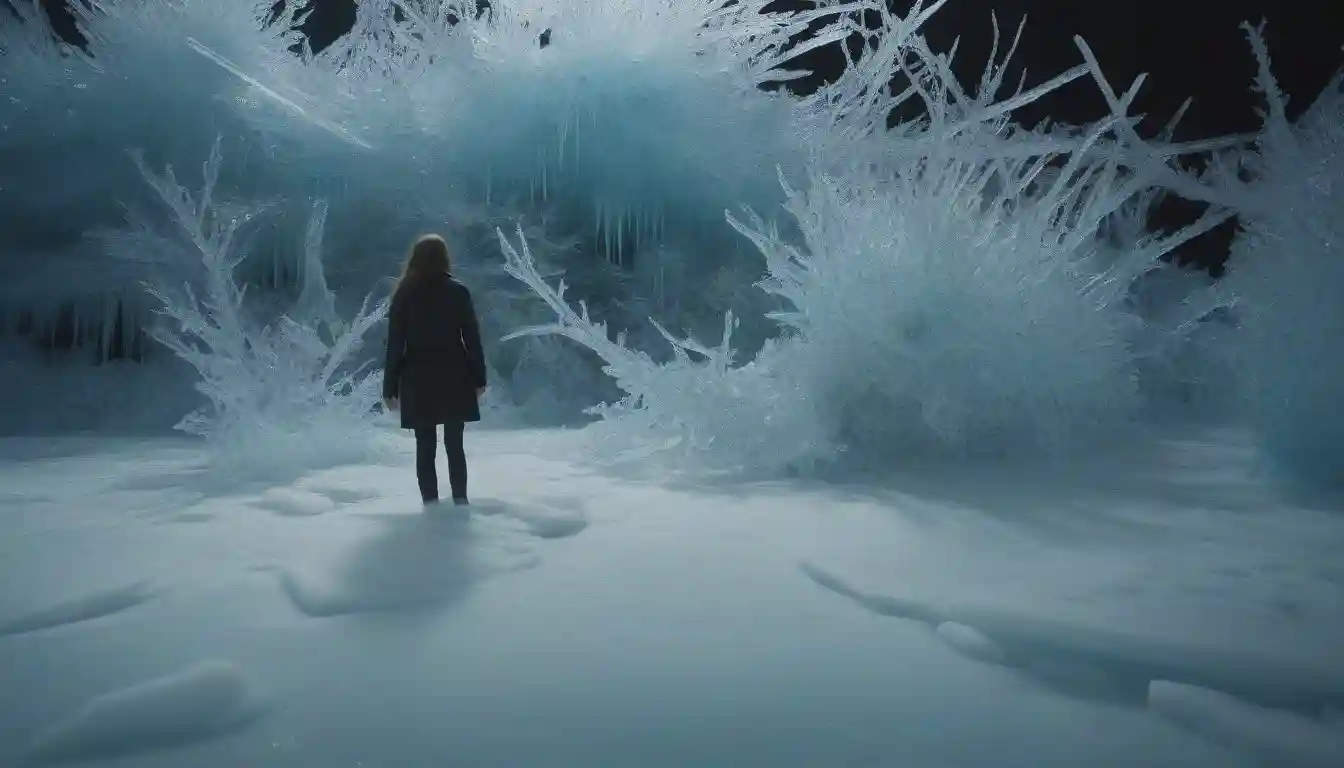
MIT CSAIL and Technical University of Munich Develop Groundbreaking "Diffusion Forcing" Technique for Video Generation and Robotics
Researchers from MIT CSAIL and the Technical University of Munich have introduced a pioneering approach known as "Diffusion Forcing," which seamlessly integrates autoregressive and diffusion models to achieve unparalleled advancements in video generation and robotic planning.
Key Takeaways
- Enhanced Performance: Diffusion Forcing amalgamates autoregressive and diffusion models to elevate performance across various applications.
- Unique Noise Levels: This technique empowers individual tokens within a sequence to possess distinct noise levels, thereby enhancing adaptability and robustness.
- Stability in Video Generation: Diffusion Forcing surpasses conventional methodologies in providing steadfast stability for video generation.
