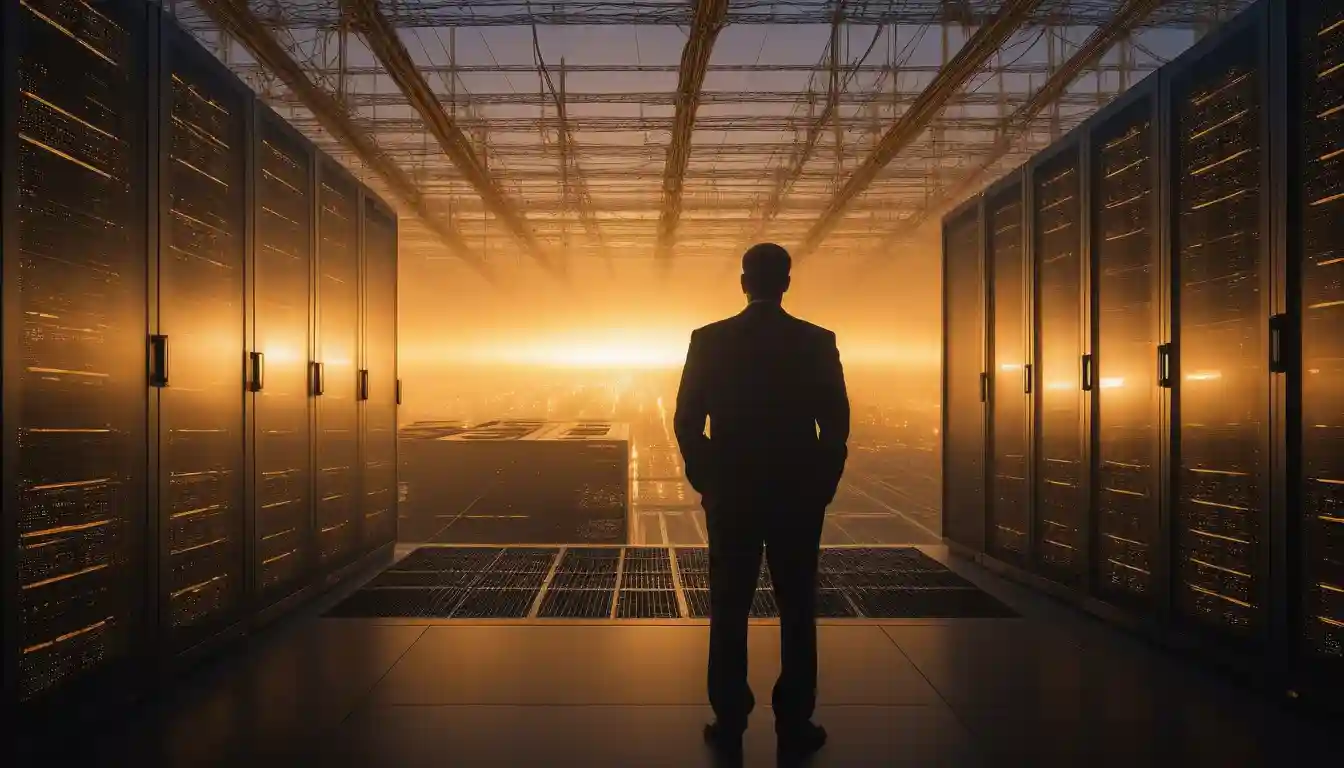
The Vital Role of Data Quality in Machine Learning and AI Projects in Enterprises
Have you ever pondered on the intricate tasks performed by machine learning engineers? More than just building models and analyzing data, their responsibilities extend into ensuring impeccable data quality. In today's business landscape, data holds paramount significance, yet its effectiveness hinges on its cleanliness and usability.
The harrowing truth is that numerous businesses grapple with data quality issues, which can significantly impede their machine learning endeavors. Shockingly, data scientists find themselves dedicating up to 80% of their time on data cleansing, consequently leaving them with limited resources to tackle actual business challenges. Tragically, this predicament often engenders projects riddled with inefficiency and diminished outcomes.
