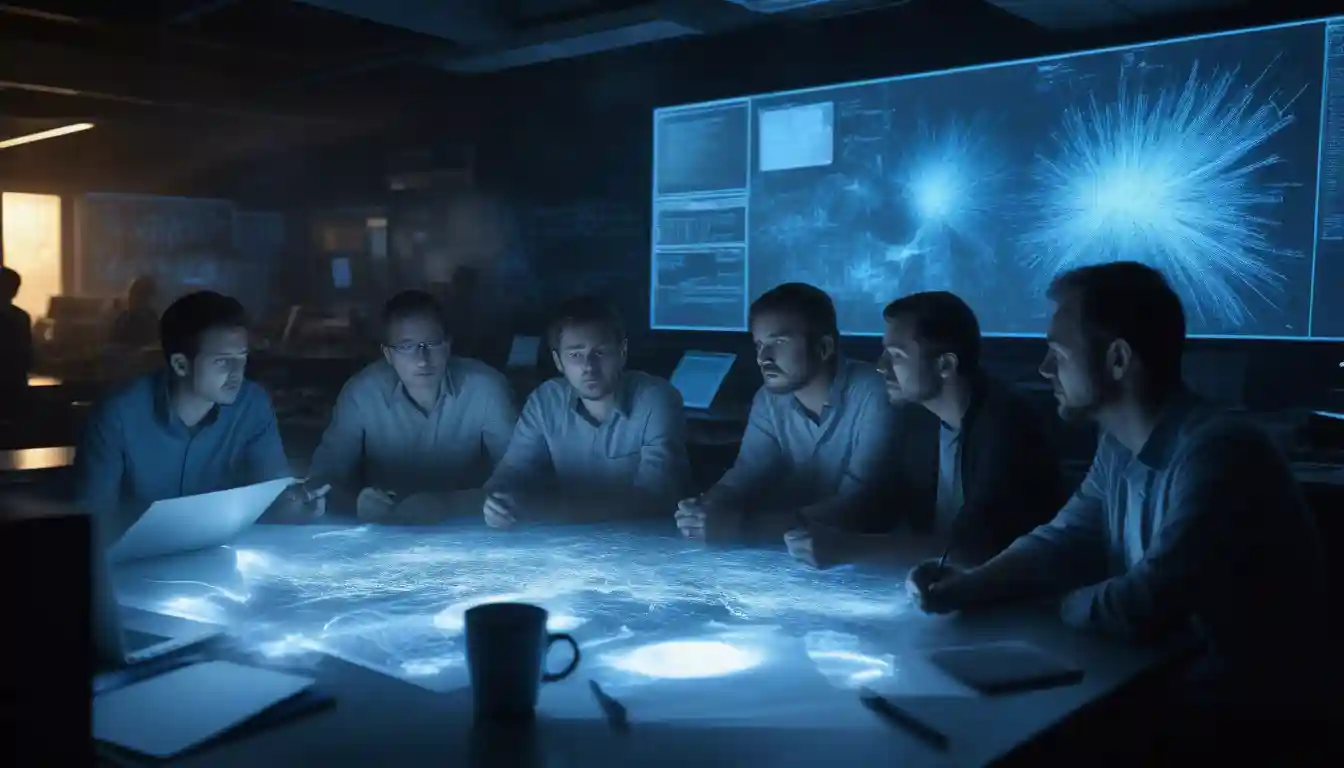
Insider’s Deep Dive: How GPT o1’s Reinforcement Learning is Revolutionizing Causal Reasoning in AI
OpenAI has introduced its latest model, GPT o1, which stands as a new landmark in artificial intelligence. By integrating reinforcement learning (RL), the model surpasses prior limitations, offering enhanced reasoning capabilities, specifically through causal reasoning. This technological leap marks a critical shift in how AI models learn, adapt, and solve complex problems.
What Happened?
The rapid evolution of AI has reached a critical juncture with OpenAI’s release of GPT o1, which embraces reinforcement learning as a fundamental tool to enhance its capabilities in chain-of-thought reasoning. Traditional methods of pretraining AI models on large datasets are showing diminishing returns. With models like GPT-3.5 and GPT-4, scaling parameters and data yielded only marginal performance improvements.
OpenAI pivoted towards post-training, utilizing reinforcement learning to help GPT o1 not just predict probable text sequences, but to understand why certain outputs are correct. This new training method refines GPT o1’s ability to grasp deeper cause-effect relationships, which boosts its proficiency in tasks that demand logical reasoning. The results? A model that not only processes information but also reasons through complex problems, making it significantly less prone to errors, such as hallucinations.
Key Takeaways
- Pretraining's Limits: OpenAI's earlier models, such as GPT-4, faced diminishing returns from scaling up parameters. With GPT o1, reinforcement learning (RL) post-training has become the new frontier.