- Flexibility in Robotics: The methodology grants heightened flexibility and efficiency in planning and executing tasks within the realm of robotics.
- Scalability: The research team aims to further expand the scope of Diffusion Forcing to encompass larger datasets and higher resolutions.
Analysis
The advent of Diffusion Forcing by MIT CSAIL and TUM stands poised to revolutionize the landscape of both video generation and robotics. This innovative fusion of autoregressive and diffusion models not only bolsters stability and adaptability but also holds crucial implications for industries such as entertainment and manufacturing. The short-term repercussions are poised to manifest in enhanced video quality and optimized robotic performance, while the long-term implications could potentially extend to AI-driven sectors on a global scale. Foreseeably, advancements in AI technology, including those facilitated by Diffusion Forcing, hold the potential to elicit a positive response from financial markets, potentially catalyzing investments in related tech stocks. However, the scaling up of this groundbreaking innovation may encounter challenges pertaining to data handling and computational costs. Nonetheless, the potential for transformative breakthroughs in AI applications remains notably high.
Did You Know?
- Diffusion Forcing: This pioneering method, conceived by researchers at MIT CSAIL and the Technical University of Munich, harnesses the strengths of autoregressive and diffusion models to catalyze advancements across diverse applications, particularly in stabilizing video generation and fortifying planning in robotics tasks.
- Autoregressive Models: A vital component of statistical modeling within machine learning and time series analysis, autoregressive models are instrumental in forecasting future values based on a sequence of preceding values, thus proving critical for tasks necessitating the comprehension and prediction of sequential data.
- Diffusion Models: Belonging to the realm of generative models, diffusion models operate by gradually infusing noise into data, subsequently learning to reverse this process to generate new data samples. This methodology exhibits exceptional utility in facilitating controlled and steady generation of sequences, a capability emerging as pivotal within the context of Diffusion Forcing.
Additional Insights from the Research Paper
The research paper "Diffusion Forcing: Next-token Prediction Meets Full-Sequence Diffusion" by Boyuan Chen, Diego Martí Monsó, Yilun Du, Max Simchowitz, Russ Tedrake, and Vincent Sitzmann provides a detailed overview of the Diffusion Forcing technique. The paper highlights the following key points:
- Combining Strengths: Diffusion Forcing combines the strengths of next-token prediction models and full-sequence diffusion models. This hybrid approach allows for variable-length generation and guidance towards desirable trajectories.
- Novel Capabilities: The method introduces new sampling and guiding schemes that leverage the unique properties of Diffusion Forcing. This includes rolling out sequences of continuous tokens (like video) beyond the training horizon, where other models typically fail.
- Monte Carlo Tree Guidance (MCTG): This new capability significantly enhances the sampling of high-reward generations in decision-making and planning tasks. It utilizes a novel "zig-zag" sampling scheme that keeps the immediate future more certain than the far future, optimizing the planning process.
- Empirical Success: The empirical evaluations demonstrate that Diffusion Forcing achieves superior performance in various domains, including video generation, model-based planning, visual imitation learning, and time series prediction.
Technical Details
The Diffusion Forcing approach involves training a diffusion model to denoise a set of tokens with independent per-token noise levels. By applying this to sequence generative modeling, the researchers trained a causal next-token prediction model to generate future tokens without fully diffusing past ones. The model utilizes a recurrent neural network (RNN) for maintaining latent states, which capture the influence of past tokens and evolve through the sequence.
- Training Process: During training, the model is exposed to sequences with different noise levels for each token, forcing it to learn to "unmask" variably noised tokens. The training objective optimizes a variational lower bound on the likelihoods of all subsequences of tokens drawn from the true joint distribution.
- Sampling Process: The sampling process involves initializing tokens with white noise and denoising them according to a prescribed noise schedule. This process allows for stable long-horizon generation and effective sequence guidance.
Future Directions
The researchers plan to scale up Diffusion Forcing to larger datasets and more complex tasks. They also aim to explore the application of this method beyond time series generative modeling, potentially impacting a wider array of machine learning applications.
For more details, you can access the full research paper here.