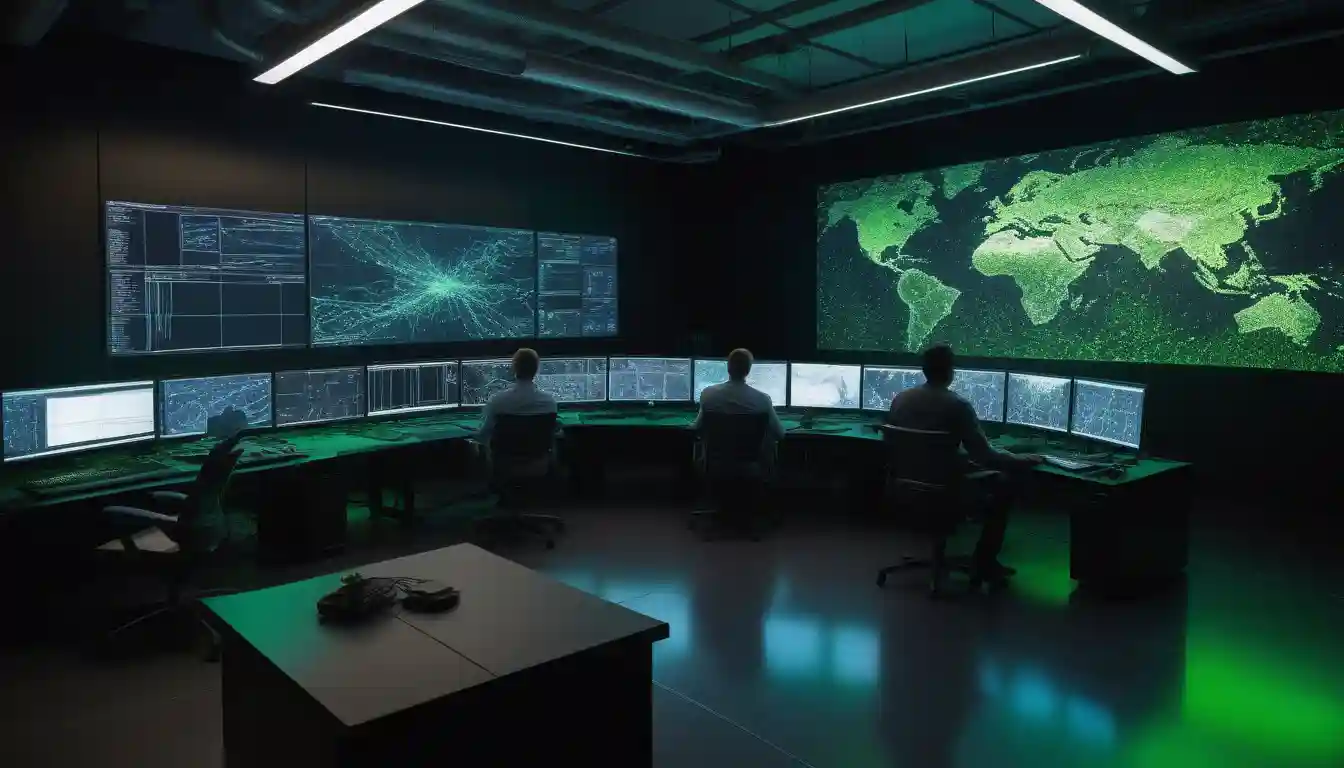
Nvidia’s Blackwell GPUs Redefine AI Performance: 2.2x Speed Boost Shakes Up Industry Benchmarks
Nvidia’s Blackwell Platform Shatters Performance Records in MLPerf Training 4.1 Benchmarks
Nvidia has once again set a new benchmark in AI hardware performance with the unveiling of its latest Blackwell platform. Showcasing significant advancements in the MLPerf Training 4.1 benchmarks, Blackwell offers substantial gains over the previous Hopper generation, making it a milestone in AI computing and machine learning infrastructure. The results were released in early November and have generated substantial excitement in both the AI and technology communities.
What Happened?
Nvidia presented its new Blackwell platform's results at the MLPerf Training 4.1 benchmarks, demonstrating massive performance gains across a variety of AI model training tasks. According to Nvidia, Blackwell GPUs delivered up to 2.2 times more performance per GPU than their predecessors, Hopper, in key benchmarks like Llama 2 70B fine-tuning and GPT-3 175B pre-training. The platform also achieved a 1.7x improvement in Stable Diffusion v2 training.
