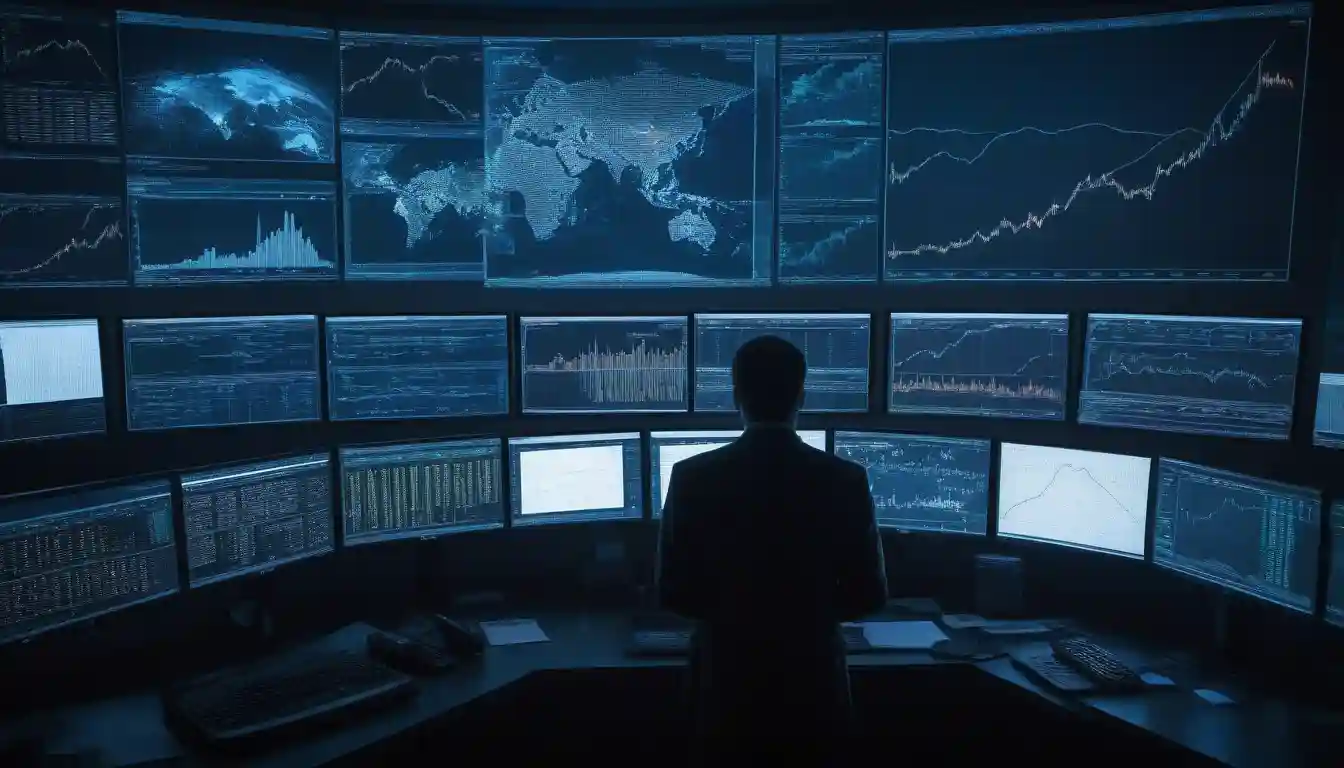
Large Language Models (LLMs) Transform Financial Analysis Landscape
A recent study conducted by the University of Chicago reveals the exceptional capability of large language models (LLMs), exemplified by GPT-4, in analyzing financial statements. The study highlights that these LLMs can surpass human analysts in accuracy while generating valuable insights and trading strategies with higher Sharpe ratios.
Key Takeaways
- GPT-4's analysis of financial statements rivals or exceeds human analysts' accuracy.
- LLMs like GPT-4 outperform human analysts in predicting changes in earnings.
- LLMs produce valuable insights and yield higher returns with trading strategies.
- The advantage of LLMs lies in their extensive knowledge base and rapid pattern recognition.
- LLMs are poised to streamline work and enhance decision-making for human analysts in the future.
Analysis
The implications of the University of Chicago's study on GPT-4's financial analysis prowess are profound for the finance sector. This development signals the potential integration of large language models (LLMs) into the analysis processes of esteemed organizations such as Moody's, S&P Global, and BlackRock, thereby enhancing efficiency and accuracy. Consequently, human analysts may need to adapt, focusing on higher-level strategic decisions and regulatory compliance.
The rapid advancement of LLMs, which excel in pattern recognition and possess vast knowledge bases, is the driving force behind this transformative development. This innovation is likely an indirect result of increased data availability and computational power. In the short term, we anticipate financial institutions exploring LLM integration, potentially prompting workflow adjustments. In the long run, this could engender a shift in the skill set required for financial analysts and enhance market transparency due to more precise earnings predictions, ultimately fostering more informed investment decisions and a more stable financial market.
Did You Know?
- Large Language Models (LLMs): These AI models are trained on extensive text data, enabling them to understand and generate human-like text. They possess the capacity to analyze, summarize, answer questions, and creatively generate content. The study utilized GPT-4, one of the most advanced LLMs developed by OpenAI.
- Sharpe ratio: This risk-adjusted measure is employed in finance to assess the performance of investments or trading strategies. A higher Sharpe ratio signifies better risk-adjusted performance. For instance, the study found that the trading strategies generated by GPT-4 exhibited higher Sharpe ratios than other models, indicating the potential for higher returns with lower risk.
- Trading strategies: These comprise rules or guidelines followed by investors and traders to make buy and sell decisions in financial markets. The researchers found that GPT-4 could generate insightful trading strategies based on corporate financial statements, potentially aiding in predicting future earnings growth and facilitating informed investment decisions, thereby possibly leading to higher returns for investors.