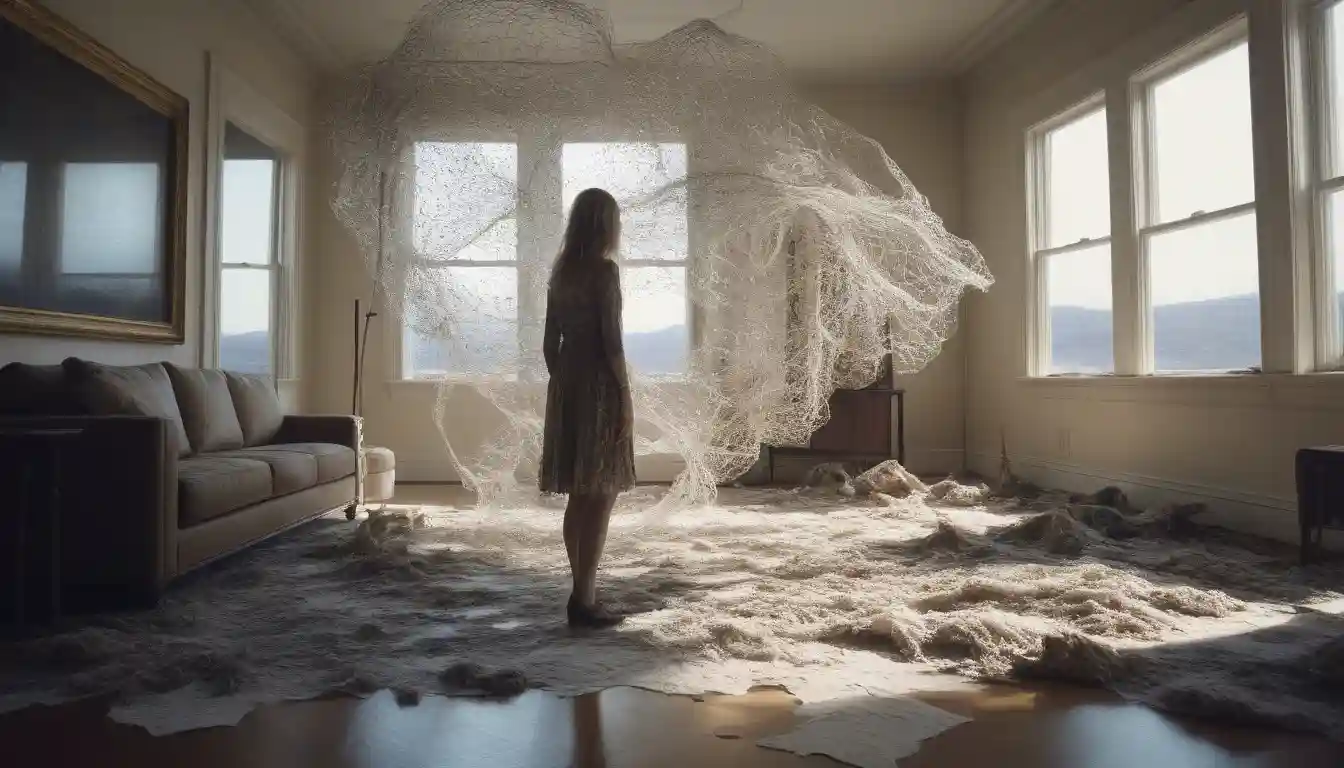
New Speculative RAG Method Enhances Retrieval Augmented Generation Systems
Speculative RAG: Revolutionizing Language Models for Enhanced Efficiency and Accuracy
Researchers have unveiled a groundbreaking method, "Speculative RAG," designed to revolutionize Retrieval Augmented Generation (RAG) systems. This innovative approach integrates two distinct language models to boost efficiency and accuracy in processing large language models (LLMs), thereby minimizing errors and "hallucinations."
The Speculative RAG method introduces a smaller, specialized model known as "RAG Drafter," which simultaneously generates multiple high-quality answer options from different subsets of retrieved documents. This specialized model is specifically trained for question-answer-document relationships. Additionally, a larger, general-purpose model, "RAG Verifier," scrutinizes and selects the most accurate answer among the options generated by the RAG Drafter.
An extensive evaluation conducted by the University of California and Google showcased impressive results. The tests revealed that Speculative RAG achieved up to 12.97% higher accuracy while reducing latency by 51% compared to traditional RAG systems. Notably, this dual-model system significantly enhances efficiency and accuracy in AI interactions.
The potential impact of this approach is substantial, particularly within the realm of AI technology providers and users. It not only enhances product efficiency and reliability for tech firms specializing in AI but also has the potential to accelerate AI adoption across critical sectors such as healthcare and finance, where accuracy is of paramount importance. Moreover, this could potentially drive increased research and development investments in AI models, ultimately reshaping industry standards for AI performance.
Key Takeaways
- "Speculative RAG" combines a smaller "RAG Drafter" and a larger "RAG Verifier" to enhance RAG efficiency.
- The "RAG Drafter" simultaneously generates multiple answer suggestions, reducing input tokens.
- The "RAG Verifier" selects the best answer, improving accuracy without lengthy context processing.
- Speculative RAG demonstrated up to 12.97% higher accuracy and 51% lower latency in benchmark tests.
- This dual-model approach aims to make RAG systems more efficient for knowledge-intensive tasks.
Analysis
The introduction of Speculative RAG by the University of California and Google is poised to significantly impact AI technology providers and users. The dual-model approach effectively addresses latency and error issues, potentially prompting competitors to innovate similarly. This, in turn, is expected to drive increased research and development investments in AI models and redefine industry standards for AI performance. This innovation could also accelerate AI adoption across sectors where accuracy is critical, such as healthcare and finance.
Did You Know?
- **Speculative RAG**:
- **Explanation**: Speculative RAG is a novel method that enhances Retrieval Augmented Generation (RAG) systems by integrating two distinct language models to improve efficiency and accuracy. It leverages a smaller, specialized model called "RAG Drafter" to generate multiple high-quality answer options from different subsets of retrieved documents, and a larger, general-purpose model named "RAG Verifier" to select the most accurate answer. This approach significantly reduces latency and enhances accuracy in processing large language models.
- **RAG Drafter**:
- **Explanation**: The RAG Drafter is a smaller, specialized language model designed to generate multiple high-quality answer options simultaneously from different subsets of retrieved documents. It is trained specifically for question-answer-document relationships, allowing it to produce a range of plausible answers quickly, thereby reducing input tokens and speeding up the overall response time in RAG systems.
- **RAG Verifier**:
- **Explanation**: The RAG Verifier is a larger, general-purpose language model that reviews the multiple answer options generated by the RAG Drafter. Its primary function is to select the most accurate answer from the options provided, thereby improving the overall accuracy of the system without requiring lengthy context processing. This model ensures that the final output is both reliable and precise, enhancing the performance of the Speculative RAG method.