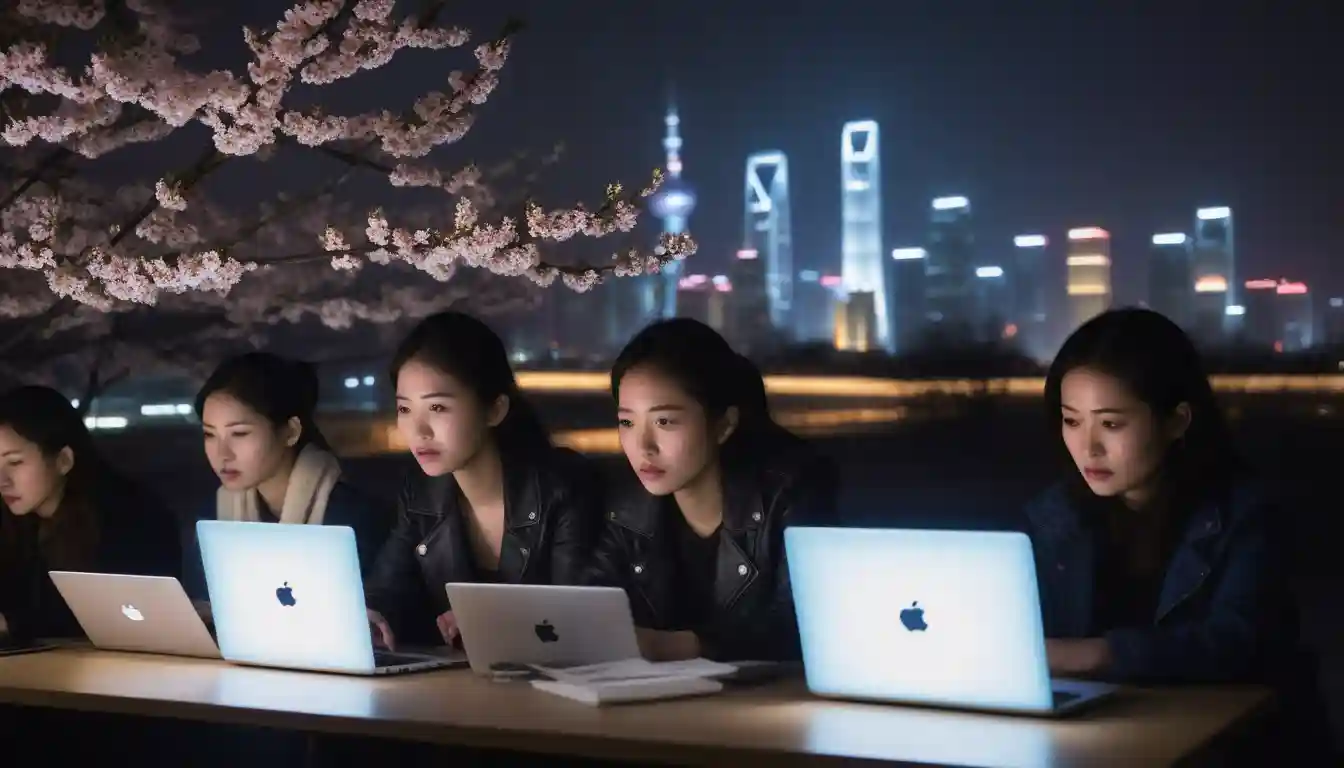
China's Gen AI Startups Struggle: Stagnation Hits Amid Global Monetization Challenges
Chinese Generative AI Startups Face Stagnation Amid Monetization Challenges
In the rapidly evolving landscape of artificial intelligence, six Chinese generative AI startups, often dubbed the "six tigers," are facing a period of stagnation, mirroring a global struggle experienced by even the most prominent players like OpenAI. These startups—智谱AI (Zhipu AI), 百川智能 (Baichuan Intelligence), 零一万物 (Zero-One Things), 月之暗面 (Dark Side of the Moon), Minimax, and 驭跃星辰 (YuYueXingChen)—are grappling with the challenge of monetizing their large language models (LLMs), a task that has proven difficult both domestically and abroad. The Chinese AI industry, once bustling with optimism and rapid growth, now finds itself at a crossroads, with these startups exhibiting varying degrees of uncertainty and strategic redirection.
Key Takeaways:
-
Strategic Retrenchment and Workforce Reduction: Some startups, like Zhipu AI, have reduced their workforce post-expansion and are preparing for an IPO. Others, like Baichuan Intelligence, have halted pre-training initiatives and consumer-facing services, indicating a period of confusion and reassessment. Minimax has locked its focus on core business areas, while startups like Zero-One Things and Dark Side of the Moon have gone silent, marking a stark contrast to their earlier high-profile activities.
-
Expansion in Uncertain Times: In a contrarian move, YuYueXingChen continues to expand, buoyed by confidence from its backing by larger companies. However, the future remains unclear for this and other startups, as they navigate an increasingly competitive and saturated market.
-
Investor Skepticism: Chinese investors, mirroring global sentiments, predict that the best outcome for these startups might be acquisition by larger tech companies. The high costs of R&D, coupled with the struggle to find profitable business models, make long-term independence challenging.
-
Global Parallel: These issues are not isolated to China. Even in the U.S., AI leaders like OpenAI are facing similar monetization struggles. The lack of a "killer app" that can drive mass adoption in both consumer and enterprise markets remains a significant barrier.
Deep Analysis: The stagnation faced by these Chinese AI startups underscores the broader challenges in the generative AI industry. On the consumer side (To C), the absence of a transformative application that could propel widespread adoption has resulted in tepid engagement and difficulties in monetization. Despite initial excitement around AI-driven tools like chatbots and content generators, they have not reached a level of indispensability that would justify large-scale consumer spending. Moreover, growing concerns over privacy and data security have further dampened enthusiasm, making it harder for companies to gain traction in this market.
In the enterprise market (To B), the challenges are equally daunting. Businesses require AI models that are not only powerful but also tailored to their specific needs. This high demand for customization, combined with the current limitations of LLMs in performing complex, domain-specific tasks, has made it difficult for these models to deliver the precision and reliability that enterprises seek. The need for extensive customization adds to the cost and complexity, making it an unattractive proposition for many businesses.
Furthermore, the competitive landscape is exacerbated by price wars and a lack of strong technological differentiation among many Chinese AI companies. As a result, the market is becoming increasingly saturated, with many firms finding it hard to stand out or offer unique value propositions. This has led to a scenario where companies are competing on price rather than innovation, further squeezing margins and making it difficult to sustain operations.
Did You Know? Despite the current challenges, the concept of large language models (LLMs) is not new. The roots of LLMs can be traced back to the development of early AI models that focused on natural language processing (NLP). However, it wasn't until the advent of transformer architectures, such as Google's BERT and OpenAI's GPT series, that LLMs began to show significant promise. These models leverage vast amounts of data and computational power to generate human-like text, enabling a wide range of applications from automated customer service to creative content generation. Yet, the journey from technological breakthrough to profitable business model remains fraught with challenges, as evidenced by the current struggles of both Chinese and global AI startups.