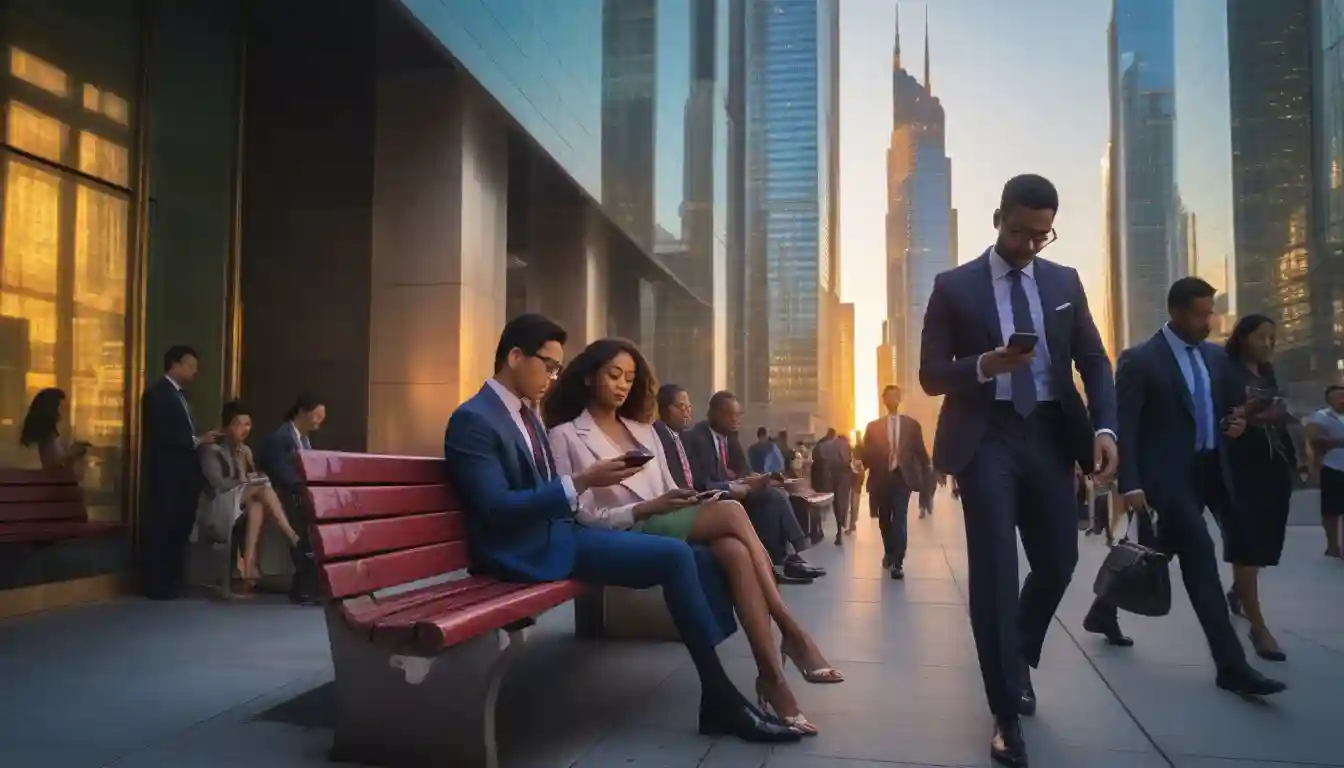
On-Device LLMs: The Next Big Wave in AI
The world of artificial intelligence (AI) is on the brink of a revolution, with on-device large language models (LLMs) poised to transform user experiences and technology landscapes. This emerging trend involves deploying LLMs directly on personal devices like smartphones, tablets, and computers, marking a significant shift from cloud-based AI services. Companies like Nvidia, Apple, and Qualcomm are at the forefront, driven by advancements in hardware and optimization techniques.
On-device LLMs are gaining momentum due to several compelling advantages. Unlike traditional cloud-based models, these LLMs process data locally, ensuring that sensitive information remains on the user's device. This development is particularly critical for applications that handle personal data, such as healthcare and financial services. The push for local deployment is also fueled by the need for reduced latency, cost efficiency, and offline capabilities. Industry leaders like Nvidia, with its "Chat with RTX," and Apple, through its research on running LLMs in flash memory, are exploring ways to bring powerful AI capabilities to users' devices. Qualcomm's efforts in developing sub-10 billion parameter models optimized for mobile and edge devices further highlight the industry's commitment to this trend.
Key Takeaways
-
Enhanced Privacy and Security: On-device LLMs keep sensitive data within the device, significantly reducing the risk of data breaches and unauthorized access. This is especially crucial for sensitive applications in healthcare, finance, and personal assistance.
-
Reduced Latency and Improved User Experience: By eliminating the need for data to travel to and from the cloud, on-device LLMs offer instantaneous responses. This is vital for real-time applications such as voice assistants, real-time translation, augmented reality (AR), and virtual reality (VR) experiences.
-
Cost Efficiency: Local processing allows users and companies to avoid the recurring costs associated with cloud-based services, making advanced AI technologies more accessible and affordable. This is particularly beneficial for small businesses and individual users.
-
Offline Capabilities: On-device LLMs enable applications to function without an internet connection, which is essential for use in remote areas, during travel, or in situations with unreliable connectivity.
Analysis
The move towards on-device LLMs is supported by a combination of optimization techniques and hardware advancements. Techniques such as quantization, pruning, distillation, and sparse modeling help reduce the size and improve the efficiency of these models without compromising performance. For instance, larger models like GPT-4 have been fine-tuned to create smaller versions like GPT-4o, which are optimized for on-device use.
Hardware improvements, including AI accelerators and specialized chips like TPUs (Tensor Processing Units) and NPUs (Neural Processing Units), allow sophisticated AI computation in compact and energy-efficient forms. This is crucial for mobile devices, wearables, and other battery-powered gadgets. Efficient architectures, such as transformers with efficient attention mechanisms, are also being designed to cater specifically to edge devices, enhancing real-time decision-making capabilities.
On-device LLMs are also making significant inroads into various applications. For example, in autonomous vehicles, they enable real-time data analysis from sensors, which is critical for navigation and safety. In healthcare, on-device models can handle sensitive patient data without the need for cloud storage, ensuring confidentiality and quick feedback in diagnostic tools or monitoring devices.
Did You Know?
-
On-device LLMs are revolutionizing smart home devices by keeping interactions and sensitive data secure within the device, reducing exposure to potential breaches. They also lower operational costs by minimizing the need for constant cloud connectivity.
-
In gaming, on-device AI can provide real-time interactions and adaptive gameplay, enhancing the overall user experience. This capability also extends to educational apps, where on-device models can personalize content and responses based on individual learning styles and progress, even in offline settings.
-
The trend towards smaller, more efficient LLMs is democratizing AI, making advanced language technologies more accessible and practical for a wide range of everyday applications. This shift not only enhances user experience but also broadens the scope of AI's applicability across various industries, from security and surveillance to wearable technology and beyond.
As state-of-the-art LLMs continue to shrink in size while maintaining or even improving their capabilities, the potential for integrating AI into everyday life is immense. This transformation is set to redefine how users interact with technology, making AI-powered experiences more personalized, efficient, and secure.