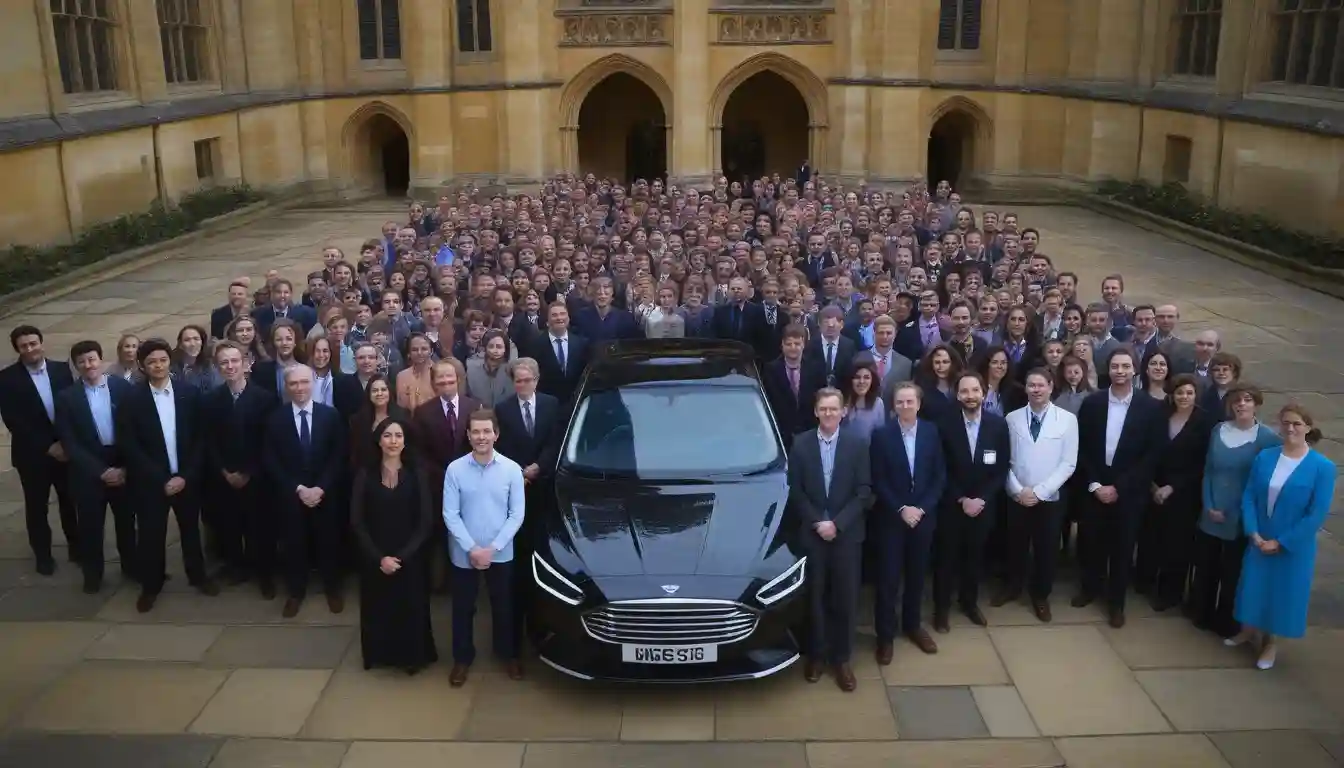
Oxford Unveils MedSAM-2: Revolutionary AI for Medical Image Segmentation
Oxford Unveils MedSAM-2 AI Model for Enhanced Medical Image Segmentation
Oxford researchers have revealed MedSAM-2, an advanced AI model aimed at improving the segmentation of both 2D and 3D medical images. This model, an extension of Meta's Segment Anything Model 2 (SAM 2), revolutionizes the treatment of medical images as video sequences, drastically reducing the workload for medical professionals by facilitating the processing of entire series with just one manual annotation.
MedSAM-2 introduces the "Confidence Memory Bank," which stores and references the most reliable predictions, thus elevating its accuracy in analyzing new images. A noteworthy feature, "one-prompt segmentation," allows the model to recognize and segment similar structures across different images using a single annotation.
In rigorous testing involving 15 diverse medical datasets, including scans of abdominal organs, optic nerves, brain tumors, and skin lesions, MedSAM-2 outperformed previously existing models across the board, setting novel benchmarks in medical image analysis. Notably, it achieved an impressive 88.6% Dice score in segmenting abdominal organs in 3D images, surpassing the previous leading model by 0.7 percentage points.
The developers have made MedSAM-2 available on GitHub, fostering further development and integration into clinical practices, with the primary goal of advancing medical image analysis and enhancing patient care through cutting-edge AI technology.
Key Takeaways
- MedSAM-2 enhances the segmentation of medical images, offering advanced features such as the "Confidence Memory Bank" and "one-prompt segmentation."
- It outperforms previous models across various medical datasets, achieving a high Dice score in segmenting abdominal organs and other tissues.
- The availability of the model and its code on GitHub encourages continued development and clinical integration.
Analysis
The introduction of MedSAM-2 by Oxford researchers has the potential to revolutionize medical imaging workflows, significantly reducing manual annotation requirements and enhancing diagnostic accuracy. Short-term effects may include increased efficiency for radiologists and possible cost savings in healthcare, while long-term implications could include standardized image analysis, thus benefiting medical education and global health initiatives. This advancement may also create added pressure for competitors in AI healthcare, such as Meta, to innovate. Additionally, the financial market might respond favorably to this development, potentially leading to increased investments in AI healthcare solutions.
Did You Know?
- MedSAM-2:
- Explanation: MedSAM-2, developed by researchers at Oxford, is an advanced AI model designed to enhance the segmentation of 2D and 3D medical images. It treats medical images as video sequences, allowing it to process entire series with only one manual annotation, presenting significant time and effort savings for medical professionals.
- Confidence Memory Bank:
- Explanation: This unique feature stores the most reliable predictions made by the model, enabling it to refer back to these high-confidence predictions when analyzing new images, thereby improving the accuracy and consistency of its segmentation outcomes and maintaining high standards of reliability in medical image analysis.
- One-prompt segmentation:
- Explanation: This feature enables the model to recognize and segment similar structures across different images using a single annotation. Once a structure is manually annotated in one image, the model can automatically identify and segment the same structure in other images without additional annotations, streamlining the image analysis process and enhancing efficiency in medical diagnostics.