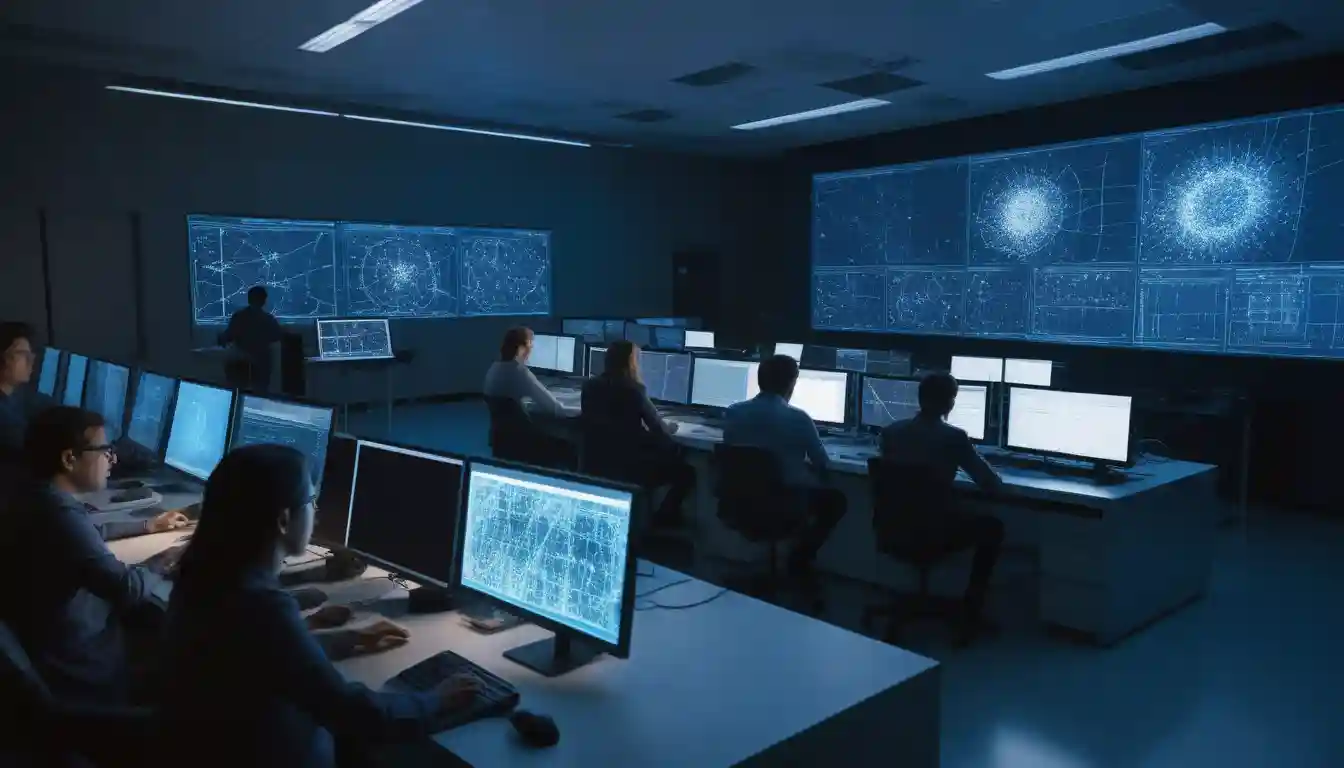
NVIDIA and Quantum Machines Break New Ground with Real-Time AI-Driven Qubit Calibration for Quantum Computing
NVIDIA and Quantum Machines Collaborate to Revolutionize Quantum Computing Calibration with Real-Time Reinforcement Learning
In a groundbreaking collaboration, NVIDIA and Quantum Machines have joined forces to address a major hurdle in quantum computing: the continuous calibration and control of qubits. By integrating NVIDIA's DGX Quantum platform with Quantum Machines' advanced control hardware, the partnership has successfully used reinforcement learning to enhance qubit control on Rigetti’s quantum chip. This achievement, revealed in late 2023, is seen as a step toward scalable, high-fidelity quantum computing that could improve the accuracy and efficiency of quantum circuits and pave the way for more reliable quantum applications.
The collaboration leverages the Twin Delayed Deep Deterministic Policy Gradient (TD3) reinforcement learning algorithm to calibrate "π pulses"—a key function in controlling qubit rotation with high precision. Unlike traditional static calibration, this approach allows for near real-time pulse adjustments, crucial in maintaining the stability of quantum systems over time. What’s impressive is the algorithm’s efficiency: implemented in only about 150 lines of code, it has already demonstrated its potential on basic quantum circuits and is theoretically scalable to handle larger, more complex systems.