The architectural innovations that enable these gains include more efficient Tensor Core kernels and the integration of faster high-bandwidth memory (HBM3e). Nvidia also highlighted a critical efficiency milestone: GPT-3 175B pre-training, which previously required 256 Hopper GPUs, can now be executed on just 64 Blackwell GPUs, slashing hardware demands and potentially reducing costs.
The results are part of a broader trend, with Nvidia emphasizing the industry's shift towards larger and more complex AI models that need efficient and scalable hardware solutions. Nvidia’s plans to release an even more powerful variant, Blackwell Ultra, next year, with enhanced memory and compute capabilities, suggest that the AI hardware race is far from over.
Key Takeaways
- Performance Leap: The Blackwell platform delivered up to 2.2x the performance of Hopper in critical AI benchmarks like Llama 2 and GPT-3.
- Efficient Hardware Use: Blackwell’s architecture allows for running large models, such as GPT-3 175B, on significantly fewer GPUs—64 compared to Hopper’s 256—reducing resource needs and operational expenses.
- Architectural Enhancements: Innovations include optimized Tensor Core utilization and high-speed HBM3e memory, leading to superior throughput and training efficiency.
- Scaling Records and Industry Implications: Nvidia also set a new scaling record with Hopper, using 11,616 GPUs for GPT-3 175B pre-training. The ripple effects of Blackwell’s launch could transform how companies approach AI infrastructure.
- Future Developments: Blackwell Ultra, expected next year, promises even more power and memory, underlining Nvidia’s commitment to staying ahead in AI hardware.
Deep Analysis
Nvidia’s Blackwell platform is more than just an upgrade; it represents a paradigm shift in AI hardware. The 2.2x performance improvement in tasks like fine-tuning Llama 2 and pre-training GPT-3 is not merely a statistical bump but a transformative change that can drastically cut costs and reduce energy consumption for AI development. Companies running large-scale AI models, like hyperscalers and enterprise clients, stand to benefit immensely, as these performance gains could make previously prohibitive projects feasible.
The new architectural features, especially the efficient use of Tensor Cores and HBM3e memory, are key enablers of these gains. The reduced hardware footprint for major models, such as using only 64 GPUs for GPT-3 175B pre-training, signifies a leap in efficiency. This has far-reaching implications for data centers, where power and space are at a premium. Lower hardware requirements mean reduced energy consumption, aligning with global sustainability goals while offering cost benefits.
However, Nvidia’s competitors like AMD and Intel will face new challenges. Blackwell’s success raises the entry barrier significantly in the AI hardware market. Nvidia's dominance could force these players to accelerate innovation or risk losing market share. Moreover, Nvidia’s continued advancements in both training and inference benchmarks ensure that the company stays ahead of the curve, solidifying its position as the leader in AI infrastructure.
The future release of Blackwell Ultra, with promises of greater memory and computing power, suggests that Nvidia is not resting on its laurels. The company seems committed to meeting the escalating demands of AI models that require real-time processing and high-efficiency training, from chatbots to autonomous systems. The industry-wide impact of these advancements will likely be seen in faster development cycles for AI-driven applications and more robust infrastructures tailored to Nvidia's ecosystem.
Did You Know?
- Nvidia’s Blackwell platform's use of FP4 precision in the MLPerf Inference v4.1 benchmark led to a performance improvement of up to 4x compared to the H100 GPU. Interestingly, FP4 precision achieves this boost without sacrificing result accuracy.
- The trend toward scaling inference-time computing, driven by low-latency needs in chatbots and real-time AI applications, underscores the growing importance of efficient and powerful hardware.
- Nvidia set a new record by using 11,616 Hopper GPUs to train GPT-3 175B, showcasing the company’s capability to scale up operations to unprecedented levels.
- The integration of HBM3e memory is part of Nvidia's strategy to address the ever-increasing data demands of AI models, ensuring faster and more reliable data throughput.
In summary, Nvidia's Blackwell platform is more than an impressive technological feat; it is a harbinger of what the future of AI infrastructure could look like. With reduced hardware needs, enhanced efficiency, and a clear path for future upgrades, Nvidia has set a new benchmark for the AI industry, shaping the technology landscape in ways that will be felt for years to come.