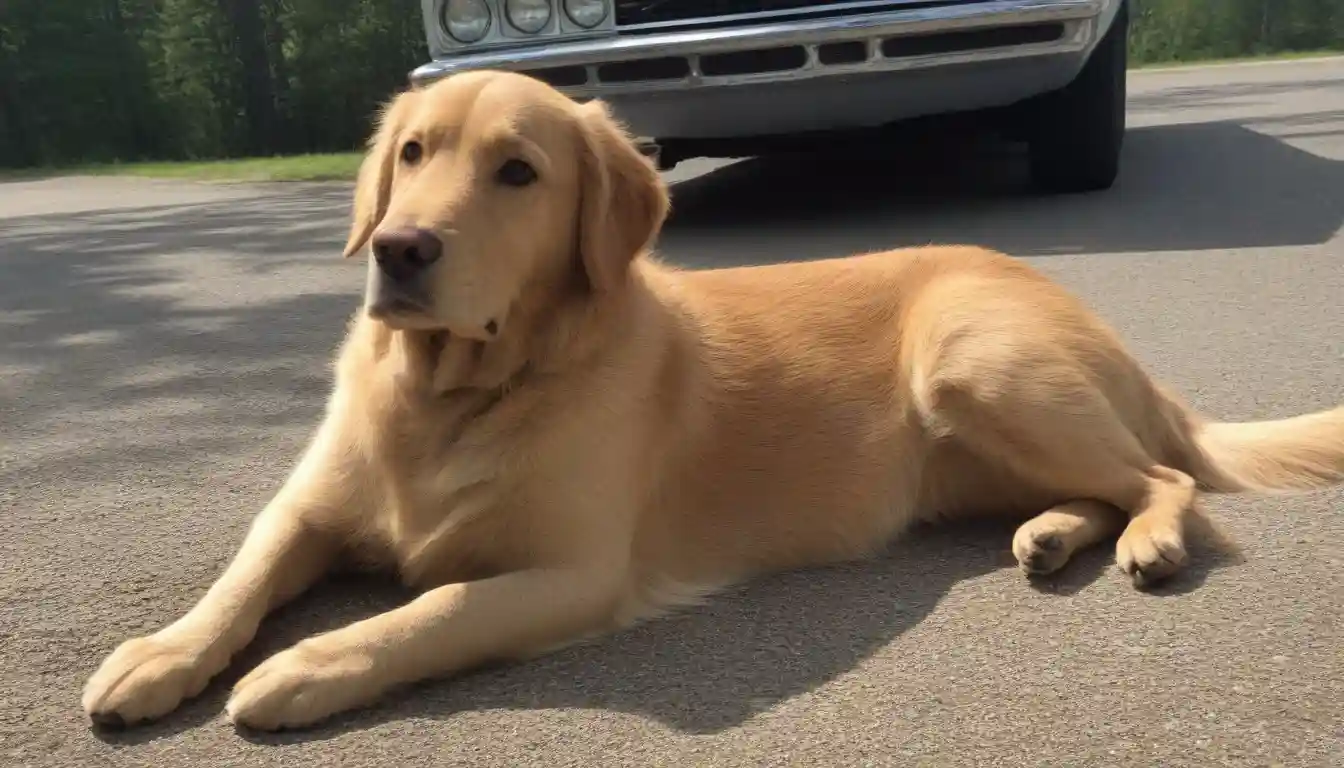
DrEureka: Automating Sim-to-Real Transfer of Robot Skills with GPT-4
A groundbreaking method named DrEureka, developed collaboratively by the University of Pennsylvania, Nvidia, and UT Austin, is revolutionizing the robotics and AI industries. DrEureka harnesses the power of Nvidia's GPT-4, a large language model, to automate the transfer of robot skills from simulation to the real world, marking a significant step towards the realization of humanoid robots in industrial settings.
DrEureka's innovative approach involves automating the creation of reward functions and configurations for domain randomization, thereby enhancing the sim-to-real transfer process. Impressively, this method surpasses the capabilities of human experts in legged and manipulator robot platforms, showcasing its potential in automating complex design aspects of sim-to-real learning.
Key Takeaways
- DrEureka streamlines sim-to-real transfer of robot skills using large language models
- This method improves domain randomization parameter selection utilizing AI language models
- DrEureka leverages OpenAI's GPT-4 to produce reward functions and domain randomization parameters
- Outperforming human experts in legged and manipulator robot tasks, DrEureka demonstrates the potential for accelerating sim-to-real transfer in robot learning research
Analysis
The emergence of DrEureka holds immense implications for the robotics and AI sectors, offering potential benefits to industry players such as Boston Dynamics and Tesla. By reducing costs and time associated with translating simulated skills into real-world applications, this technology stands to revolutionize the field. Moreover, manufacturing-driven economies like China and Germany could witness heightened efficiency and productivity in their factory operations.
The integration of language models with reinforcement learning techniques is the driving force behind this advancement, facilitating the creation of reward functions and domain randomization parameters. DrEureka's superior performance in diverse robot tasks underscores the capacity of AI language models, like GPT-4, to address intricate design facets of sim-to-real learning.
In the near term, researchers and businesses can anticipate accelerated progress in robot learning research, with prospective applications spanning manufacturing, logistics, and service industries. In the longer term, the success of DrEureka could stimulate increased investments in AI and robotics, fostering progress in humanoid robots and automation technologies. However, it also raises pertinent concerns about job displacement and ethical AI usage in the workforce.
Did You Know?
- Sim-to-real transfer of robot skills: This process involves transferring a robot's learned skills from a simulated environment to the real world, posing a challenging problem in robotics due to the unpredictable nature of the real world.
- Domain randomization: This technique enhances the sim-to-real process by randomizing parameters within the simulated environment, enabling the robot to adapt to a wider array of real-world conditions.
- Large language models (LLMs): These AI models generate human-like text based on patterns learned from extensive datasets. In the context of DrEureka, LLMs automate the creation of reward functions and configurations for domain randomization, enhancing the efficiency of the sim-to-real process.