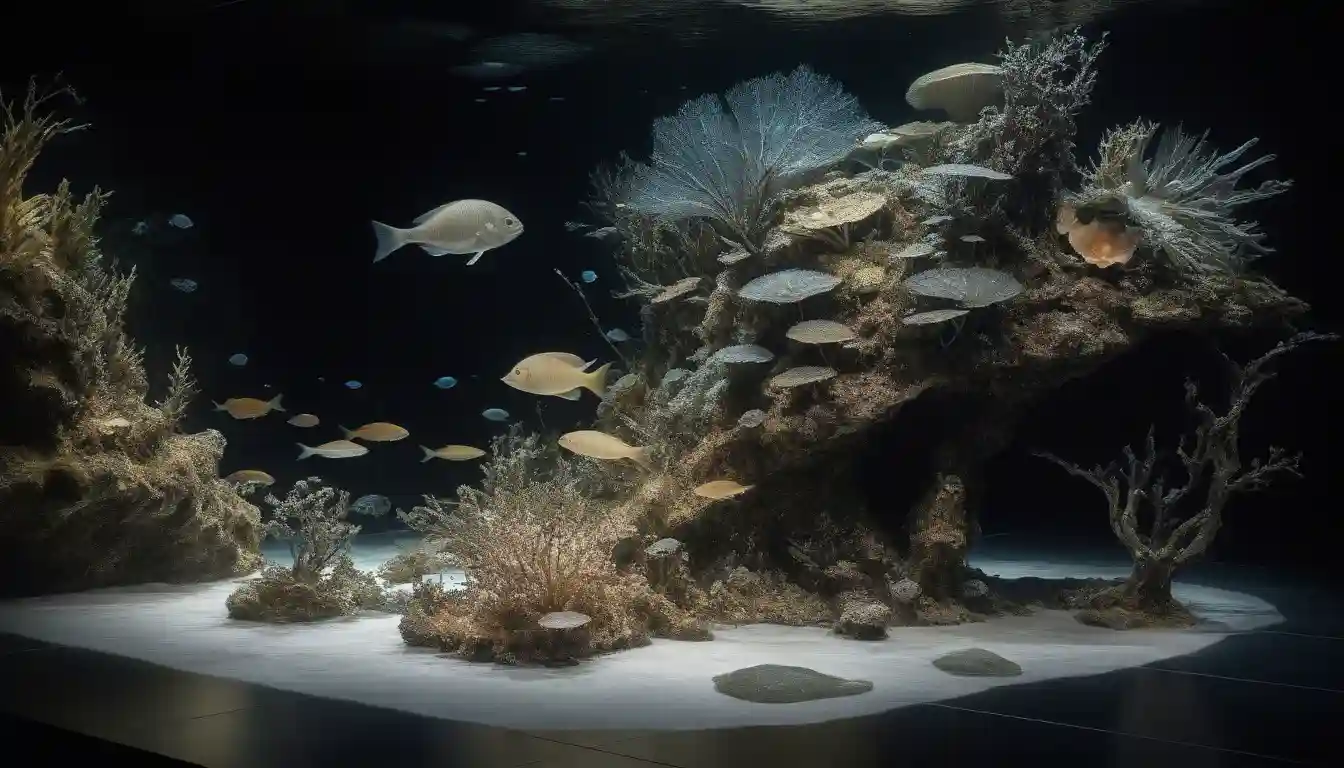
Revolutionizing AI: Cambrian-1 Unveils Vision-Centric Multimodal Language Models for Real-World Mastery
Cambrian-1: Pioneering a Vision-Centric Approach to Multimodal LLMs
Researchers at New York University have introduced Cambrian-1, a groundbreaking family of multimodal large language models (MLLMs) that prioritize vision-centric approaches. Led by Shengbang Tong, Ellis Brown, Penghao Wu, and a team of experts, this project addresses the existing gap between language models and visual representation learning. The release, which took place on June 24, 2024, encompasses model weights, open-source code, datasets, and comprehensive recipes for model training and evaluation. Cambrian-1 aims to enhance the capabilities of MLLMs in real-world scenarios by focusing on sensory grounding through advanced visual representation techniques.
Key Takeaways
- Vision-Centric Design: Cambrian-1 prioritizes vision components in MLLMs, bridging the gap between language models and visual representation learning.
- Comprehensive Benchmarking: Introduction of CV-Bench, a new vision-centric benchmark that evaluates the 2D and 3D understanding of MLLMs.
- Advanced Connector: The Spatial Vision Aggregator (SVA) dynamically integrates high-resolution vision features with LLMs, enhancing visual grounding while reducing the number of tokens.
- High-Quality Data Curation: Emphasis on balancing and curating high-quality visual instruction-tuning data from publicly available sources.
Analysis
Cambrian-1 represents a significant shift in the design and evaluation of multimodal LLMs by focusing on vision-centric approaches. Traditionally, the integration of vision and language models has been hindered by a lack of comprehensive studies on visual representation learning. Cambrian-1 tackles this issue by evaluating over 20 vision encoders through various experimental setups, including self-supervised, strongly supervised, and hybrid models.
The introduction of CV-Bench addresses the limitations of existing benchmarks by transforming traditional vision tasks into visual question answering (VQA) formats. This approach provides a robust evaluation protocol for MLLMs, ensuring that models are tested on diverse perception challenges found in real-world scenarios.
Moreover, the Spatial Vision Aggregator (SVA) enhances the integration of vision features with LLMs. By maintaining high-resolution visual information and reducing the token count, SVA ensures that models retain crucial visual details, thereby improving their performance on tasks requiring strong visual grounding.
To support these advancements, Cambrian-1 includes a well-curated dataset, Cambrian-10M, which balances data sources and adjusts distribution ratios. This curated dataset plays a pivotal role in instruction tuning, enabling models to perform better across various tasks by alleviating issues such as the "answer machine phenomenon," where models provide overly concise responses.
Did You Know?
- The Cambrian explosion, which inspired the name Cambrian-1, was a period approximately 541 million years ago when most major animal phyla appeared. It highlights the importance of vision in evolutionary progress, similar to how Cambrian-1 emphasizes vision for advancing MLLMs.
- The project provides open-source resources, including model weights and detailed training recipes, on platforms like GitHub and Hugging Face, fostering a collaborative research environment.
- The Spatial Vision Aggregator (SVA) not only reduces the number of tokens but also maintains spatial structure, allowing models to better understand complex visual scenes.
Cambrian-1 stands as a milestone in the field of multimodal learning, offering a comprehensive, open approach to improving visual representation in large language models. This initiative not only sets a new standard for MLLM development but also paves the way for future advancements in multimodal systems and visual representation learning.