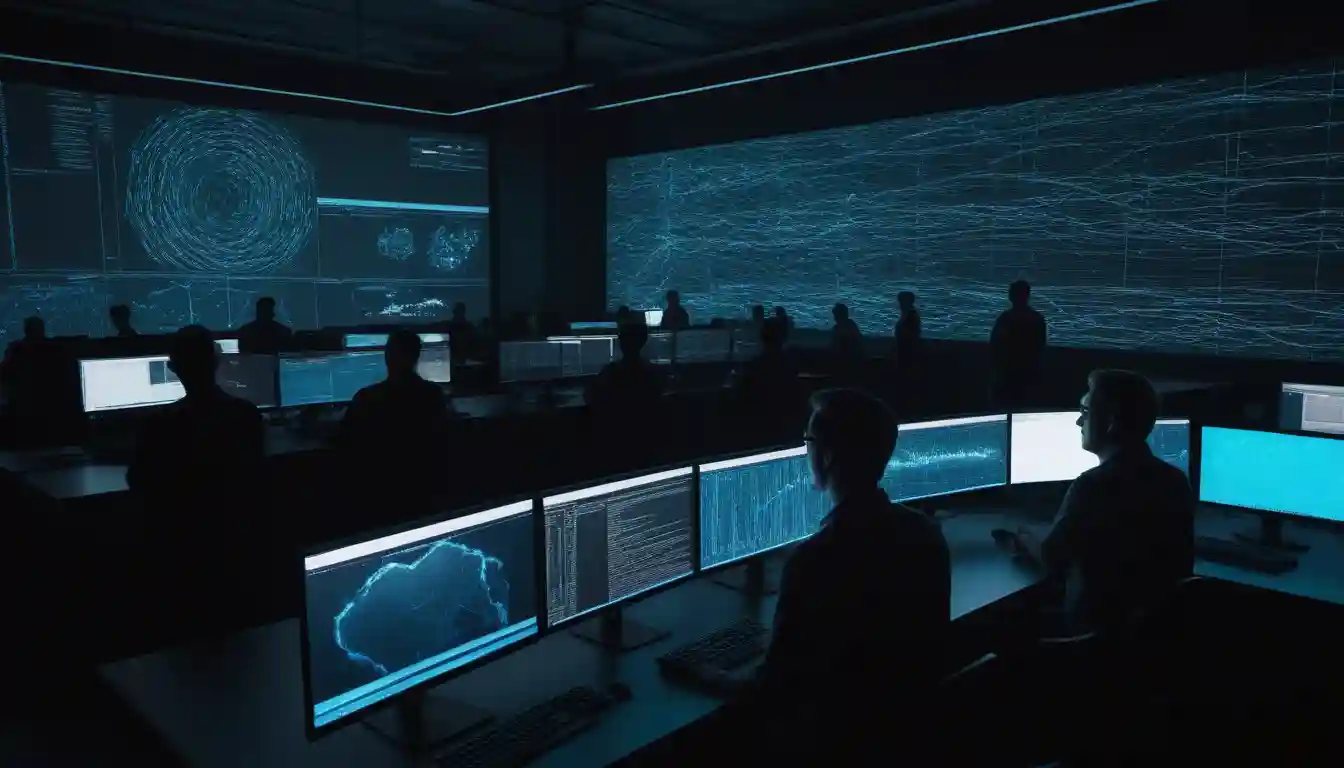
OpenAI has introduced a groundbreaking approach called Reinforcement Fine-Tuning (RFT), poised to significantly advance the capabilities of specialized AI systems across various sectors. This innovative training method differs from conventional supervised fine-tuning by enabling artificial intelligence models to develop their own problem-solving strategies, handle complex technical tasks, and excel with minimal initial data. As the AI market surges toward a projected $1.4 trillion by 2027, and industry leaders like Nvidia push boundaries with open-source multimodal large language models, RFT stands out as a powerful technique that not only improves efficiency but also addresses pressing challenges in accuracy, scalability, and ethical considerations. With early case studies already demonstrating remarkable results in fields as diverse as law, finance, engineering, insurance, and healthcare research, OpenAI’s RFT sets the stage for a new era of AI-driven innovation and domain-specific expertise.
OpenAI’s New Training Method
OpenAI’s Reinforcement Fine-Tuning (RFT) is a novel customization strategy designed to help AI models tackle complex, domain-specific tasks using remarkably few training examples—sometimes as few as a dozen. Unlike traditional supervised fine-tuning, which often leads models to merely replicate patterns from their training data, RFT encourages them to discover new ways of reasoning. This shift promotes true problem-solving capabilities over rote memorization.
