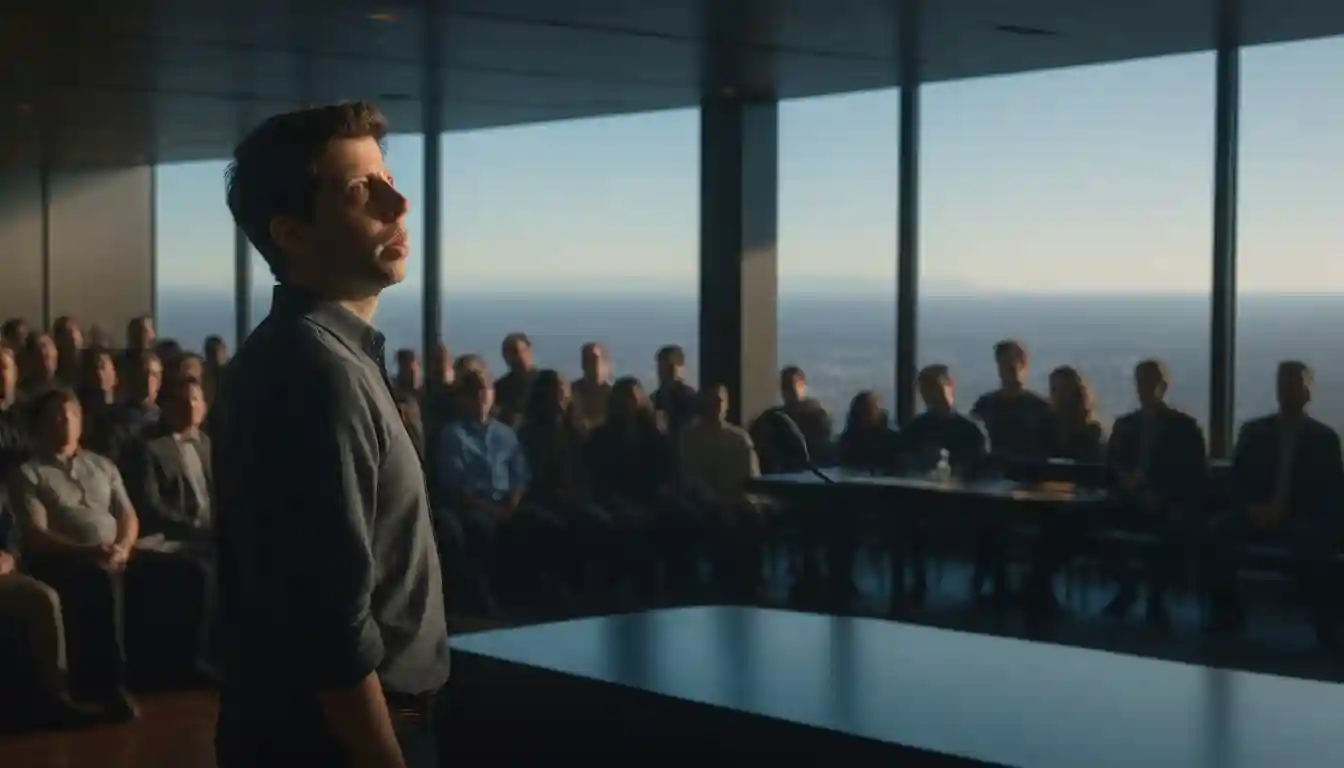
OpenAI Unveils 2025 Common Themes for Improvements, Overlooks Critical Cost Reduction Challenge
As the calendar turns to 2025, OpenAI, the trailblazer in artificial intelligence, finds itself at a pivotal juncture. CEO Sam Altman recently presented a comprehensive plan aimed at elevating the company’s AI capabilities, drawing heavily from extensive user feedback. However, amidst these forward-looking enhancements, a crucial issue remains conspicuously unaddressed: the urgent need for cost reduction.
Sam Altman's Vision for OpenAI’s Future
Today on X, Sam Altman outlined several key areas of improvement that reflect the evolving demands of OpenAI’s diverse user base. Central to these initiatives is the development of more advanced artificial general intelligence (AGI) agents, designed to perform complex tasks with increased autonomy and intelligence. Users have also expressed a desire for improved memory capabilities, enabling the AI to retain and recall information over extended interactions, thereby fostering more coherent and contextually relevant conversations.
Another significant enhancement is the extension of context windows, allowing the AI to manage larger data inputs and sustain longer dialogues without losing track. Additionally, OpenAI is introducing a “grown-up mode,” a specialized setting tailored for professional environments. This mode ensures the AI can handle sensitive and serious topics without triggering content warnings, addressing the needs of professionals such as lawyers dealing with cases like human trafficking.
The roadmap also includes enhanced deep research features, which will provide more accurate and comprehensive data analysis tools for in-depth research. Improvements to Sora, OpenAI’s video processing model, aim to deliver higher quality and more efficient video analysis. Furthermore, the company is prioritizing greater personalization, allowing users to customize their AI interactions to better fit individual preferences and needs.
Community Insights Shape Strategic Directions
The feedback from OpenAI’s user community has been instrumental in shaping these enhancements. Industry experts like Luke Hornof emphasized that innovation often progresses in layers, with some advancements meeting user expectations while more transformative breakthroughs emerge unexpectedly. Jeff Weinstein highlighted the practical necessity of “grown-up mode” in professional settings, underscoring its importance for handling sensitive legal cases without triggering inappropriate content warnings.
