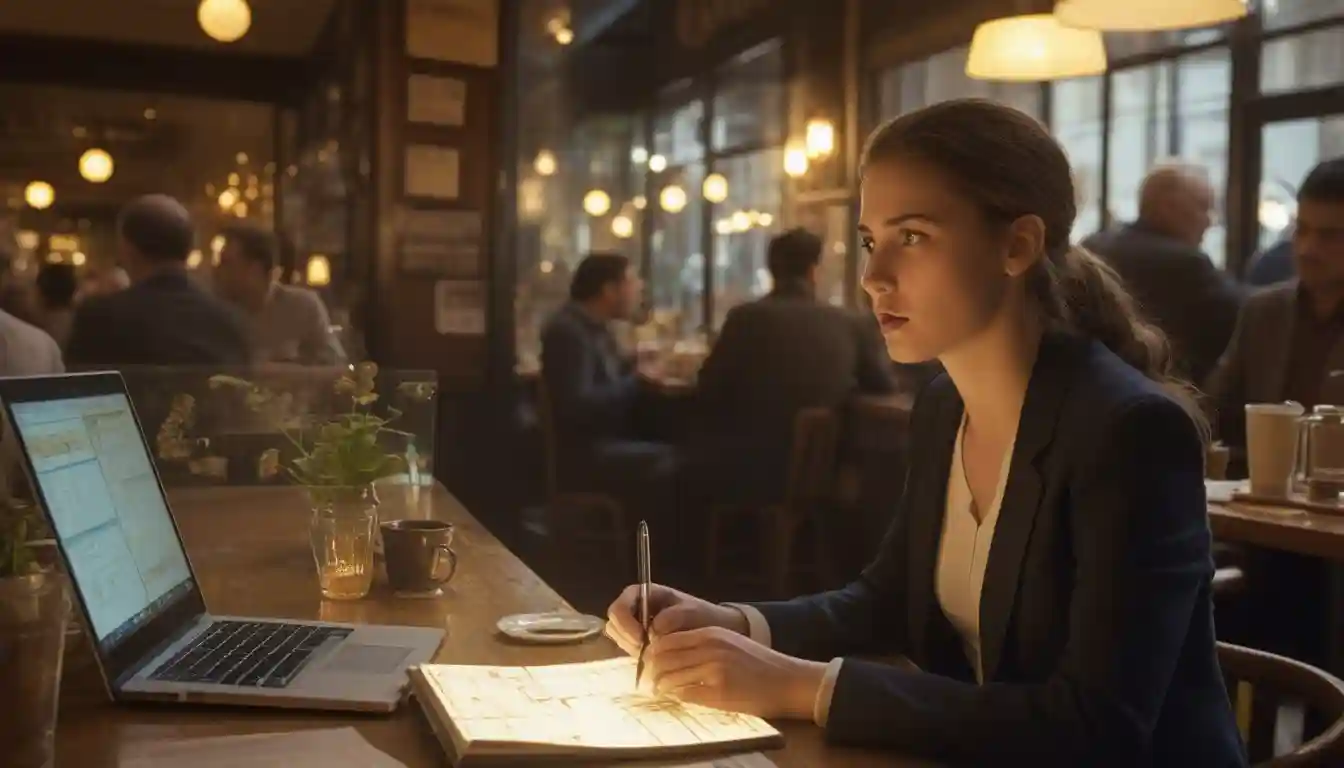
High-Flyer’s DeepSeek-V3 Excels in AI Innovation, But Active Traders Still Outperform Its Trading AI in Markets
High-Flyer’s DeepSeek-V3 Sets New Standards in AI, But Active Managers Still Outperform AI-Driven Stock Trading
January 1, 2025 – On December 30th, 2024, DeepSeek, an AI startup established by the renowned quantitative asset management leader High-Flyer, unveiled its groundbreaking large language model, DeepSeek-V3. This cutting-edge model surpasses mainstream open-source alternatives in various benchmark tests and performs comparably to top-tier closed-source models, with exceptional capabilities in coding and mathematics. However, while the model represents a major leap forward in AI technology, the performance of AI-driven stock trading strategies, including High-Flyer’s own efforts, highlights ongoing challenges in achieving consistent market outperformance.