This advancement is especially significant in light of quantum computing's ongoing battle with performance drift. Quantum systems tend to lose fidelity over time due to noise and environmental factors, necessitating regular recalibration. Historically, this recalibration has been a time-consuming, one-time process, creating a bottleneck in the advancement of error-corrected, fault-tolerant quantum computing. However, NVIDIA’s low-latency DGX platform makes it feasible to conduct these computations in near real-time, allowing for continuous calibration and, as a result, more reliable quantum operations.
Key Takeaways
- Dynamic Calibration Overcomes Drift: By enabling continuous calibration, NVIDIA and Quantum Machines address the challenge of performance drift, maintaining high fidelity essential for error correction.
- Reinforcement Learning in Quantum Computing: The use of the TD3 reinforcement learning algorithm to control qubit "π pulses" represents an efficient approach, implemented in only 150 lines of code, making it feasible for real-world applications.
- Scalability Promise: While still in testing on basic quantum circuits, the solution is theoretically scalable to more complex quantum systems, marking a potential leap toward fault-tolerant quantum computing.
- Limitations Acknowledged: Despite its promise, the TD3 algorithm wasn’t designed specifically for quantum systems, and scalability to large-scale quantum circuits may still face computational barriers.
Deep Analysis
The integration of NVIDIA's DGX Quantum platform with Quantum Machines' control hardware signifies a promising shift in quantum technology, especially concerning error correction—a critical requirement for quantum computers to become viable for large-scale applications. Error correction is heavily dependent on maintaining high fidelity in qubit states, and even minor deviations can cause exponential increases in computational errors. Traditional static calibration processes have often struggled to keep up with the rapid performance drift in quantum systems, which is compounded by factors like thermal fluctuations, external noise, and electromagnetic interference.
By using the TD3 reinforcement learning algorithm, NVIDIA and Quantum Machines have unlocked a way to adapt to these performance drifts dynamically. TD3, a reinforcement learning model designed for efficient decision-making, helps adjust qubit control pulses in real time. The algorithm calibrates "π pulses," responsible for qubit rotation accuracy, by using a feedback mechanism that continuously fine-tunes qubit control based on system performance. This novel approach means that quantum systems can retain high fidelity for longer periods, making them more robust and reliable.
Moreover, the low-latency capabilities of NVIDIA’s DGX Quantum platform play a crucial role here. Real-time pulse adjustments demand fast computations, which NVIDIA’s platform is optimized to handle, allowing for the quick adjustments necessary to keep quantum operations stable. This fusion of fast computation and adaptive calibration has led to successful testing on simple quantum circuits, with Quantum Machines and NVIDIA aiming to extend it to more complex circuits in the future.
However, this development is not without its limitations. The TD3 algorithm was not specifically created for quantum environments, which could impact its efficiency and adaptability as it scales to more complex circuits. Larger circuits demand more intensive real-time computation, and without further optimization or advancements in hardware, the model may encounter limitations that could hinder scalability. Despite this, the collaboration offers a significant proof of concept, showing that reinforcement learning, when combined with high-performance computing, holds real potential for overcoming some of the foundational challenges in quantum computing.
Did You Know?
-
Quantum Drift: Quantum computers are prone to "performance drift," a phenomenon where qubit fidelity degrades over time due to factors like environmental noise. This drift has historically required complex, time-intensive recalibration efforts, limiting quantum systems' reliability for extended computations.
-
TD3 Reinforcement Learning: The Twin Delayed Deep Deterministic Policy Gradient (TD3) algorithm, a reinforcement learning model initially designed for traditional machine learning applications, uses a feedback loop to optimize decision-making. In the context of this collaboration, TD3's feedback mechanism is repurposed to continually adjust "π pulses" for precise qubit control.
-
150 Lines of Code: The breakthrough solution for dynamic calibration was achieved with only about 150 lines of code, emphasizing the simplicity and efficiency of this innovative approach.
Limitations and Future Outlook
While the NVIDIA and Quantum Machines collaboration marks an exciting advancement, it also brings to light important limitations. The TD3 algorithm, though powerful, was not created with quantum computing's specific challenges in mind. Real-time reinforcement learning in quantum computing requires exceptionally high processing speeds, which NVIDIA’s DGX platform can support to some extent, but may still fall short when dealing with increasingly complex quantum circuits. The inherent scalability of this model remains promising but untested on a large scale, and the potential for unforeseen computational challenges is a factor.
Furthermore, the approach depends on robust, high-speed hardware, such as NVIDIA's low-latency DGX platform, which might not be widely accessible or feasible in all quantum setups. Therefore, while this proof of concept demonstrates that reinforcement learning can indeed enhance qubit control, fully scalable implementations will likely require additional advances in both hardware and algorithmic design.
Overall, the collaboration between NVIDIA and Quantum Machines is a noteworthy step forward, offering a fresh perspective on how continuous calibration might address quantum computing's performance drift challenge. However, moving from concept to large-scale application will require further breakthroughs, particularly in adapting machine learning algorithms specifically for quantum systems and developing hardware that can support high-speed, complex computations at scale.