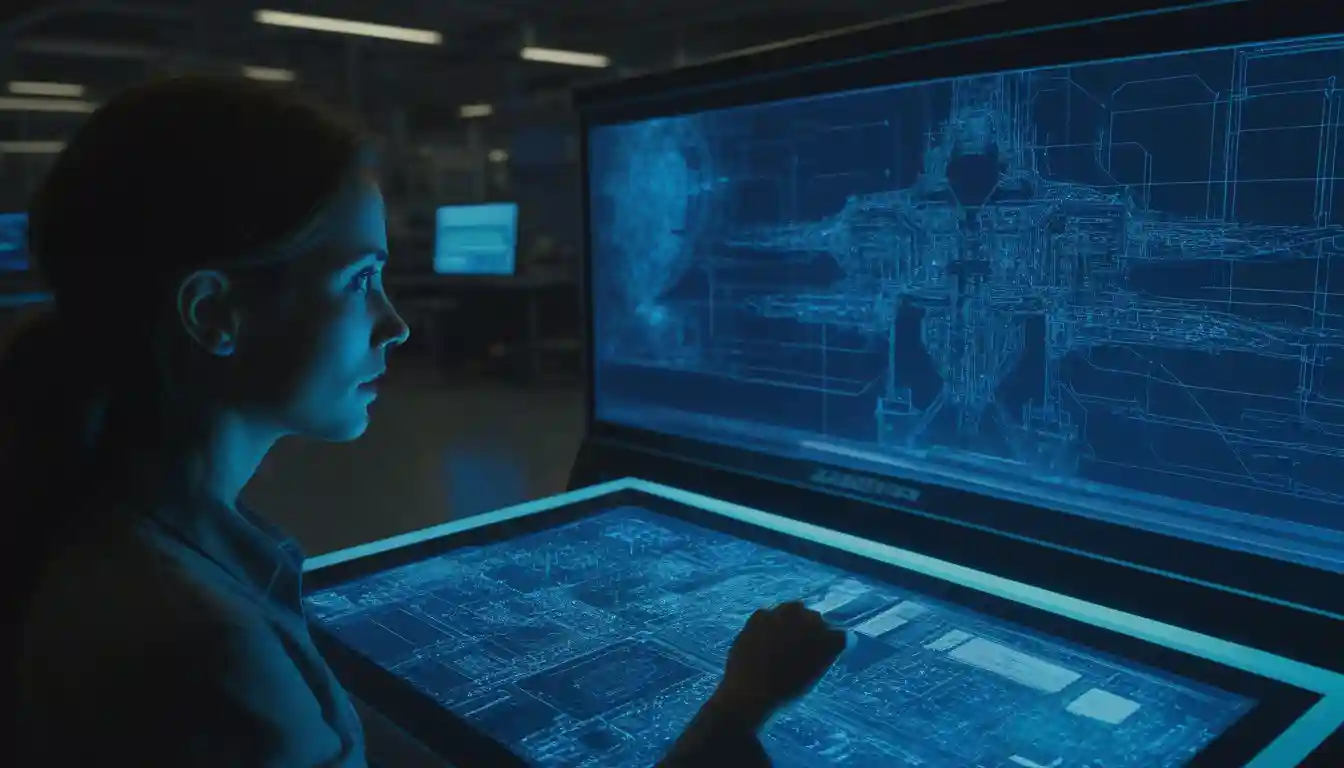
Google's AlphaChip Revolutionizes Chip Design, Outpacing Human Experts
Google DeepMind's AlphaChip: Revolutionizing Computer Chip Design with AI
Google DeepMind has unveiled groundbreaking details about its artificial intelligence system, AlphaChip, which is transforming the landscape of computer chip development. This innovative AI solution dramatically accelerates and enhances the chip design process, with its creations already powering Google's cutting-edge AI accelerators.
Building upon their 2021 Nature study, Google DeepMind has now shared in-depth information about AlphaChip's capabilities. The system leverages reinforcement learning algorithms to rapidly generate optimized chip layouts, a task that traditionally required weeks or months of human expertise.
AlphaChip's impact is already evident in Google's hardware. The AI system has been instrumental in designing chip layouts for the last three generations of Google's Tensor Processing Unit (TPU) AI accelerators. Its performance has shown remarkable improvement with each iteration. For the TPU v5e, AlphaChip successfully placed 10 blocks and achieved a 3.2% reduction in wire length compared to human experts. This performance leap continued with the current 6th generation TPU, codenamed Trillium, where AlphaChip placed 25 blocks and reduced wire length by an impressive 6.2%.
The system's approach mirrors that of DeepMind's famous AlphaGo and AlphaZero, treating chip layout as a strategic game. AlphaChip utilizes a sophisticated graph neural network to understand and optimize the intricate relationships between connected components, allowing it to generalize across various chip designs.
Key Takeaways:
-
AI-driven chip design: AlphaChip uses reinforcement learning to create optimized chip layouts in hours, outperforming human designers who typically require weeks or months.
-
Proven performance: The system has been successfully employed in designing Google's last three generations of TPU AI accelerators, showing consistent improvement.
-
Widespread adoption: Beyond Google, other tech giants like MediaTek are expanding AlphaChip's capabilities for developing advanced chips, including the Dimensity Flagship 5G used in Samsung smartphones.
-
Open-source initiative: Google DeepMind has released open-source resources for AlphaChip, including a software repository and pre-trained model, enabling external researchers to leverage and build upon this technology.
-
Future potential: DeepMind envisions AI optimizing the entire chip design cycle, from computer architecture to manufacturing, potentially revolutionizing the semiconductor industry.
Deep Analysis:
AlphaChip represents a paradigm shift in the semiconductor industry, where AI is not just the end product but also the means of production. This creates a virtuous cycle: AI-designed chips power more advanced AI models, which in turn can design even better chips.
The system's ability to reduce wire length by 6.2% in the latest TPU generation is particularly significant. In chip design, shorter wire lengths translate to reduced delays, lower power consumption, and improved overall performance. This efficiency gain could lead to more powerful and energy-efficient devices across the tech spectrum.
AlphaChip's expanding capabilities are equally noteworthy. Its progression from placing 10 blocks in TPU v5e to 25 blocks in Trillium demonstrates rapid learning and adaptation. This scalability suggests that future iterations could potentially design entire chip layouts with minimal human intervention.
The open-sourcing of AlphaChip resources is a strategic move by Google DeepMind. By allowing external researchers to pre-train the system on various chip blocks, they're fostering innovation and potentially accelerating advancements in chip design across the industry. This collaborative approach could lead to unforeseen breakthroughs and applications of AI in hardware design.
Did You Know?
-
AlphaChip treats chip layout as a game, similar to how AlphaGo approached the game of Go. It starts with a blank grid and places circuit components one at a time until the layout is complete.
-
The system uses a novel "edge-based" graph neural network to learn relationships between interconnected chip components, allowing it to generalize across different designs.
-
Google DeepMind has provided a pre-trained model checkpoint trained on 20 TPU blocks, allowing researchers to jumpstart their own chip design projects using AlphaChip technology.
-
The open-source repository released by Google DeepMind can fully reproduce the methods described in their original Nature study, promoting transparency and reproducibility in AI research.
-
While AlphaChip has shown impressive results, DeepMind researchers recommend pre-training on custom, application-specific blocks for optimal performance in specialized chip designs.