To achieve this, RFT employs an evaluation system that rates the model’s output. Successful reasoning patterns are rewarded and reinforced, while incorrect or inefficient approaches are weakened. As a result, the model steadily refines its logic, becoming more adept at navigating challenging queries. This evolution makes RFT-driven models highly valuable for areas that demand exceptional precision and insight, such as legal analysis, financial modeling, engineering diagnostics, and insurance claim assessments.
Key Applications and Performance
RFT offers a transformative advantage for specialized fields. Traditional large AI models often require extensive training examples, which can be time-consuming and resource-intensive. By contrast, RFT-trained models learn more efficiently and adapt to niche problems without sacrificing accuracy. Their capacity to develop unique reasoning strategies allows them to outperform larger, standard models, even when operating at a smaller scale and with lower computational costs.
These performance gains are particularly beneficial in industry sectors that rely on highly accurate insights. Legal firms can use RFT-driven tools to interpret complex statutes or case law, engineering teams can simulate intricate system failures, financial analysts can detect subtle market patterns, and insurers can streamline claim review processes. The strong reasoning frameworks that RFT imparts empower these models to provide not only correct answers but also well-structured explanations for their conclusions.
Case Study – Thomson Reuters
A prime example of RFT’s potential is OpenAI’s collaboration with Thomson Reuters. Together, they developed an RFT-trained “o1 Mini” model tailored for legal applications. This specialized model functions as a legal assistant, parsing intricate legal texts, analyzing contractual nuances, and generating fact-based summaries. By focusing on reasoning rather than merely reproducing input data, this RFT-driven model helps legal professionals navigate large volumes of documents, identify relevant precedents, and ensure compliance—all while significantly reducing time and cost overheads.
Berkeley Lab Research
In another striking demonstration, Justin Reese, a computational biologist at Berkeley Lab, applied RFT to biomedical research. He curated data from hundreds of scientific papers to identify genes associated with rare genetic diseases. The RFT-trained o1 Mini model excelled in this domain, achieving up to 45% accuracy in pinpointing specific genes linked to particular conditions—far surpassing the performance of a standard o1 model.
Crucially, the RFT-driven model not only produced better results with less computational overhead but also offered clear explanations behind its predictions. This transparency is particularly valuable in medical research, where understanding the rationale behind a conclusion can guide further investigation, inform clinical decision-making, and bolster trust in AI-driven discoveries.
Deployment Plans
OpenAI is inviting organizations to join its Reinforcement Fine-Tuning Research Program, an alpha initiative aimed at refining and expanding RFT’s capabilities before a broader release. Participants will gain early access to the RFT API and the opportunity to provide feedback, shaping the evolution of this cutting-edge training methodology.
The wider public rollout of RFT is slated for early 2025. By then, a broader array of enterprises, academic institutions, and research organizations is expected to leverage RFT for highly customized AI solutions. As a result, these entities will be better equipped to address domain-specific challenges—from legal compliance and financial forecasting to intricate engineering diagnostics and rare disease research.
Comprehensive Analysis and Market Outlook
Industry experts anticipate that RFT will help drive the AI market’s explosive growth. By enabling smaller, more cost-effective models to outperform their larger counterparts in specialized tasks, organizations of all sizes can tap into advanced AI capabilities without the prohibitive hardware and software investments often required by conventional training methods.
At the same time, key players like Nvidia are working on open-source multimodal large language models, laying the groundwork for more accessible and energy-efficient AI solutions. However, along with these advancements comes the responsibility to manage computational demands sustainably, ensure model transparency, and mitigate potential biases. As governments and regulators pay closer attention to AI’s growing influence, frameworks around responsible data use, ethical deployment, and clear accountability will be essential.
Forward-looking scenarios envision RFT’s synergy with emerging technologies like quantum computing, potentially enabling real-time fine-tuning of even more complex models. In education, personalized learning experiences could arise from RFT-trained AI tutors, and in geopolitical contexts, strategic investments in RFT-enhanced solutions may reshape global technology leadership.
Still, as AI automates tasks in fields like law and healthcare, the workforce will face disruptions. Organizations and policymakers must prepare through reskilling initiatives and robust ethical guidelines. Balancing technological innovation with social responsibility will be key to achieving sustainable growth in this evolving ecosystem.
Conclusion
OpenAI’s Reinforcement Fine-Tuning method represents a pivotal advancement in AI training and deployment. It shifts the focus from data replication to creative reasoning, enabling smaller models to handle specialized, complex tasks with striking effectiveness. Early collaborations with Thomson Reuters and promising results in gene identification research highlight RFT’s immense potential.
As RFT heads toward a wider public release in early 2025, it promises to reshape entire industries. By democratizing access to high-level AI reasoning, fostering more efficient computational practices, and encouraging transparent decision-making, RFT stands poised to define a new standard for AI-driven solutions. In an era where sustainable innovation and ethical governance are paramount, OpenAI’s RFT offers a pathway to more intelligent, responsible, and impactful AI applications worldwide.