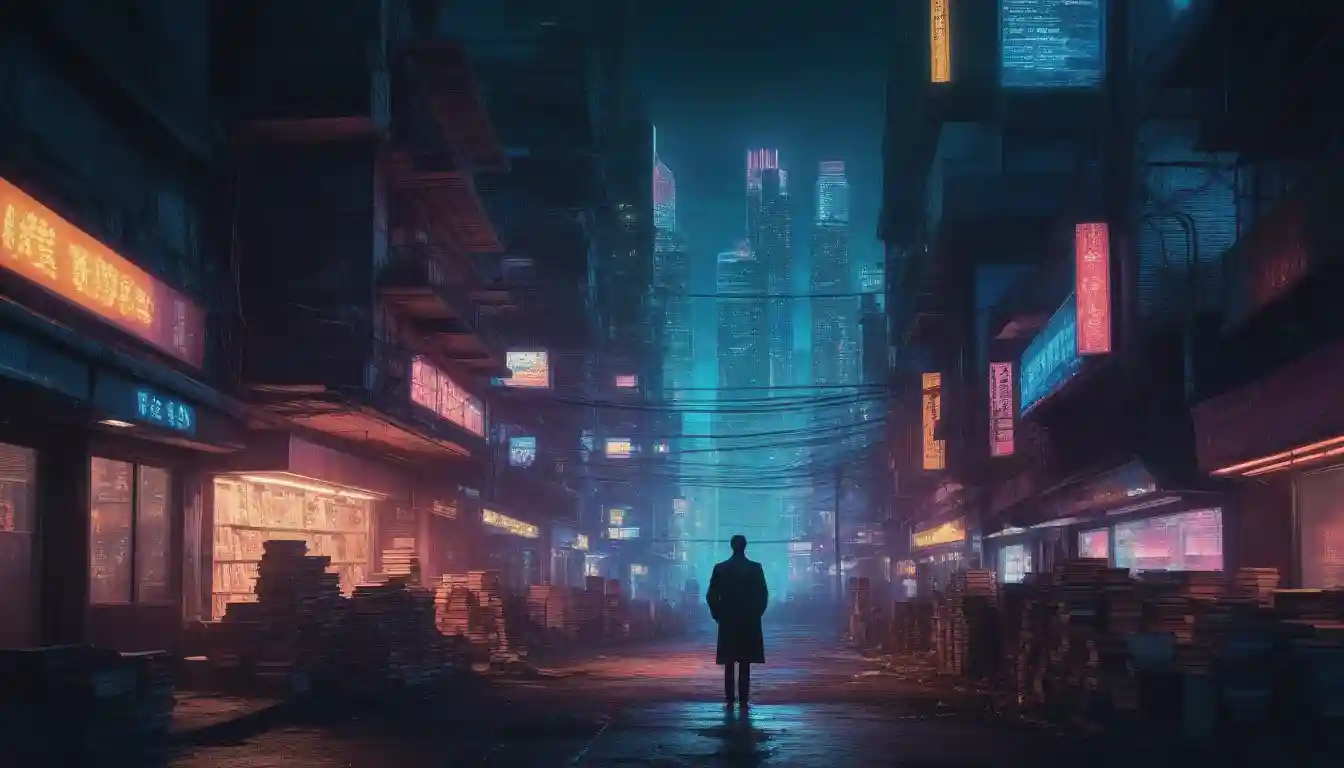
Revolutionizing AI: Long-Context Language Models Poised to Replace RAG Systems in the End
In a significant advancement in artificial intelligence, researchers have explored whether long-context language models (LCLMs) could replace Retrieval-Augmented Generation (RAG) systems. The study, detailed in a recent paper by Jinhyuk Lee and colleagues from Google DeepMind, introduces the Long Context Frontiers (LOFT) benchmark. LOFT aims to assess LCLMs' performance in handling extensive context windows, stretching up to millions of tokens. This exploration could mark a paradigm shift in how AI models retrieve and process information, potentially streamlining complex tasks into more user-friendly, efficient systems.
