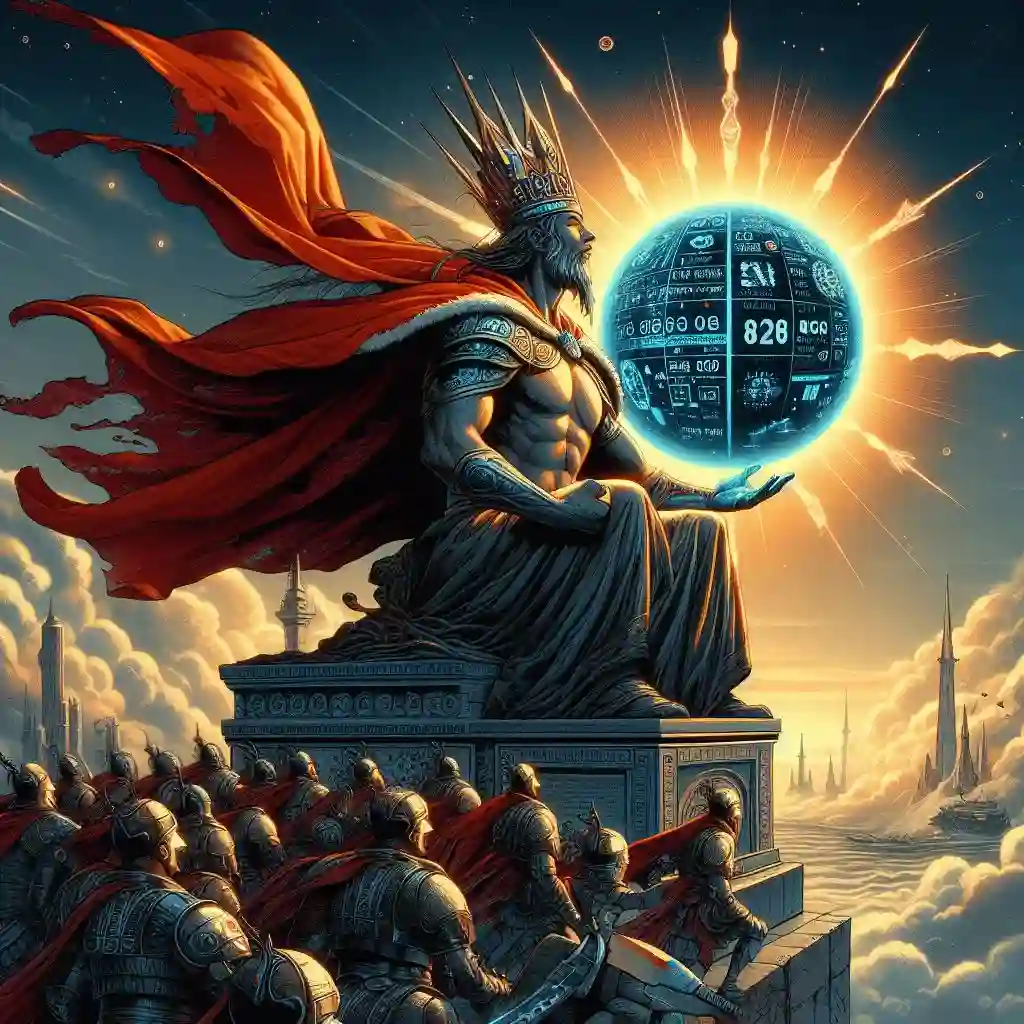
Mixtral 8x22B Shows Promising Results in Benchmark Tests: King of the Open Source LLMs
In the latest round of AI benchmark tests, the Mixtral 8x22B model has displayed impressive capabilities, outperforming several competitors in key areas of reasoning and comprehension. Despite facing stiff competition from top-tier models like GPT-4 and Claude 3 Opus, Mixtral 8x22B has proven to be a formidable contender in the AI landscape. Additionally, at CTOL.digital we tested Mixtral 8x22B model using our proprietary test set CTOL-HUMAN-F1, it achieved the higest score beating all the other open source models like Mixtral 7x8B, Llama 70B.
Key Takeaways:
- Mixtral 8x22B beats all the other open source models on our proprietary test set CTOL-HUMAN-F1
- Mixtral 8x22B excelled in the PIQA and BoolQ tasks, reflecting strong physical commonsense reasoning and factual understanding, with accuracy scores above 83%.
- The model showed decent performance in science and elementary reasoning tasks (ARC Challenge and ARC Easy) with scores around 59% and 86%, respectively.
- Mixtral 8x22B struggled in the OpenBookQA task, indicating a potential area for improvement in open-domain knowledge application.
- Compared to other AI models, Mixtral 8x22B holds its own, especially in the Hellaswag and Winogrande tasks, with scores just shy of those achieved by advanced models like GPT-4.
Analysis: Mixtral 8x22B's varied performance highlights the nuanced landscape of AI capabilities where different models have distinct strengths and weaknesses. The model's strong showing in structured tasks suggests a solid foundation in logic and reasoning within specific contexts. However, the results also pinpoint the challenges in developing AI that can seamlessly apply knowledge across a broad spectrum of topics and question types. The benchmarks underscore the continuous need for advancement in AI models to improve their generalization abilities and adaptability.
Did You Know?
- Benchmark tests like those conducted for Mixtral 8x22B and its contemporaries are essential for measuring the progression of AI. They provide a standardized way to compare different models' abilities to reason, understand, and interact with the world.
- The tasks these models are tested on, such as the PIQA, BoolQ, and Winogrande, are designed to mimic complex human thought processes, from simple Q&A to deep comprehension and reasoning challenges.
- AI models' performance can fluctuate significantly based on their design, training, and the nature of the tasks, revealing much about the future potential and current limitations of artificial intelligence.
- CTOL-HUMAN-F1: CTOL.digital's own test set that evaluates a large language model's capability in Cognitive Abilities, Learning and Adaptation, Emotional Intelligence, Creativity and Divergent Thinking, Practical Intelligence, Social Intelligence, Cultural Context and more. The test set is fully private in order to provide a more object evaluation on LLMs (Many LLMs are trained on public evaluation data to rank higher on the leaderboard)