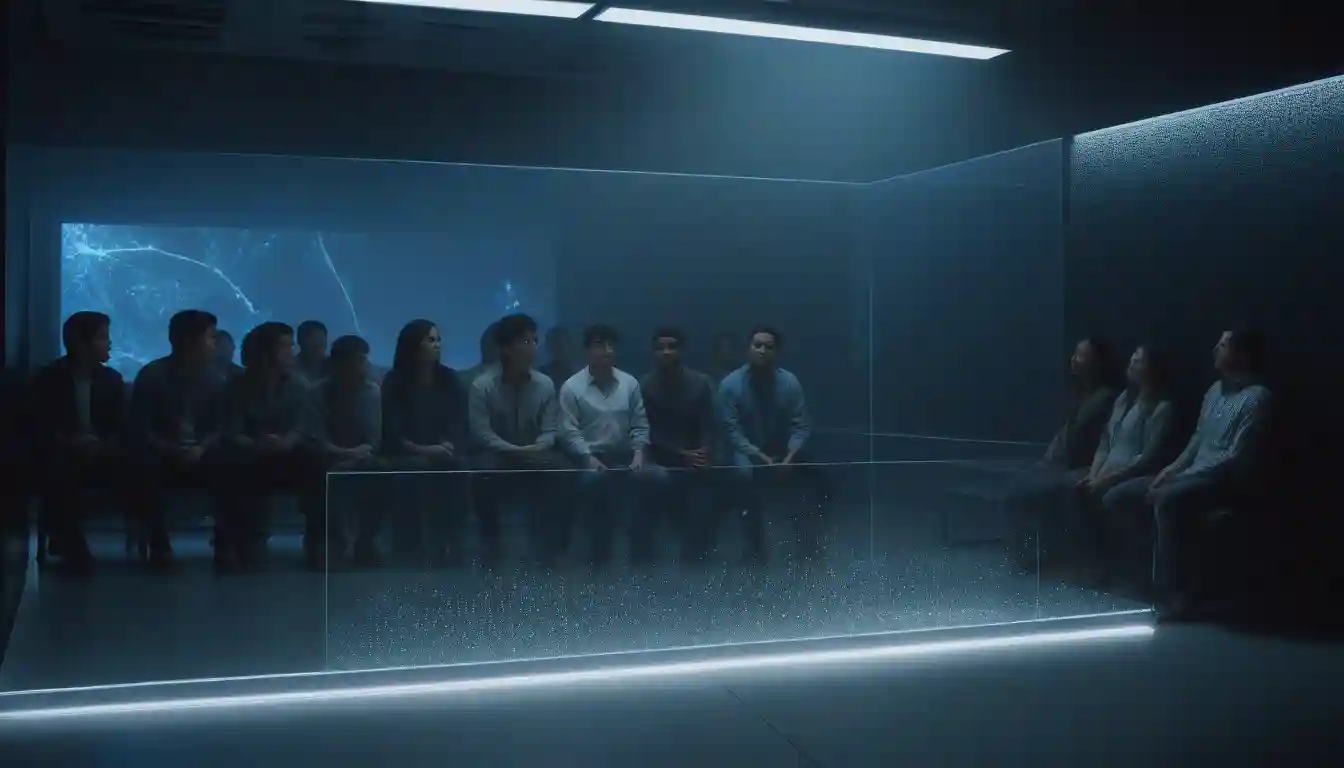
Sierra’s TAU-bench Unveils Challenge for Conversational AI Agents
Sierra, a startup co-founded by OpenAI board member Bret Taylor and Google AR/VR veteran Clay Bavor, has launched TAU-bench, a new benchmark designed to assess the performance of conversational AI agents. The benchmark evaluates the ability of AI agents to handle complex tasks requiring multiple exchanges with simulated users, revealing the limitations of current models. This underlines the necessity for more advanced agent architectures and improved evaluation metrics.
Key Takeaways
- Sierra's TAU-bench evaluates AI agents on complex tasks requiring multiple exchanges with simulated users.
- TAU-bench challenges AI agents with diverse, open-ended tasks and realistic tool use.
- The benchmark objectively evaluates task completion, not conversation quality, for reliability assessment.
- TAU-bench’s modular design allows easy addition of new domains, rules, and evaluation metrics.
- Current LLMs struggle with TAU-bench, highlighting the need for more advanced models and fine-grained evaluation metrics.
Analysis
The introduction of TAU-bench by Sierra unveils the limitations of current AI agents in handling complex, multi-exchange tasks, emphasizing the necessity for advanced architectures. It impacts AI developers and tech giants like OpenAI and Google, compelling them to enhance their models' reasoning and planning capabilities. The short-term consequence is a push for more sophisticated LLMs, while long-term implications include potential improvements in AI reliability and effectiveness in real-world applications. TAU-bench's modular design facilitates ongoing refinement, suggesting a future where AI benchmarks evolve in tandem with technological advancements.
Did You Know?
- TAU-bench: A new benchmark developed by Sierra to evaluate conversational AI agents on their ability to handle complex, multi-exchange tasks with simulated users. It focuses on the agents' final outcomes, using realistic dialog scenarios and tool use, and is designed to be modular for easy updates and additions.
- ReAct: A term referring to a method used by AI agents where they react to stimuli or prompts in a conversation. In the context of TAU-bench, it was observed that agents using simple constructs like ReAct struggled with basic tasks, indicating a need for more sophisticated agent architectures.
- Large Language Models (LLMs): Advanced AI models designed to understand and generate human-like text based on the data they are trained on. The initial tests with TAU-bench on LLMs from OpenAI, Google, and others showed significant challenges in task completion and reliability, suggesting a need for more advanced models with enhanced reasoning and planning capabilities.