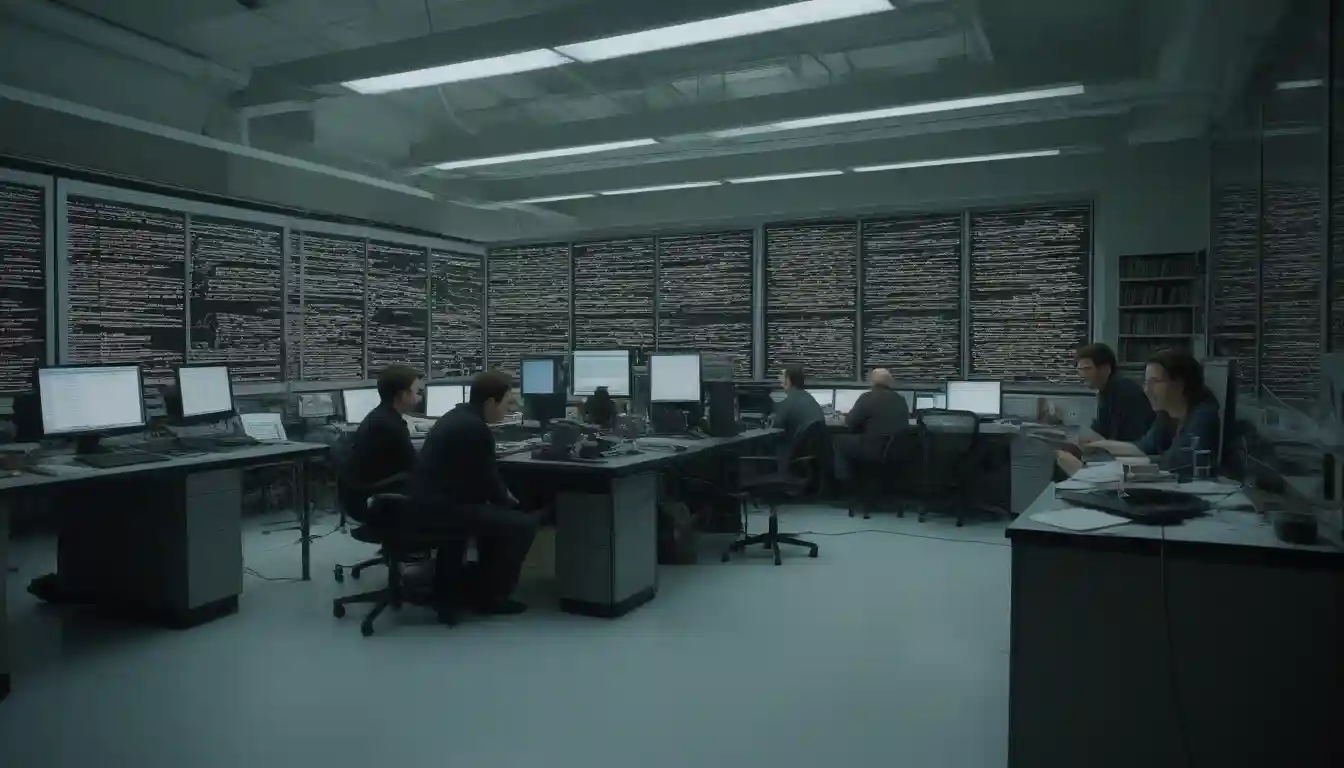
MIT Research Reveals Emergent Understanding in Language Models
Large Language Models (LLMs): Uncovering Their Emergent Understanding of the World
Researchers at MIT have made a groundbreaking discovery regarding large language models (LLMs). Their study reveals that as these models' language skills improve, they may develop a deeper understanding of the world, going beyond mere statistical correlations to potentially form internal models of reality. This suggests an emergent ability of LLMs to interpret and develop a formal understanding of the environments they are trained to navigate.
The research is conducted by MIT's Computer Science and Artificial Intelligence Laboratory (CSAIL). The study explores how large language models (LLMs) may go beyond statistical correlations to potentially develop an internal model of reality. By training LLMs on tasks like Karel puzzles, where they generate instructions for robot navigation without being explicitly shown how the instructions work, the researchers found that the LLMs spontaneously developed an understanding of the underlying simulation. This suggests that LLMs may be capable of forming an internal representation of the environments they navigate, even without direct exposure during training. The study used a probing technique to examine the LLMs' thought process, revealing that the model's ability to generate correct instructions improved significantly during training.
The research further indicates that LLMs might be learning something deeper about language than previously thought. To test this, the researchers introduced a "Bizarro World" where the meanings of the instructions were flipped, and found that the LLM's original understanding of instructions was preserved, indicating that it had internalized the correct semantics independently of the probing classifier. While this study provides evidence that LLMs can develop an understanding of reality, the researchers acknowledge limitations, such as the simplicity of the programming language and the small model size used. Future work will explore more complex settings to refine these insights and further understand how LLMs might use their internal models for reasoning.
Key Takeaways
- MIT researchers suggest LLMs may develop world understanding as language skills improve.
- Training LLMs on synthetic programs reveals emergent ability to interpret hidden states.
- Probing classifier extracts accurate representations from LM's hidden states.
- OthelloGPT experiment shows internal "world model" in LLMs for decision-making.
- Study challenges notion that LLMs are merely "stochastic parrots," proposing internal models.
Analysis
The MIT study on LLMs developing internal world models could significantly impact AI research and development. This could lead to more accurate AI applications in the short term and potentially redefine AI's role in society in the long term, emphasizing ethical considerations and transparency in AI decision-making processes. Direct and indirect beneficiaries of this breakthrough include AI companies, tech giants, and industries relying on AI for complex problem-solving.
Did You Know?
- Large Language Models (LLMs):
- Definition: Advanced AI systems designed to understand and generate human-like text.
- Functionality: Process vast amounts of text data for translations, summarizations, and complex reasoning.
- Emergent Capabilities: Recent studies suggest they may develop a deeper understanding of the world.
- Probing Classifier:
- Definition: A tool used in machine learning to analyze the representations learned by a model.
- Purpose: Understand the information encoded in the intermediate layers of a neural network.
- Application in LLMs: Reveals if the model has developed an internal representation of hidden states or concepts.
- Internal "World Model" in LLMs:
- Concept: Hypothetical representation that LLMs might develop internally to understand and interact with the environment.
- Evidence: Experiments suggest LLMs can develop such internal models.
- Implications: Challenges the view that LLMs are merely "stochastic parrots" and suggests they might develop a meaningful understanding of the reality they interpret.