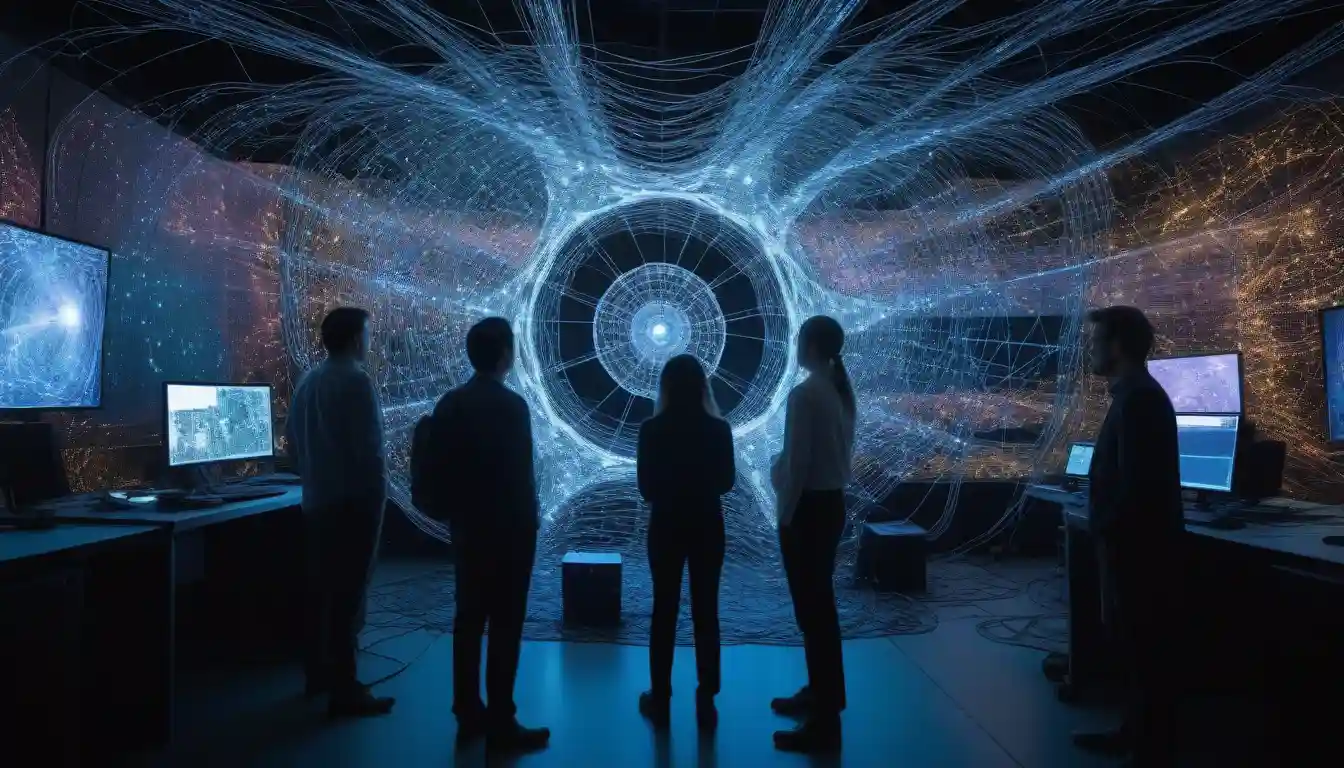
Stanford Report Raises Concerns Over Depletion of High-Quality Language Data for AI Models
A recent report from Stanford’s Human-Centered Artificial Intelligence Institute forecasts the depletion of high-quality language data for training AI models in 2023, potentially leading to a "quality wall" for large language models. This could pose significant challenges for AI companies like OpenAI and Anthropic. Concerns are amplified by limited data transparency, raising questions about the sustainability and quality of AI model development. However, there are indications that the situation may not be as dire as predicted.
Key Takeaways
- The AI Index Report from Stanford’s Human-Centered Artificial Intelligence Institute predicts a depletion of high-quality language data for training AI models in 2023.
- Large language models rely on data quantity and computing power to improve, but the forecast suggests a potential quality wall due to data supply depletion.
- Limited data transparency regarding the training data used by companies like OpenAI and Anthropic raises concerns about the future sustainability and quality of AI models.
- There are indications that the forecast's implications might not be as severe as initially anticipated.
Analysis
The potential exhaustion of high-quality language data for AI models in 2023, as projected by the Stanford report, has far-reaching implications for AI companies, particularly in the realm of large language models. Transparency issues regarding training data further compound these concerns, prompting discussions on data governance and responsible AI practices.
Did You Know?
- High-quality language data: In the context of AI, this refers to the extensive text data employed to train large language models. The data's quality significantly influences the accuracy and relevance of the AI's generated text.
- Scaling laws: In the realm of AI, this concept denotes the relationship between a machine learning model's performance and the computational resources used in its training. The forecast's potential quality wall for LLMs underscores the impact of data depletion on model performance.
- Data transparency: This term alludes to the extent to which companies and researchers divulge information about the data utilized in training AI models. Limited transparency raises concerns about the long-term sustainability and quality of AI model development.