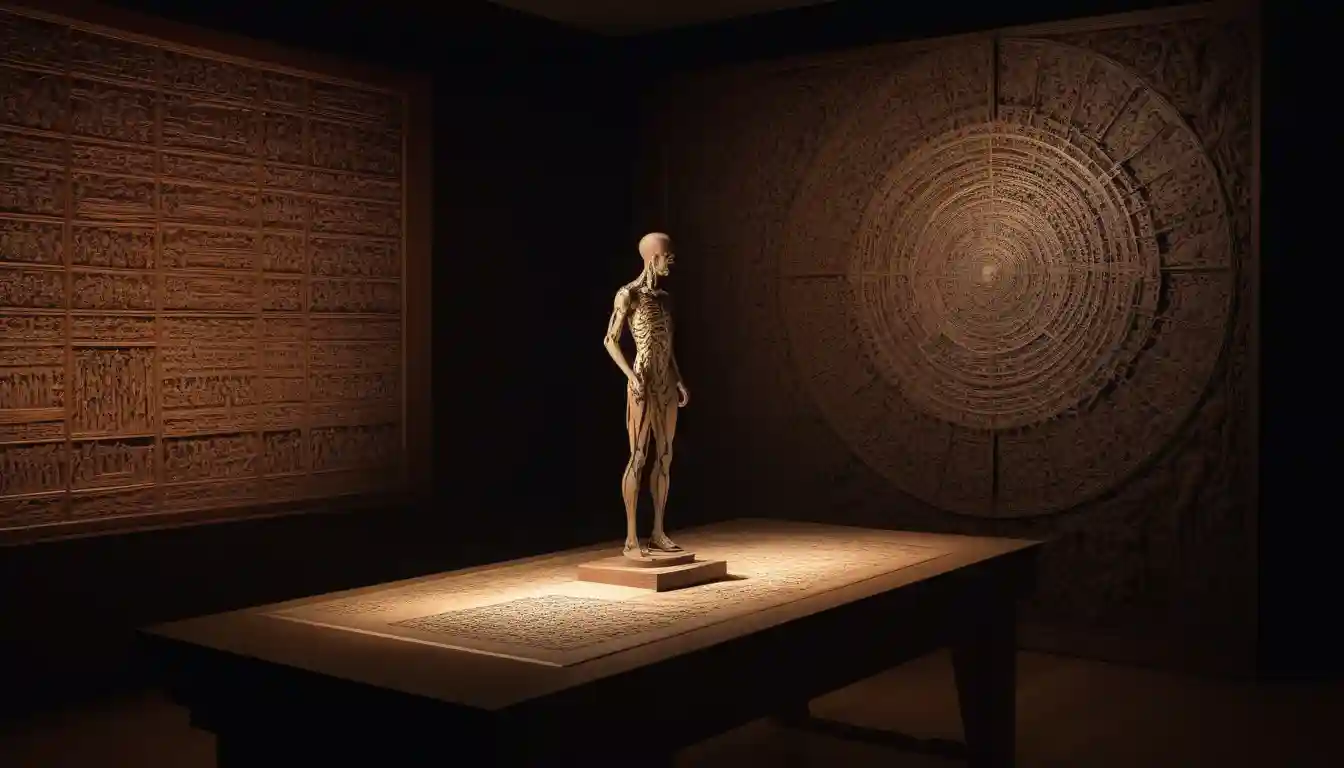
NeedleBench Reveals Major Flaws in AI's Ability to Comprehend Long Texts
Large language models (LLMs) are renowned for their data processing capabilities, yet they face significant challenges in understanding lengthy texts, as revealed by the "Needle In A Haystack" (NIAH) benchmark. This benchmark, used by industry leaders like Google and Anthropic, shows that while LLMs excel at finding information in long texts, they struggle with comprehending the full context. To address this, researchers from the Shanghai AI Laboratory and Tsinghua University have developed NeedleBench, a bilingual benchmark aimed at evaluating LLMs' contextual capabilities more thoroughly. NeedleBench includes tasks that assess information extraction and reasoning within long texts of varying lengths.
One significant task within NeedleBench, the Multi-Needle Reasoning Task (M-RS), challenges models to draw conclusions from dispersed information in large documents, highlighting a gap between retrieval and reasoning abilities among open-source models. The Ancestral Trace Challenge (ATC) was created to test context-dependent performance, particularly in kinship relationships. Although models like GPT-4-Turbo and Claude 3 showed strong performance, they struggled with increased data and complexity. The open-source model DeepSeek-67B also demonstrated notable capabilities. Despite claims of processing over a million tokens, NeedleBench reveals that LLMs face limitations in extracting complex information from long texts, even with just a few thousand tokens, emphasizing the need for more nuanced evaluations of LLM capabilities in real-world tasks involving large data volumes. The study concludes that LLMs require substantial improvement in handling complex logical reasoning challenges and notes that open-source models perform better when source content precedes the prompt, with chain-of-thought prompting enhancing results.
Key Takeaways
- LLMs encounter challenges in understanding lengthy texts beyond basic data retrieval.
- NeedleBench provides comprehensive evaluation of LLMs' contextual comprehension and summarization abilities.
- GPT-4-Turbo and Claude 3 demonstrate proficiency in complex reasoning but experience limitations with increased data.
- The open-source model DeepSeek-67B excels in multi-level logical challenges.
- Significant improvement is needed in LLMs for practical tasks involving large data and complex reasoning.
Analysis
The introduction of NeedleBench sheds light on the limitations of LLMs in long-context reasoning, which could have implications for tech giants like Google and Anthropic. In the short term, this may hamper the deployment of LLMs in complex applications, while in the long term, it could drive innovation in LLM architecture and training methods, benefiting sectors reliant on deep contextual understanding. This development might also contribute to volatility in financial instruments linked to AI advancements. Additionally, open-source models like DeepSeek-67B are positioned to gain prominence, potentially influencing market dynamics and investment trends.
Did You Know?
- Needle In A Haystack (NIAH) Benchmark: This specialized testing framework, employed by major tech companies like Google and Anthropic, evaluates the performance of Large Language Models (LLMs) in specific information extraction tasks from extensive texts. It emphasizes the proficiency of models in locating data within long documents and also highlights their limitations in fully comprehending the broader context of the retrieved information.
- NeedleBench: Developed by researchers at the Shanghai AI Laboratory and Tsinghua University, NeedleBench is a bilingual benchmark that comprehensively evaluates the contextual capabilities of LLMs. It encompasses tasks beyond simple information retrieval, focusing on the models' ability to extract and reason about information in long texts across various length intervals. This benchmark is crucial for understanding the practical limitations and potential of LLMs in real-world applications involving large volumes of complex data.
- Ancestral Trace Challenge (ATC): A specific test within the NeedleBench framework, the ATC evaluates LLMs' performance in understanding and reasoning about context-dependent relationships, particularly kinship relationships. This challenge is crucial for assessing the models' ability to handle complex, interrelated information, showcasing their capacity to maintain and utilize contextual understanding in intricate scenarios.