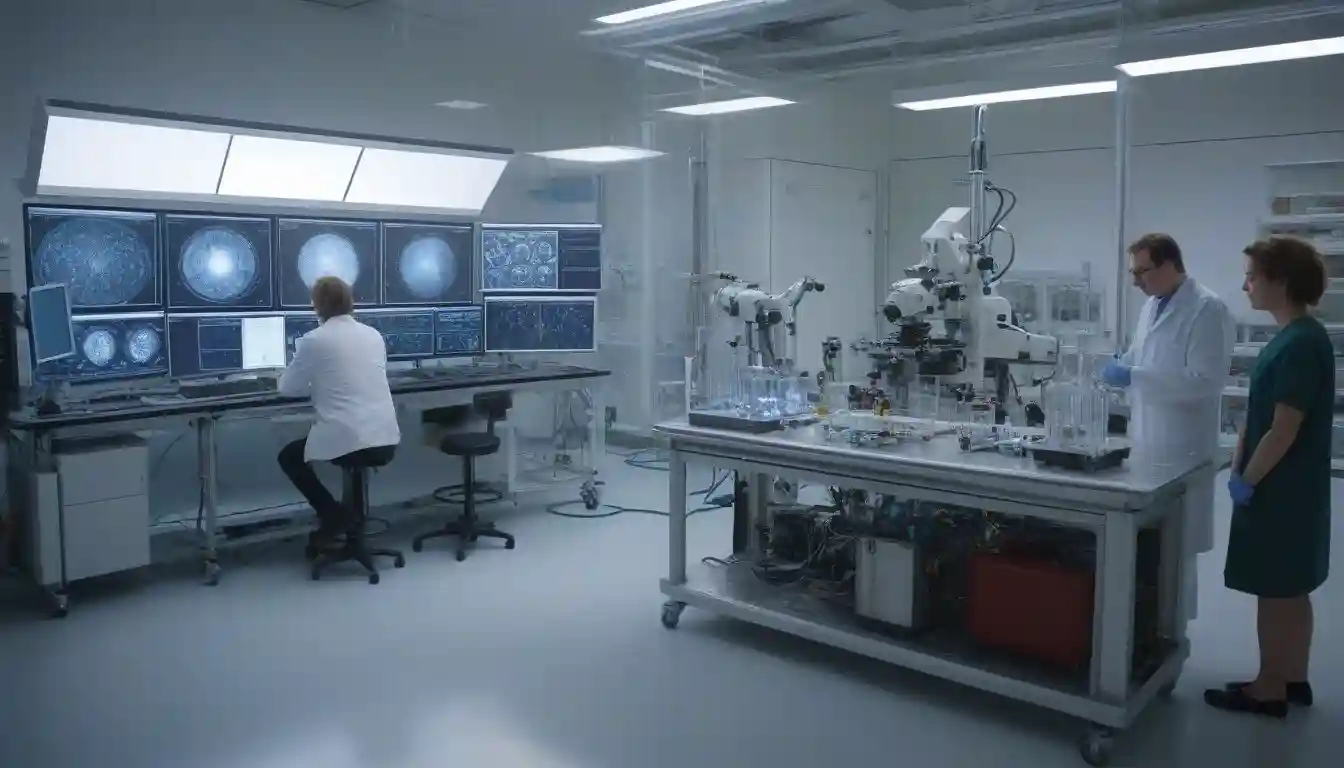
Revolutionizing Cancer Diagnosis: Prov-GigaPath AI Model Unveiled
AI-Powered Model, Prov-GigaPath, Aims to Revolutionize Cancer Diagnosis
A groundbreaking AI model, Prov-GigaPath, developed by a consortium including Microsoft, Providence Health System, and the University of Washington, has the potential to transform cancer diagnosis. The model, featured in a Nature study, draws on an analysis of over a billion tissue sample images from 30,000 patients and has already been integrated into clinical settings. Proven-GigaPath is designed to uncover previously unnoticed insights within pathology slides, surpassing human capabilities. Its formulation involved the most extensive pre-training effort seen to date employing whole-slide modeling. The GigaPath platform, which underpins this innovative approach, dissects high-resolution digital images, rendering them into manageable segments and hones the model by linking image data with actual pathology reports. Encouragingly, Prov-GigaPath has demonstrated superior performance, surpassing other models in numerous cancer subtyping and analytical tasks. Yet, there remains a wealth of unexplored avenues, particularly in precision health exercises, such as mapping tumor microenvironments and projecting treatment responses.
Key Takeaways
- A collaboration between Microsoft, Providence Health System, and the University of Washington resulted in the development of an AI model, Prov-GigaPath, aimed at cancer diagnosis, utilizing 1.3 billion pathology image tiles from 30,000 patients.
- This open-access model is being deployed in clinical applications, showcasing better results in 18 out of 26 assessments compared to alternative models.
- Prov-GigaPath is the culmination of the most extensive pre-training endeavor to date leveraging whole-slide modeling, with data volume five to ten times larger than other datasets.
- Microsoft's GigaPath platform, incorporating AI strategies, divides large-scale images into manageable tiles and identifies patterns related to diverse cancer subtypes.
- The fine-tuning of the model involved associating image data with processed pathology reports using OpenAI's GPT-3.5 generative-AI platform.
Analysis
The unveiling of Prov-GigaPath, an AI model for cancer diagnosis, by Microsoft, Providence Health System, and the University of Washington, harnessing 1.3 billion pathology image tiles, could significantly enhance diagnostic precision. Its application in clinical settings may offer substantial advantages, especially in regions with limited pathology expertise and resources. However, contentious ethical issues may emerge concerning data privacy and the usage of OpenAI's GPT-3.5 for pathology reports processing. In the long run, Prov-GigaPath could contribute to reducing healthcare expenses and enhancing access to first-rate cancer diagnoses. Its impact might indeed reach AI developers, leading to a heightened demand for broader and more diverse medical datasets and advanced AI utilities, thereby necessitating a reevaluation of regulations governing data usage and AI applications in healthcare.
Did You Know?
- Whole-slide modeling: This state-of-the-art AI method, integral to Prov-GigaPath's development, involves scrutinizing high-resolution digital images, such as pathology slides, holistically rather than concentrating on individual sections. This comprehensive approach facilitates the discovery of insights from pathology slides that may elude human perception. Prov-GigaPath’s whole-slide modeling was underpinned by a pre-training effort employing a dataset five to ten times larger than other datasets, rendering it a potent instrument for cancer diagnosis.
- GigaPath (Microsoft's AI-based platform): GigaPath, a Microsoft AI platform tailored to tackle extensive image analysis tasks, particularly in cancer diagnosis, dissects high-resolution digital images into manageable tiles, facilitating more efficient data analysis by AI models like Prov-GigaPath. GigaPath's AI tactics also involve identifying patterns linked to various cancer subtypes and honing the model by connecting image data with actual pathology reports.
- Fine-tuning with OpenAI's GPT-3.5 generative-AI platform: Fine-tuning entails adjusting a model's parameters to enhance its performance for specific tasks. For Prov-GigaPath, fine-tuning involved connecting image data with processed pathology reports utilizing OpenAI's GPT-3.5 generative-AI platform. This process bolstered Prov-GigaPath's capabilities to scrutinize and interpret pathology slides, ultimately enhancing its performance in cancer subtyping and analytical tasks.