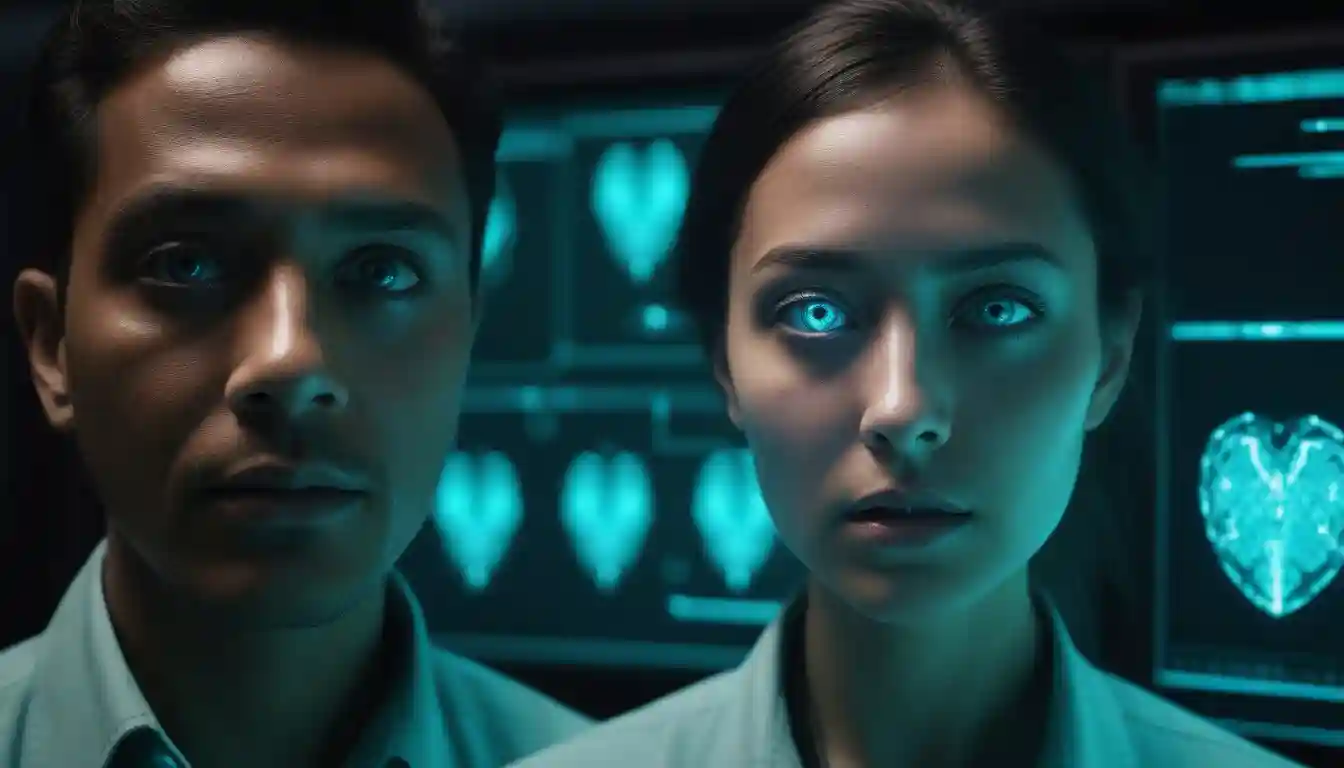
Meta Unveils Next-Gen AI Inference Chip
Meta Launches Next-Generation AI Inference Accelerator, MTIA
Meta has revealed its latest AI inference accelerator, the Meta Training and Inference Accelerator (MTIA), developed to improve recommendation models for Facebook and Instagram. The new MTIA chip, constructed using TSMC's 5nm process, delivers a 3.5x performance boost over its predecessor, featuring 177 TFLOPS at BF16 and 354 TOPS (INT8). It incorporates hardware-based tensor quantization for FP32 accuracy and optimized PyTorch support, facilitating swift job launches and replacements. Additionally, the chip operates at 1.35 GHz with a 90-watt TDP, includes 128GB LPDDR5 memory, and is powered by RISC-V cores. Meta's presentation at the Hot Chips symposium highlighted the chip's efficiency improvements and its potential to decrease reliance on Nvidia GPUs, although it remains tight-lipped about future memory expansion options.
Key Takeaways
- Meta's next-gen MTIA chip enhances GEMM TOPs by 3.5x to 177 TFLOPS at BF16.
- The chip, built on TSMC's 5nm process, provides 354 TOPS (INT8) and 177 TOPS (FP16).
- Meta aims to enhance performance per TCO and per watt compared to the previous generation.
- MTIA chip features RISC-V cores and dual CPUs, running at 1.35 GHz with a 90-watt TDP.
- Meta hints at potential memory expansion via PCIe switch, though not currently deployed.
Analysis
Meta's launch of the MTIA chip could disrupt the AI accelerator market, potentially diminishing reliance on Nvidia. In the short-term, TSMC and RISC-V stand to benefit from increased demand. In the long term, Meta's cost efficiency and performance gains may induce competitors to innovate. Investors in Nvidia may encounter volatility, whereas Meta's stock could experience a surge. This move underscores Meta's commitment to AI, potentially catalyzing broader industry shifts in hardware and software optimization.
Did You Know?
- **MTIA (Meta Training and Inference Accelerator)**:
- **Explanation**: MTIA is Meta's custom-designed AI inference accelerator chip, specifically engineered to enhance recommendation models for platforms like Facebook and Instagram. It is built on TSMC's advanced 5nm process technology, offering significant performance improvements over its predecessor. The chip is optimized for tasks such as matrix multiplication (GEMM) and supports various precision levels (BF16, INT8, FP16), making it highly efficient for AI workloads.
- **BF16 (Brain Floating Point 16-bit)**:
- **Explanation**: BF16 is a floating-point format that uses 16 bits to represent a number, similar to FP16 (Half-Precision Floating Point). However, BF16 has a larger exponent range compared to FP16, which makes it more suitable for deep learning tasks where maintaining precision is crucial. The MTIA chip achieves 177 TFLOPS (Tera Floating Point Operations Per Second) at BF16 precision, indicating its high computational efficiency in AI inference tasks.
- **RISC-V Cores**:
- **Explanation**: RISC-V is an open standard instruction set architecture (ISA) based on established reduced instruction set computer (RISC) principles. Unlike proprietary architectures like ARM or x86, RISC-V is open-source, allowing for greater flexibility and customization in chip design. The MTIA chip incorporates RISC-V cores, which are optimized for specific tasks within the chip, contributing to its overall efficiency and performance.