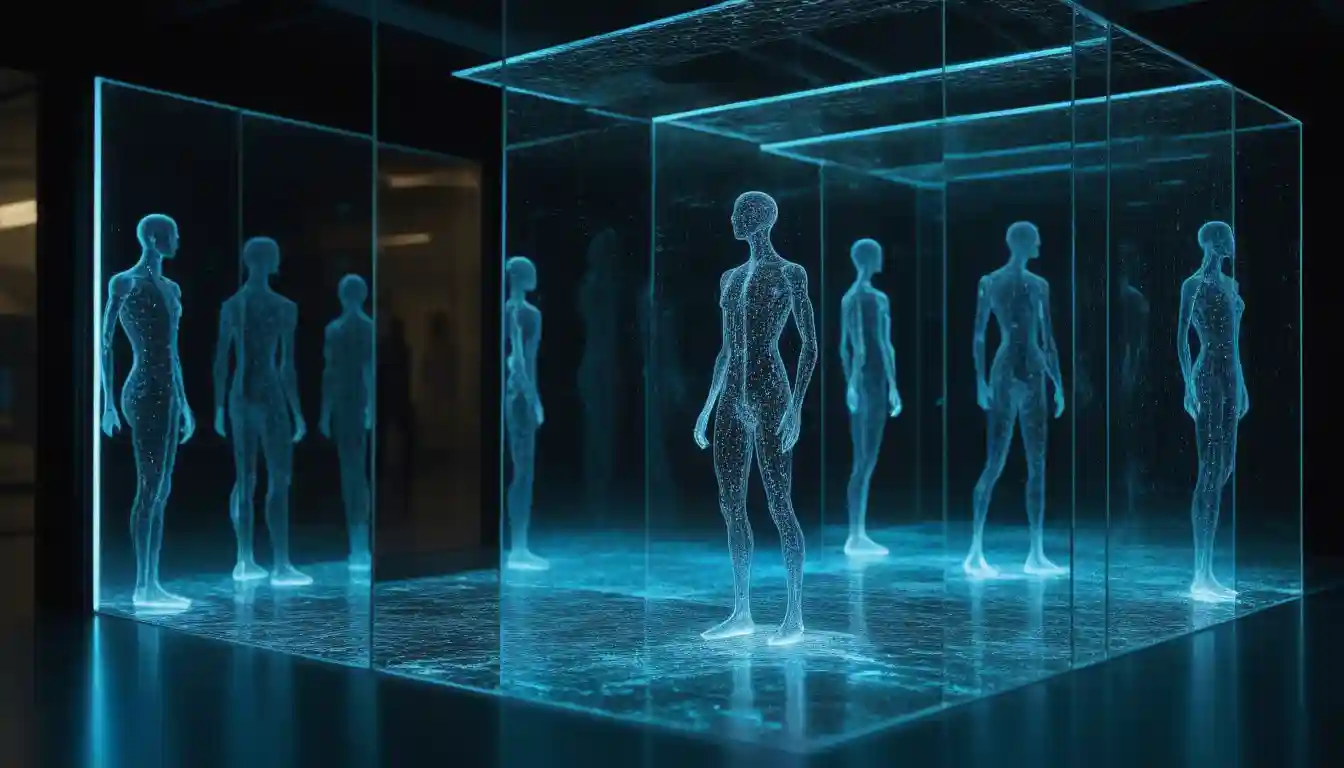
DeepMind Researcher Unveils Socratic Learning Framework for Self-Improving AI
Google DeepMind researcher Tom Schaul introduces a groundbreaking framework aimed at empowering AI systems to self-improve without further human intervention.
A new research paper by Google DeepMind's Tom Schaul proposes a revolutionary framework called "Socratic learning," which is intended to allow artificial intelligence (AI) systems to enhance their capabilities autonomously. This new approach addresses a critical challenge in AI: how to create systems that can continue learning and advancing even after their initial training phase. Schaul’s research, which is currently under peer review, focuses specifically on language-based systems, suggesting a potential shift in how we view AI's capacity for self-improvement.
The paper puts forward a theoretical model where AI could master any skill within a closed system, given three fundamental conditions: aligned feedback, broad experience coverage, and adequate computational resources. The concept is particularly significant for language-based AI, which could use its own outputs as new inputs, fostering continuous learning without external human inputs. This could pave the way for AI systems to become more sophisticated, potentially leading to artificial superhuman intelligence (ASI).
Key innovations in the proposed framework include the introduction of "language games" to drive AI's self-improvement and a focus on specialized tasks, rather than trying to achieve a universal learning approach. Schaul's framework also tackles fundamental issues in AI alignment—ensuring AI systems evolve in line with human values—and suggests a strategy that may help mitigate risks linked to AI autonomy.
The paper further elaborates on the three critical conditions necessary for effective Socratic learning:
- Aligned Feedback: Feedback must be carefully crafted to guide the AI towards desirable outcomes. This involves designing reward mechanisms that reflect human values and goals, ensuring that the AI's progression aligns with what is beneficial for humanity.
- Broad Coverage of Experiences: The AI system needs access to a wide range of experiences within the closed system to continually improve. The broader the scope of experiences, the more capable the AI becomes at generalizing its knowledge to new, unforeseen tasks.
- Sufficient Computational Resources: AI must have access to substantial computational power to iterate, learn, and refine its capabilities. This is essential for supporting complex internal simulations and generating new training data autonomously.
The proposed framework makes extensive use of language games—structured interactions that help the AI system question, answer, and refine its understanding of the world. These games provide a dynamic way for AI to self-assess and generate new learning challenges internally. This approach moves beyond simple reinforcement learning by encouraging the AI to think iteratively and explore different possible solutions for the same problem, akin to how a philosopher might explore multiple dimensions of a philosophical question.
