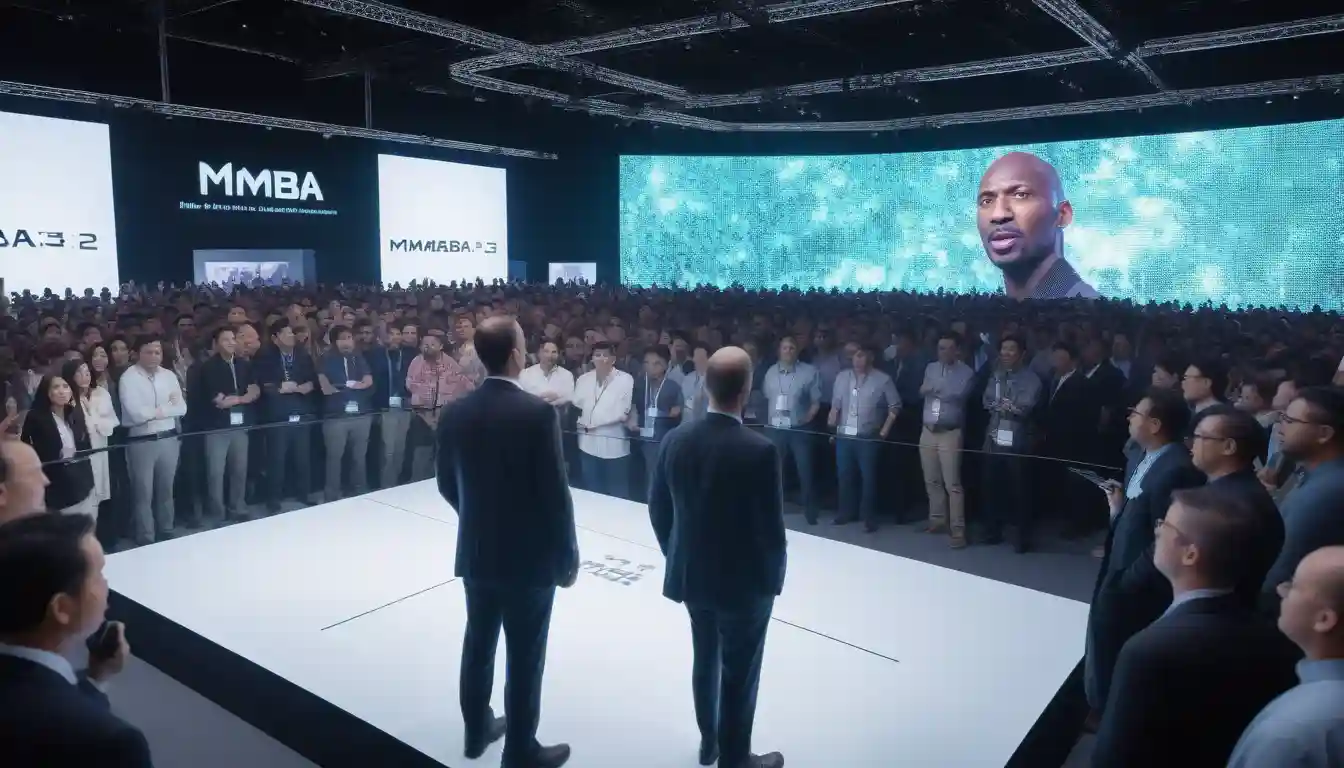
Revolutionizing AI: Mamba2 Unveils Next-Gen Architecture for Faster, Smarter Language Modeling
Revolutionizing AI: Mamba2 Unveils Next-Gen Architecture for Faster, Smarter Language Modeling
The new Mamba2 architecture marks a significant advancement in the realm of machine learning, specifically in the application of state-space models (SSMs) for language modeling. Developed as an enhancement of the original Mamba architecture, Mamba2 promises increased efficiency and improved performance, rivaling and even surpassing the well-established Transformer models in certain scenarios. This leap in capabilities is rooted in theoretical connections between SSMs and various attention mechanisms, optimized through advanced matrix operations.
Key Takeaways
- Enhanced Efficiency and Speed: Mamba2 introduces optimizations that deliver a performance speed-up of 2-8 times over its predecessor, particularly through its state space duality (SSD) framework which enhances matrix operations crucial for deep learning tasks.
- Competitive Accuracy: Across multiple standard benchmarks like LAMBADA and PIQA, Mamba2 has shown to either match or outperform traditional Transformer models and its predecessor, especially in complex language modeling tasks that involve memory and associative recall.
- Scalability: Mamba2 scales efficiently with model size, maintaining or improving performance metrics such as perplexity and accuracy as it grows, thus providing robustness across varying scales from 125M to 2.8B parameters.
- Hybrid Model Potentials: The architecture also experiments with hybrid models combining SSM layers with attention and MLP layers, finding that a mix can sometimes yield better results than single-method models.
Deep Analysis
The Mamba2 architecture stands out due to its innovative combination of SSMs and attention mechanisms, a union that is theoretically grounded in the study of structured semiseparable matrices. This blend not only improves computational efficiency but also enhances the model's ability to handle large-scale language tasks. The model's architecture allows for extensive scalability and adaptability, adjusting to different model sizes and tasks with minimal loss in performance. This is particularly evident in its superior handling of associative recall tasks, where it significantly outperforms earlier models.
One notable aspect is the integration of SSD, which leverages matrix multiplication optimizations on modern hardware (like GPUs), significantly reducing the wall-clock time for training and inference. The results from zero-shot evaluations across various tasks confirm that Mamba2 not only speeds up processing but does so without compromising, and sometimes improving, the accuracy and performance of language modeling tasks.
Did You Know?
- State Space Models and Transformers: While SSMs like Mamba2 are relatively new in the spotlight of AI architectures, they share a close relationship with the widely-used Transformer models. This relationship is centered around their handling of sequences and structured data, where both aim to optimize how information is processed over time.
- Beyond Language Models: The principles used in Mamba2's development are not just applicable to language tasks. The underlying architectural improvements have potential applications in other areas of artificial intelligence, such as pattern recognition, autonomous systems, and predictive analytics, where efficiency in handling large data sets at high speeds is crucial.
- Future of Hybrid Models: The exploration into hybrid models combining SSD, MLP, and attention layers is setting the stage for future research, where the integration of different architectural approaches could lead to even more powerful AI systems. This approach reflects a growing trend in AI research that seeks to blend the best features of various model types to optimize both performance and resource usage.