DeepSeek-V3: A Game-Changer in AI Technology
DeepSeek-V3 showcases High-Flyer’s technical prowess, outshining many open-source models and rivaling leading proprietary systems in complex domains such as mathematics and coding. This development cements High-Flyer’s position as a major player in the AI sector, leveraging its extensive expertise in quantitative finance to push the boundaries of artificial intelligence.
High-Flyer’s AI for Stock Trading: Mixed Results for Investors
High-Flyer’s foray into AI-driven trading strategies has attracted significant attention from investors intrigued by the potential of "AI stock trading." However, results reveal a more nuanced reality. According to the Private Fund Rankings Network, out of 65 funds managed by High-Flyer that publicly disclose performance data, only 29 achieved annual returns exceeding 10%, while the remaining 36 experienced declines. Furthermore, quantitative long positions underperformed discretionary stock strategies, raising questions about the effectiveness of AI in delivering superior investment returns.
Active vs. AI-Driven Management: A Global Perspective
The struggles of AI-driven strategies to outperform active management are not unique to High-Flyer or China. In the United States, AI-powered funds like those managed by Qraft Technologies, including the Large Cap Momentum ETF (AMOM) and the diversified multi-factor ETF (QRFT), have similarly underperformed their benchmarks. These global trends underline the challenges AI faces in navigating unpredictable and dynamic market conditions.
AI in Asset Management: Enhancer, Not a Replacement
Despite these challenges, AI remains a vital tool in asset management, enhancing tasks such as data analysis, predictive modeling, and risk management. Experts emphasize that AI is best used as a complement to human fund managers, not as a substitute. The combination of AI’s computational capabilities with human judgment and strategic decision-making is widely seen as the optimal approach for achieving better investment outcomes.
Performance Analysis: Why Active Management Prevails
The Strength of Active Management
Active fund managers succeed by leveraging:
- Human Intuition: The ability to incorporate non-quantifiable factors like geopolitical events, regulatory changes, and market sentiment.
- Dynamic Adaptability: Quick pivots in strategy to respond to unforeseen market changes.
- Strategic Allocation: Expertise in identifying and weighting emerging sectors or undervalued themes.
Challenges for AI Strategies
- Overfitting and Bias: AI models may overfit historical data, creating strategies that excel in simulations but fail in live markets.
- Complexity of Market Dynamics: Human behavior, black swan events, and shifting market structures are inherently difficult to model mathematically.
- Competition Among AI Models: The proliferation of similar strategies dilutes the alpha-generating potential.
- Market Liquidity Constraints: AI often operates in highly liquid markets, where alpha opportunities are scarce.
Looking Ahead: The Future of AI in Finance
AI’s Advantages in the Long Run
- Data Processing at Scale: AI can analyze vast datasets, identifying correlations that might elude human analysis.
- Operational Efficiency: Automating routine tasks reduces costs and increases speed.
Emerging Roles for AI
- As a decision-support tool, AI can aid in scenario analysis, portfolio optimization, and risk assessment, empowering human managers to make more informed choices.
Industry Shifts and Strategic Adaptations
AI’s widespread adoption is transforming asset management:
- Market Hyper-Efficiency: AI’s growing presence in liquid markets is driving efficiency, leaving less room for exploitable inefficiencies.
- Hybrid Models: The future lies in blending human creativity with AI insights, creating strategies that leverage the strengths of both.
Recommendations for Stakeholders
For Asset Managers:
- Invest in developing hybrid approaches that integrate AI’s analytical capabilities with human decision-making.
- Explore less efficient markets where AI has room to generate unique insights.
For Investors:
- Diversify portfolios to include both active and AI-driven strategies.
- Favor funds that provide clear communication about their AI integration and oversight processes.
For AI Developers:
- Focus on adaptability and real-time learning to improve AI’s performance in dynamic markets.
- Collaborate with finance professionals to enhance the relevance and interpretability of AI models.
Conclusion
High-Flyer’s DeepSeek-V3 underscores the immense potential of AI to transform industries, including finance. However, the challenges faced by AI-driven quantitative strategies reveal the enduring importance of human expertise in navigating complex and unpredictable markets. As technology advances and hybrid strategies emerge, the collaboration between AI and human fund managers will likely shape the future of asset management, blending computational power with strategic insight for a more sophisticated and efficient financial ecosystem.