Another significant insight from Schaul's paper is the concept of generative feedback loops, where the AI system can create its own training scenarios based on past experiences and current objectives. This type of self-generated feedback aims to minimize the need for human intervention, allowing the AI to adapt to new challenges independently. It also introduces an additional layer of safety, as the AI can identify gaps in its knowledge and actively seek to address them through these feedback loops.
The research comes at a time when DeepMind has made remarkable progress in AI capabilities, including recent successes in solving advanced mathematical problems at the level of the International Mathematical Olympiad. In particular, DeepMind has shown how sophisticated models can engage in tasks such as automated theorem proving and mathematical conjecture exploration. Though theoretical in nature, the framework provides a clear roadmap for building self-improving AI, hinting at what might be possible in future iterations of artificial intelligence.
Key Takeaways
- Socratic Learning: This new approach emphasizes using language as the primary means for recursive learning, which could revolutionize the development of AI that learns autonomously without further human input.
- Language Games for AI Development: "Language games" serve as a novel mechanism that allows AI systems to generate their own training scenarios and feedback mechanisms—leading to continuous improvement. These games are modeled after human interaction patterns and provide a rich structure for iterative knowledge building.
- Targeted Self-Improvement: The focus on specialized, narrow tasks rather than a universal system may offer a safer, more controlled path towards creating advanced AI systems that still align with human values. Specialized tasks help maintain a clear goal orientation, preventing the AI from developing unpredictable behaviors.
- Generative Feedback Loops: The ability of AI to create its own learning opportunities and refine its understanding without human intervention is a major step towards reducing dependency on manually labeled datasets.
- Risk Management: The paper highlights the risks involved, particularly in maintaining value alignment, and suggests that a narrow focus on defined tasks can help manage these potential threats. Robust oversight mechanisms are needed to ensure that the system evolves safely and remains aligned with human ethical standards.
Deep Analysis
The introduction of Socratic learning is a notable step forward in addressing one of the core ambitions of AI research: autonomous, ongoing learning. This framework builds upon advances in large language models and suggests an evolution toward self-sustaining AI development. In essence, Schaul’s framework envisions AI systems that can bootstrap their learning capabilities through iterative questioning and refinement, much like how human philosophers engage in Socratic dialogues.
One of the key innovations is the use of "language games" as a core mechanism for AI to refine its understanding. Instead of relying solely on pre-constructed datasets, AI could generate new learning opportunities by creating internal dialogues and scenarios. This has vast potential applications, from mathematical research to natural language understanding. For example, Schaul provides a thought-provoking example of how AI could theoretically work on mathematical problems like the Riemann hypothesis, using its self-generated knowledge to drive new insights.
This method diverges from the monolithic, one-size-fits-all approach to AI learning and instead favors multiple narrow, specialized tasks. By focusing on specific domains, such as mathematical research or language reasoning, Socratic learning aims to create more robust, specialized AI systems that can continually improve while mitigating the risks of unchecked evolution or misalignment. The safety aspect is crucial—instead of building an AI that seeks to understand "everything," a narrower scope ensures more predictable and controllable development paths.
However, this proposal also comes with challenges, particularly regarding ethical considerations. The risk of misalignment in a closed, self-referential learning loop is significant, and the research emphasizes the importance of oversight mechanisms. If AI systems evolve by referencing only their own outputs, there is the potential for unintended behaviors or emergent characteristics that diverge from human values. Ensuring that feedback mechanisms remain aligned is critical for safe advancement. The ethical oversight suggested includes monitoring the AI's feedback generation processes and implementing strict alignment checks to avoid any drift from intended goals.
Did You Know?
- Recursive Learning Could Change the Game: Recursive Socratic learning aims to keep AI systems improving indefinitely. Unlike current models that require updated human training data, this new approach would let AI systems drive their own learning process.
- AI in Mathematics: The paper suggests that AI could autonomously explore complex mathematical problems like the Riemann hypothesis, potentially pushing the frontiers of human knowledge in pure mathematics. This aligns with DeepMind's recent achievements in automating theorem proving and competing at Olympiad-level problem-solving.
- Language Games as AI Teachers: Language games aren't new—they've been used in linguistics for decades. Applying this to AI learning could open up entirely new avenues for autonomous learning, allowing AI systems to learn by creating internal "teaching" situations. The concept is reminiscent of classic educational psychology, where engagement and dialogue play crucial roles in the learning process.
- Ethical Oversight is Key: The concept of self-improving AI may sound exciting, but it raises critical ethical questions. The paper suggests maintaining strict alignment protocols to ensure that AI developments remain beneficial to humans. Strong ethical oversight and regular audits of the AI's learning progress are necessary to prevent undesirable emergent behaviors.
- Multi-Agent Socratic Learning: The framework hints at the possibility of using multiple AI agents in collaborative "language games" to achieve collective problem-solving, thereby improving the overall robustness of the learning process and diversifying the learning experiences.
Conclusion
Tom Schaul's framework for Socratic learning could potentially redefine how we view AI’s capabilities, pushing toward an era where AI systems are not just passive tools but active participants in their own evolution. By leveraging language as a vehicle for recursive learning, this research hints at the development of AI systems that could make continuous, autonomous strides in areas ranging from scientific research to conversational interactions. However, the journey toward autonomous AI will need careful monitoring, with human values remaining central to prevent unintended outcomes.
The challenge now lies in translating these theoretical advancements into practical applications while ensuring robust ethical governance. As DeepMind pushes the boundaries of AI research, Schaul's Socratic learning framework presents an exciting, albeit complex, path forward. The real-world implementation of these ideas will need to address concerns of feedback alignment, ethical oversight, and computational scalability to ensure that the benefits of self-improving AI are realized safely and effectively.