Kyrelle Buckley advocated for an “auto mode,” where the AI automatically selects the most appropriate model for each task, simplifying the user experience by eliminating the need for manual model selection. Ritesh Lal discussed the competitive landscape, contrasting OpenAI’s strategies with those of competitors and underscoring the importance of data, compute, and algorithms as the three pillars of AI competition. In response to concerns about the affordability of AI, Sam Altman reaffirmed OpenAI’s mission to make “intelligence too cheap to meter,” though this statement has sparked debate regarding its feasibility amidst rising costs.
The Overlooked Crisis: Escalating Operational Costs
While Altman’s vision paints an optimistic future for OpenAI, industry insiders and stakeholders are raising alarms over the company’s financial sustainability. As of December 31, 2024, OpenAI is grappling with significant operational costs, primarily driven by the deployment of advanced AI models like the o3 reasoning model. This model employs “test-time scaling,” which allocates additional computational resources during inference to enhance performance and accuracy. Although this approach boosts the AI’s capabilities, it simultaneously drives operational costs into the thousands of dollars per high-compute task.
Partners such as Microsoft, which integrate OpenAI’s models into their services, are particularly affected by these high expenses. This financial strain raises serious concerns about the long-term sustainability and profitability of OpenAI’s AI solutions. Despite achieving an impressive annual recurring revenue (ARR) of $4 billion by September 2024 and projecting revenues of $11.6 billion for 2025, the company faces anticipated losses of $5 billion in 2024, escalating to $14 billion by 2026. These figures starkly highlight the immense costs associated with developing and deploying cutting-edge AI technologies.
Global Competition Intensifies with DeepSeek-V3’s Cost Efficiency
Amid OpenAI’s financial struggles, competitors are capitalizing on opportunities to outpace the AI giant through cost-effective innovations. China’s DeepSeek has introduced the DeepSeek-V3 model, which exemplifies significant advancements in both training and inference cost efficiencies. Utilizing a Mixture-of-Experts (MoE) architecture with 671 billion parameters, of which only 37 billion are activated per token, DeepSeek-V3 enhances efficiency by selectively engaging relevant parameters during processing. This allows the model to train on 14.8 trillion tokens over two months at a cost of approximately $5.5 million, a stark contrast to OpenAI’s GPT-4, which has reported training costs exceeding $100 million.
During inference, DeepSeek-V3’s MoE architecture activates only the necessary subset of parameters, significantly reducing computational load and operational costs. The model achieves a token generation speed of 90 tokens per second, making it highly suitable for real-time applications. This level of cost efficiency not only challenges OpenAI’s market dominance but also sets new standards in the AI landscape, emphasizing the critical importance of strategic architectural choices and efficient training protocols.
An Urgent Call for Cost Control
Industry experts and stakeholders are now urging OpenAI to prioritize cost reduction as a top strategic objective for 2025. While the announced feature enhancements like “grown-up mode” and improved memory capabilities address user needs and competitive differentiation, they do little to mitigate the financial pressures threatening the company’s viability. The escalating operational costs associated with advanced models like o3 are hindering accessibility and affordability, particularly in global markets where price sensitivity is high.
Moreover, the mismatch between revenue growth and escalating expenses underscores the necessity for immediate cost efficiencies. With competitors like DeepSeek achieving state-of-the-art performance at significantly lower costs, OpenAI risks losing its competitive edge if it fails to address this fundamental issue. Aligning with OpenAI’s mission of making “intelligence too cheap to meter” requires breakthroughs in cost reduction to remain attainable as models grow more complex and resource-intensive.
Strategies for Achieving Cost Efficiency
To navigate these financial challenges, OpenAI must explore several strategic avenues aimed at reducing costs without compromising innovation. Architectural innovations, such as implementing sparse models and utilizing model distillation and compression techniques, can create smaller, more cost-effective models. Adopting dynamic inference methods to allocate compute resources based on task complexity further enhances efficiency.
Hardware optimization is another critical area, with OpenAI needing to partner with hardware manufacturers to develop AI-specific chips and invest in energy-efficient data centers. Exploring emerging technologies like quantum computing could also provide long-term cost benefits. Additionally, research into training efficiency involves developing more efficient algorithms for backpropagation and gradient optimization, as well as utilizing distributed training approaches to reduce redundancy and improve speed.
OpenAI is also negotiating more favorable terms with cloud providers or developing proprietary infrastructure to lower reliance on external platforms. Collaborating with governments or organizations to subsidize compute costs for socially beneficial applications could further alleviate financial pressures. Furthermore, model lifecycle management, such as extending the lifecycle of existing models through continual refinement and offering tiered model versions based on performance versus cost trade-offs, allows OpenAI to target diverse user segments more effectively.
Balancing Innovation with Fiscal Responsibility
Focusing on cost reduction does not mean abandoning feature enhancements. Instead, OpenAI can integrate cost-efficient strategies into its development processes. For instance, personalization features can leverage dynamic inference, and longer context windows can be optimized through sparse attention mechanisms. By making cost reduction a cornerstone of its 2025 strategy, OpenAI can maintain its leadership in both AI performance and accessibility, ensuring that its advancements remain cutting-edge and universally beneficial.
As OpenAI navigates these challenges, the coming year will be pivotal in determining whether it can harmonize innovation with financial sustainability. Successfully balancing these elements will secure its position as a leader in the rapidly evolving artificial intelligence sector, ensuring that advancements in AI remain both groundbreaking and accessible to a global audience.