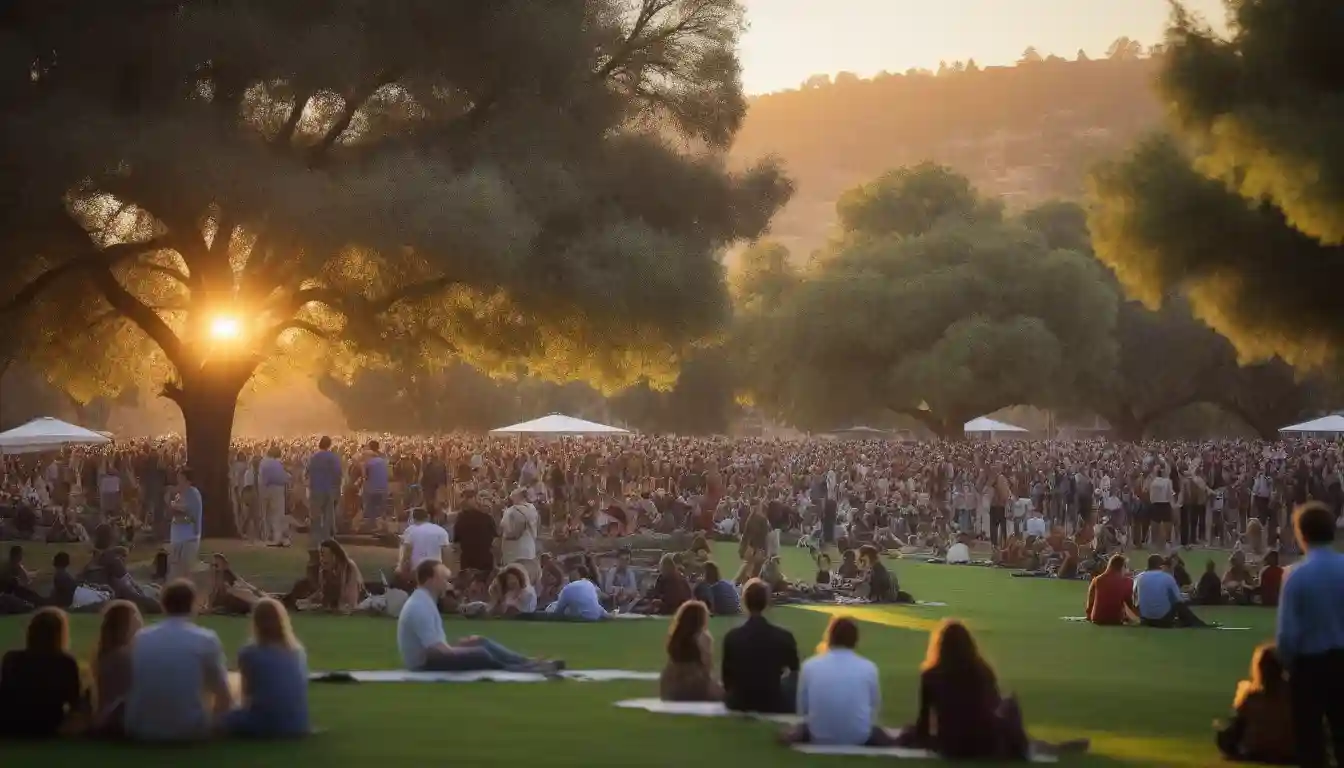
Stanford University researchers conducted a study on the effectiveness of Retrieval Augmented Generation (RAG) in improving the accuracy of Large Language Models (LLMs). The study revealed that the reliability of RAG systems depends on both the quality of the data sources and the prior knowledge of the language model. When tested, the models showed a significant increase in accuracy when provided with correct reference information through RAG. However, the study highlighted the importance of the quality and reliability of the reference information, especially in commercial applications such as finance, medicine, and law. It emphasized the need for transparency in how these models handle conflicting or incorrect information, cautioning that RAG systems, like LLMs, can be prone to errors.
Key Takeaways
- Retrieval Augmented Generation (RAG) systems' reliability depends on the quality of data sources and the language model's pre-trained knowledge.
- Tension exists between the internal knowledge of a language model and information provided via RAG, especially when it contradicts the model's pre-trained knowledge.
- Accuracy of language models significantly improves with high-quality reference data, increasing from 34.7% to 94% with RAG systems.
- Well-trained prior knowledge of the model is crucial in recognizing and ignoring unrealistic information, especially in areas like finance, medicine, and law.
- Transparency is crucial for the commercial use of RAG systems, as users and developers need to be aware of potential conflicts or incorrect information.
Analysis
The study by Stanford University reveals that the reliability of Retrieval Augmented Generation (RAG) systems is contingent on data quality and prior knowledge of Large Language Models (LLMs). This finding raises concerns for commercial applications in finance, medicine, and law, where the accuracy and transparency of reference information are crucial. The tension between the model's internal knowledge and RAG-provided information is emphasized, signaling potential implications for organizations relying on language models. Short-term consequences may include increased scrutiny on data sources, while long-term effects could lead to improved transparency standards and better training of language models to handle conflicting information. Stakeholders in technology, finance, and legal sectors may be impacted.
Did You Know?
- Retrieval Augmented Generation (RAG) systems' reliability depends on the quality of data sources and the language model's pre-trained knowledge.
- Tension exists between the internal knowledge of a language model and information provided via RAG, especially when it contradicts the model's pre-trained knowledge.
- Accuracy of language models significantly improves with high-quality reference data, increasing from 34.7% to 94% with RAG systems.