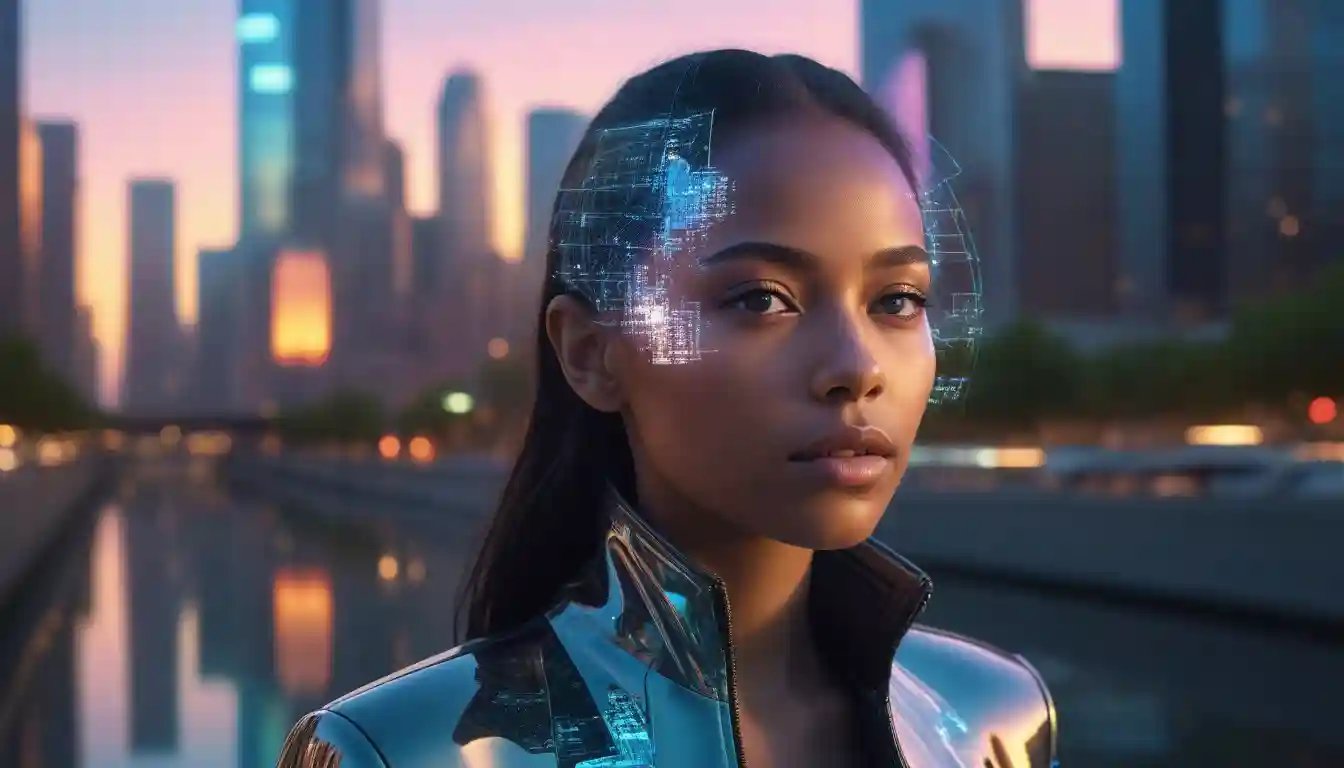
Liquid AI, a cutting-edge startup co-founded by renowned robotics expert Daniela Rus, has successfully secured an impressive $250 million in Series A funding led by semiconductor giant AMD. This substantial investment values the company at over $2 billion and signals a major leap forward in developing next-generation Artificial Intelligence (AI) models known as Liquid Foundation Models (LFMs). Built on the pioneering concept of liquid neural networks, these LFMs promise heightened efficiency, adaptability, and significantly reduced computational demands compared to traditional AI models. As Liquid AI partners strategically with AMD, it aims to reshape the AI landscape by delivering scalable, resource-efficient intelligence across various high-growth industries—ultimately challenging the dominance of current AI powerhouses.
Liquid AI: Funding and Vision
Liquid AI’s substantial $250 million Series A funding round not only underscores investor confidence but also sets the stage for robust innovation in the AI sector. The capital infusion—led by AMD—propels the startup toward rapid scaling of its Liquid Foundation Models, enabling the development of smaller, more adaptable, and resource-efficient AI tools. Valued at over $2 billion, Liquid AI’s approach centers on the idea that cutting-edge neural architectures can power everything from mobile devices to complex data centers, all while reducing computational overhead and energy costs.
Key Features of Liquid Neural Networks
Liquid neural networks form the core technology behind Liquid AI’s offerings, providing a host of advantages over traditional models:
-
Efficiency: LFMs demand less computing power, resulting in lower energy consumption and a reduced memory footprint. This efficiency makes them an attractive alternative for companies looking to curb operational costs while maintaining top-tier AI performance.
-
Flexibility: Drawing inspiration from the neural structure of roundworms, these networks excel at continuous adaptation. By processing time-series data and adjusting to new inputs in real time, liquid neural networks enable applications like autonomous driving, robotics, and IoT devices to learn continuously and respond effectively to evolving conditions.
-
Smaller Size: In stark contrast to large, resource-hungry models, LFMs can achieve similar—or even superior—performance with significantly fewer parameters. For example, certain tasks that conventionally require tens of thousands of neurons can be handled by under a hundred neurons in a liquid neural network, dramatically decreasing complexity and cost.
Liquid AI’s Offerings: Tailored LFM Models
Liquid AI has developed three distinct model sizes to address a wide range of computing scenarios:
-
LFM-1B: A 1.3 billion-parameter model engineered for on-device applications, including smartphones and embedded systems. This model delivers AI capabilities directly at the edge, ensuring low latency and energy efficiency.