- Causal Reasoning: GPT o1 stands apart from predecessors by mastering causal reasoning, moving beyond simple correlations. This makes the model more adept at solving complex, real-world problems.
- Reducing Hallucinations: The chain-of-thought reasoning introduced in GPT o1 significantly reduces hallucinations, where models typically generate false information.
- Coding as a Prime Application: GPT o1 is expected to show the strongest real-world impact in coding tasks, thanks to RL's trial-and-error refinement, ideal for structured environments like programming.
- Self-Play Reinforcement Learning: A unique RL method, self-play, has been incorporated into GPT o1 to allow the model to refine its skills iteratively, particularly in reasoning and problem-solving.
Deep Analysis
Pretraining’s Diminishing Returns and RL’s Rising Role
Pretraining has been the cornerstone of AI model development, but its effectiveness is waning. As OpenAI scaled its models from GPT-3.5 to GPT-4, the improvement in performance was not proportional to the investment. This plateau prompted a shift towards reinforcement learning, a training technique that involves the model interacting with an environment, receiving feedback, and adjusting its approach based on this feedback.
GPT o1 represents the beginning of this post-training era. By leveraging RL, the model evolves through interaction rather than just static learning. The transition from supervised fine-tuning (SFT), which teaches models to mimic patterns from human-labeled data, to RL allows GPT o1 to learn not only what patterns exist but why they are the correct solution. This new approach enhances the model’s understanding of causality—critical for handling complex, real-world challenges.
Causal Reasoning: A Quantum Leap for AI
One of the groundbreaking features of GPT o1 is its ability to engage in causal reasoning. Traditional models have excelled at identifying correlations, but often failed to recognize cause-and-effect relationships. With RL, GPT o1 experiments with different sequences and receives feedback on its choices. This process enables the model to make decisions based on the logical flow of information, greatly improving its reasoning capabilities. This is especially evident in tasks that require deep thinking and problem-solving, such as coding or scientific inquiry.
Minimizing Hallucinations
A common issue with large language models is the generation of incorrect or illogical statements—termed hallucinations. These errors arise from the model’s failure to fully understand the data’s logical structure. GPT o1 tackles this issue head-on by introducing chain-of-thought reasoning, which enhances its ability to follow logical steps from premises to conclusions. Furthermore, the RL-based feedback mechanism allows GPT o1 to continuously refine its responses, learning from its mistakes and reducing the likelihood of producing false information.
Challenges and Solutions in RL for GPT o1
While GPT o1's advancements are impressive, it faces three significant challenges: rewards modeling, prompting, and search optimization.
- Rewards Model: GPT o1 requires a sophisticated rewards model that can properly guide its behavior without reinforcing undesirable actions like bias or hallucinations. This model ensures that feedback is aligned with human values, a complex but essential part of the RL process.
- Prompting: Effective prompting is another challenge. To fully leverage its reasoning capabilities, GPT o1 must be presented with prompts that are just beyond its current capabilities—pushing the model to grow and learn without overwhelming it.
- Search Optimization: In tasks where multiple possible outputs exist, GPT o1 must efficiently explore various solutions. Reinforcement learning facilitates this by allowing the model to test multiple approaches, but optimizing this exploration remains a significant hurdle.
The combination of these elements—rewards, prompting, and search—enables GPT o1 to break new ground in reasoning and adaptability, though these advancements are challenging to replicate outside of OpenAI's highly specialized environment.
Did You Know?
-
Self-Play: GPT o1 employs a version of self-play, a method that has achieved remarkable success in game-playing AIs, such as those mastering Go. In this method, the model improves by iteratively challenging itself. However, in GPT o1’s case, OpenAI had to adapt self-play to work with language tasks, a feat that has not been simple to accomplish.
-
Limited Data Availability: As AI models like GPT o1 advance, the amount of high-quality training data available diminishes. Reinforcement learning helps mitigate this challenge by allowing the model to generate and refine its own data through self-play and feedback. However, this data scarcity remains a long-term concern for the AI field.
-
Proximal Policy Optimization (PPO): One of the reinforcement learning techniques used in GPT o1 is PPO (Proximal Policy Optimization), which allows the model to explore various possibilities and improve through trial and error. PPO makes GPT o1 more computationally intensive but exponentially boosts its reasoning capabilities compared to older methods like DQN.
Conclusion
GPT o1 is pushing the boundaries of AI with its focus on chain-of-thought reasoning, causal understanding, and a robust reinforcement learning framework. As the world moves towards more intelligent, reasoning-capable models, GPT o1 stands as a prime example of the next generation of AI systems. Its success in handling complex tasks like coding and causal reasoning is just the beginning, with the promise of further innovations on the horizon.