The research, led by Jinhyuk Lee, Anthony Chen, and Zhuyun Dai at Google DeepMind, introduced the LOFT benchmark to evaluate the capabilities of LCLMs in performing tasks traditionally managed by specialized RAG systems. The benchmark includes a suite of tasks requiring extensive context, up to one million tokens, to test the models' retrieval, reasoning, and generation abilities. The motivation behind this research is to simplify AI pipelines. Traditional RAG systems rely on complex, task-specific tools and pipelines that are prone to errors and require significant expertise to manage. By contrast, LCLMs promise a unified approach that can handle diverse tasks within a single model. These findings, published on arXiv in June 2024, mark a significant step forward in AI and natural language processing, suggesting that long-context windows are promising and might be the real future in this field.
Key Takeaways
-
LCLMs Show Promise: Initial results from the LOFT benchmark indicate that LCLMs can rival state-of-the-art RAG systems in several tasks, including text retrieval and retrieval-augmented generation, despite not being specifically trained for these tasks.
-
Scalability: LOFT supports context lengths up to one million tokens, with potential to scale further. This scalability is crucial for real-world applications where context can span millions of tokens.
-
Simplified Pipelines: By integrating retrieval and reasoning capabilities into a single model, LCLMs can eliminate the need for specialized retrievers and databases, potentially reducing errors and improving efficiency.
-
Room for Improvement: Despite their potential, LCLMs still face challenges, particularly in tasks requiring compositional reasoning, such as SQL-like operations. This highlights the need for ongoing research to enhance their capabilities.
Analysis
The introduction of LOFT is a groundbreaking step in evaluating the limits and potentials of LCLMs. The benchmark encompasses six main areas:
-
Text Retrieval: LCLMs can directly ingest and retrieve information from large corpora, reducing the need for separate retrieval systems. In tests, models like Gemini 1.5 Pro performed comparably to specialized systems like Gecko.
-
Retrieval-Augmented Generation (RAG): By reasoning over large corpora directly, LCLMs simplify RAG pipelines, addressing issues like query decomposition and cascading errors.
-
SQL-Like Reasoning: LCLMs show potential in processing entire databases as text, enabling natural language querying without converting to formal query languages. However, performance still lags behind specialized SQL systems.
-
Many-Shot In-Context Learning (ICL): LCLMs can handle more examples in context compared to traditional few-shot setups, potentially improving learning and adaptation to new tasks.
The performance of LCLMs in these tasks demonstrates their ability to handle complex, long-context tasks with a streamlined approach, reducing the need for multiple, specialized systems. However, challenges remain, particularly in ensuring consistent performance across extremely large contexts and enhancing their compositional reasoning abilities.
Did You Know?
-
Gemini 1.5 Pro vs. Specialized Models: In the LOFT benchmark, Gemini 1.5 Pro outperformed GPT-4o and other specialized models in various retrieval tasks, showcasing the evolving capabilities of LCLMs in handling multimodal data including text, images, and audio.
-
Cost Considerations: Evaluating LCLMs over the extensive datasets in LOFT can be expensive. For instance, running the 128k token test set across all datasets costs approximately $1,568 for Gemini 1.5 Pro, highlighting the significant computational resources required for such advanced models.
-
Efficiency Enhancements: One of the key advantages of LCLMs is their compatibility with prefix-caching techniques, which can significantly reduce the computational overhead by encoding the corpus only once, despite the large context size.
The ongoing advancements in LCLMs and their evaluation through benchmarks like LOFT are paving the way for more robust, scalable, and efficient AI systems that can handle unprecedented volumes of contextual information. As research continues, the potential for LCLMs to fully replace traditional RAG systems becomes increasingly plausible, heralding a new era in AI-driven information retrieval and processing.