-
LFM-3B: With 3.1 billion parameters, this model is optimized for edge deployments requiring slightly more computational power. It suits mid-range devices, edge servers, and decentralized computing environments where both performance and efficiency are critical.
-
LFM-40B Mixture of Experts: Specifically designed for more complex tasks, this model leverages a mixture-of-experts approach to tackle intricate problems. Its larger parameter count supports advanced applications such as complex data analytics, real-time financial modeling, or sophisticated biotechnology research.
Strategic Partnership with AMD
A cornerstone of this funding round is the strategic alignment between Liquid AI and AMD:
-
Hardware Optimization: By collaborating closely with AMD, Liquid AI aims to fine-tune its LFMs for AMD’s powerful GPUs, CPUs, and AI accelerators. This integration ensures that the next generation of LFMs will deliver exceptional performance on cutting-edge hardware platforms.
-
Infrastructure Scaling: The partnership lays a strong foundation for accelerating infrastructure expansion, empowering Liquid AI to broaden the scope and scale of its LFM deployments. This synergy could challenge dominant players in the market by offering efficient, high-performance AI solutions that run seamlessly on AMD hardware.
Industry Applications of LFMs
Liquid AI envisions its LFMs making significant inroads across diverse sectors, including:
- E-commerce: Personalization engines, inventory management, and dynamic pricing can benefit from LFMs’ real-time adaptation and efficiency.
- Consumer Electronics: On-device AI intelligence for smartphones, wearables, and smart home devices, reducing the need for cloud-based computations.
- Biotechnology: Enhanced modeling for drug discovery, protein folding, and genomic analysis, all while cutting down on computational intensity.
- Telecommunications: Improved network monitoring, predictive maintenance, and customer experience optimization.
- Financial Services: Real-time risk assessment, fraud detection, and algorithmic trading powered by resource-friendly yet high-performing AI models.
Responses from Experts: Supportive and Critical Perspectives
Supportive Perspectives:
- Efficiency and Adaptability: Advocates highlight how liquid neural networks offer continuous learning and adaptability, making them ideal for dynamic fields like autonomous driving. Their ability to adjust parameters in real time can revolutionize sectors that demand immediate, responsive decision-making.
- Resource Optimization: Supporters emphasize that liquid neural networks can produce comparable results to traditional models using far fewer neurons. This translates to lower computational costs, reduced energy consumption, and an overall more sustainable AI ecosystem.
- Strategic Collaboration: Industry observers praise the Liquid AI–AMD partnership, anticipating that hardware-software synergy will amplify LFMs’ capabilities, encourage faster innovation, and drive broader commercial adoption.
Critical Perspectives:
- Limited Research Base: Critics caution that liquid neural networks remain a relatively new concept. Limited academic literature and real-world case studies mean that widespread adoption may be premature until more robust evidence of scalability and reliability emerges.
- Parameter Tuning Challenges: Fine-tuning neural networks remains a complex, time-intensive process. For liquid neural networks, improper parameter settings can yield suboptimal performance, potentially undermining their touted efficiency benefits.
- Applicability Constraints: While liquid neural networks excel at processing continuous data streams, they may not be as effective for static datasets. This limitation suggests certain sectors or applications might find conventional models more suitable.
Predictions and Market Outlook
Market Impact and Positioning:
Liquid AI’s $250 million funding and innovative LFMs could disrupt the current AI landscape. By delivering models that are both adaptive and less resource-intensive, Liquid AI may challenge industry behemoths like OpenAI and Google’s DeepMind. If widely adopted, LFMs could trigger a shift away from massive, energy-hungry models toward leaner, more efficient solutions.
Strategic Stakeholders:
- AMD’s Role: As AMD integrates LFMs into its GPUs, CPUs, and accelerators, it positions itself as a formidable contender against rivals like Nvidia. This hardware-software synergy could shape the future of AI computing hardware.
- Clients and Competitors: Industries already facing high computational costs—like biotech, telecom, and finance—stand to gain significantly. Meanwhile, competitors may scramble to develop similar architectures or strike similar partnerships to remain relevant.
Industry Trends and Broader Implications:
- Decentralized AI: As LFMs bring AI intelligence closer to the data source, decentralization—running powerful models on edge devices—will likely become a dominant industry theme.
- Environmental and ESG Considerations: Reduced energy usage in LFMs aligns with environmental, social, and governance (ESG) goals, making Liquid AI’s technology appealing to eco-conscious investors.
- Educational and Regulatory Aspects: Successful LFM deployment demands new skill sets and training programs. Additionally, the rapid evolution of adaptable AI models outpacing current regulations may spark debates on ethical AI use, data privacy, and oversight.
Speculative Outlook:
If Liquid AI’s technology scales smoothly and demonstrates tangible performance benefits, it could revolutionize key markets that depend on agility and low-latency decision-making. However, potential stumbling blocks—like validation challenges and parameter tuning complexities—could slow adoption. Still, many investors remain cautiously optimistic, viewing LFMs as a catalyst for a more efficient, sustainable, and widely accessible AI future.
Conclusion
Liquid AI’s breakthrough funding round, bold technological vision, and strategic partnership with AMD herald a new chapter in AI’s evolution. By championing liquid neural networks and their Liquid Foundation Models, Liquid AI aims to bring forth a paradigm shift—one that emphasizes efficiency, adaptability, and real-world scalability. As industries explore the promise of these models, the next few years will determine whether LFMs can truly redefine the boundaries of artificial intelligence or remain an ambitious experiment in the ever-competitive world of AI innovation.