A real-world example of the critical importance of data quality can be seen in the healthcare sector. IBM's Watson for Oncology partnered with The University of Texas MD Anderson Cancer Center to analyze patient data and provide treatment recommendations. However, the system was trained on a limited set of hypothetical patient data rather than real-world data. This led to incorrect and potentially dangerous treatment advice, highlighting the risks of using flawed or incomplete data in machine learning applications.
Data quality's impact extends beyond model accuracy; it also affects the generalizability and real-world performance of machine learning models. Poor data quality, such as missing values or inconsistencies, can lead to biased or inaccurate models, ultimately resulting in unreliable predictions and decisions. This is especially critical in high-stakes industries like healthcare, where flawed data can have severe consequences.
Another notable example is the UK exam grading algorithm used during the COVID-19 pandemic. Due to the pandemic, exams were canceled, and an algorithm was used to predict students' grades based on historical data and teacher assessments. However, the algorithm's reliance on flawed data led to widespread downgrading of students' grades, disproportionately affecting those from disadvantaged backgrounds. This incident caused significant public outcry and demonstrated the potentially harmful effects of poor data quality in automated decision-making systems.
As the importance of AI continues to soar in the corporate sphere, the backbone of AI undeniably remains data quality. Businesses plagued by subpar data quality are bound to witness the downfall of their AI initiatives. The key lies in implementing best practices such as developing robust data collection strategies, ensuring comprehensive data validation and cleansing, and leveraging automated data quality checks. For instance, using machine learning algorithms for anomaly detection can proactively identify and address data issues, maintaining high data integrity throughout the machine learning lifecycle.
In essence, the crux of the matter stretches beyond mere model construction and data analytics; it revolves around ensuring the cleanliness and usability of the data utilized, enabling businesses to genuinely conquer their operational dilemmas. Organizations can overcome data quality challenges by prioritizing continuous testing and appointing dedicated data custodians. By elevating the status of data quality, companies can optimize their machine learning models and yield superior business outcomes, driving innovation and efficiency in their respective fields.
Key Takeaways
- The reliability of machine learning models is significantly influenced by data quality.
- Data scientists invest a staggering 60-80% of their time in data cleansing activities.
- Ineffectual data quality results in suboptimal project outcomes and hampers progress.
- The growth of AI hinges on data quality, with 33% of AI projects succumbing to data inadequacies.
- Persistent data scrutiny and unequivocal ownership are pivotal for surmounting data quality impediments.
Analysis
Data quality challenges emerge as formidable bottlenecks for AI and machine learning projects, exerting profound repercussions on their efficacy and progress. Esteemed entities like Google and IBM, heavily immersed in the realm of AI, confront potential setbacks should data quality lag behind. Financial instruments sensitive to technological advancements might encounter volatility as a consequence. In the short term, inadequate data handling imperils project timelines and budgets. Long-term sustenance involves vital enhancements in data quality, emerging as an imperative for scaling and fortifying AI reliability. The crux lies in unwavering testing procedures and comprehensive data governance to overcome these challenges and ensure the stalwart development of AI.
Did You Know?
- Data Quality in Machine Learning:
- Encompassing accuracy, completeness, consistency, and reliability, data quality is crucial for machine learning models. High-quality data nurtures accuracy, leading to dependable predictions and outcomes. Conversely, poor data quality spawns biases and errors, profoundly affecting the model's performance and the ensuing business decisions.
- Data Cleansing:
- The process of identifying and rectifying corrupt or inaccurate data within a dataset is encapsulated in data cleansing. This crucial procedure involves the identification and elimination of incomplete, incorrect, or irrelevant data segments, ultimately shaping the efficacy of the models built upon said data.
- Data Ownership:
- Within the context of data quality, data ownership pertains to the accountability held by specific individuals or teams to ensure the precision and upkeep of data. Clear data ownership facilitates seamless data management, continuous monitoring, and timely resolution of data quality predicaments, vital for sustaining high data quality amidst evolving business landscapes.