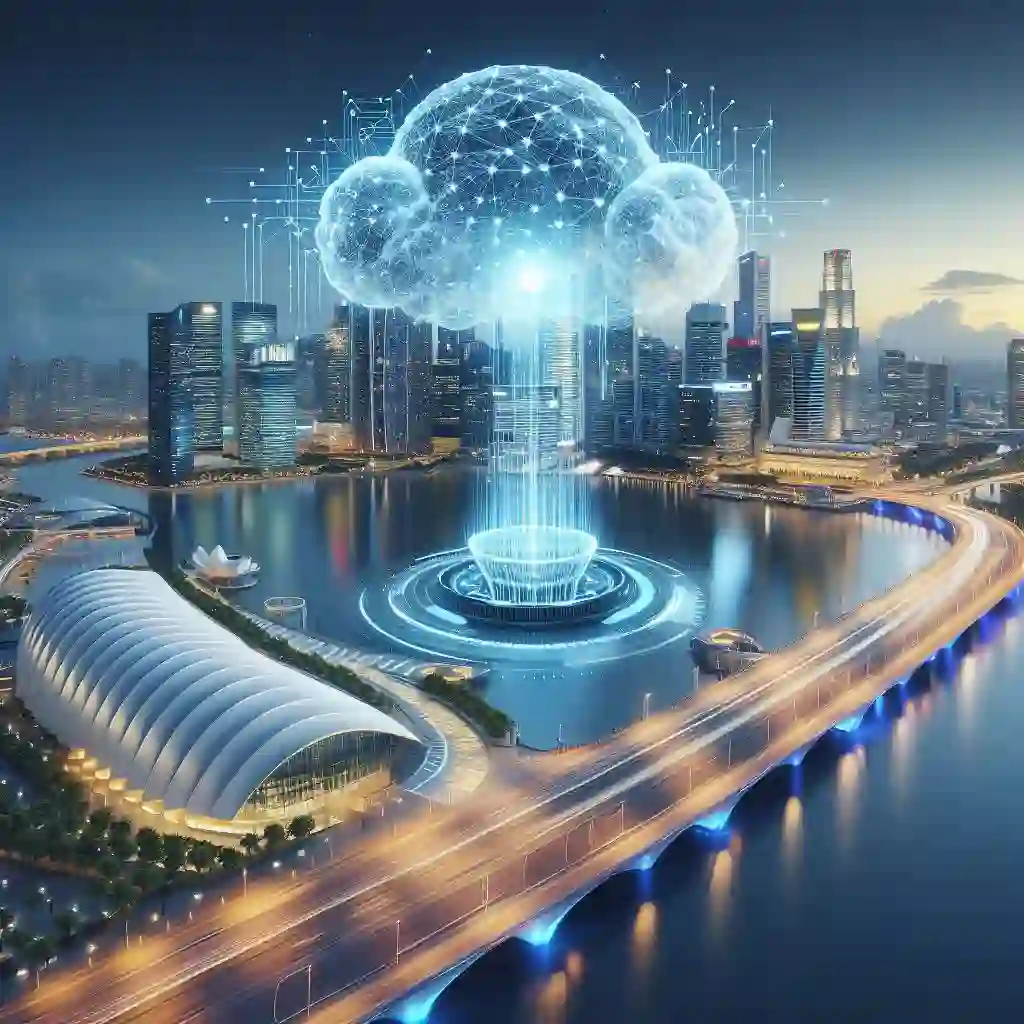
KAN: Redefining Machine Learning with Precision Data Analysis and Resource Efficiency
Kernel Attention Network Sets a New Benchmark for Intelligent Data Processing
The Kernel Attention Network (KAN) offers a groundbreaking shift in data processing, merging the efficiency of kernel methods with the flexibility of attention mechanisms. Its unique ability to discern critical information makes it an ideal successor to traditional Multi-Layer Perceptrons (MLPs). With successes in healthcare and computer vision, KAN is reshaping how we think about data analysis.
Key Takeaways
-
Advances in Brain Disorder Classification: The Diffusion Kernel Attention Network (DKAN) harnesses a non-linear attention mechanism to detect patterns in the brain, delivering precise classifications for ADHD and Alzheimer's disease. This promises more accurate diagnoses, giving patients and healthcare providers valuable early insights.
-
Optimized Scene Text Removal: MSLKANet excels in text removal from natural images while preserving the background's integrity. This enables accurate, efficient image processing, essential for privacy protection and document editing.
-
Resource Optimization: Unlike MLPs, KAN allocates computational power to the most pertinent data through selective attention. This results in faster, more accurate data analysis while minimizing resource expenditure.
Analysis
MLPs are widely used, but they can struggle to handle large-scale data efficiently due to their fully connected layers, often requiring significant computing power. KAN steps in with its kernel-based attention mechanism, smartly focusing on relevant data while discarding less useful information. This significantly enhances predictive analytics and early detection in fields like finance and healthcare.
By zeroing in on critical patterns across complex datasets, KAN aids financial market trend predictions, fine-tunes customer service chatbots, and offers enhanced sentiment analysis for understanding consumer preferences. The reduced computational burden and long-range data dependency management position KAN as a game-changer.
Did You Know?
- KAN's selective attention mechanism can fine-tune customer service responses by accurately identifying relevant customer feedback, helping businesses refine strategies.
- Its advanced pattern recognition capabilities can unlock new levels of market insight, transforming how companies predict and adapt to financial market trends.
Conclusion: KAN has ushered in a new era of data processing, efficiently handling complex data sets and reshaping business strategies across healthcare, finance, and customer service. By offering an innovative alternative to MLPs, it is paving the way for the next generation of machine learning tools.