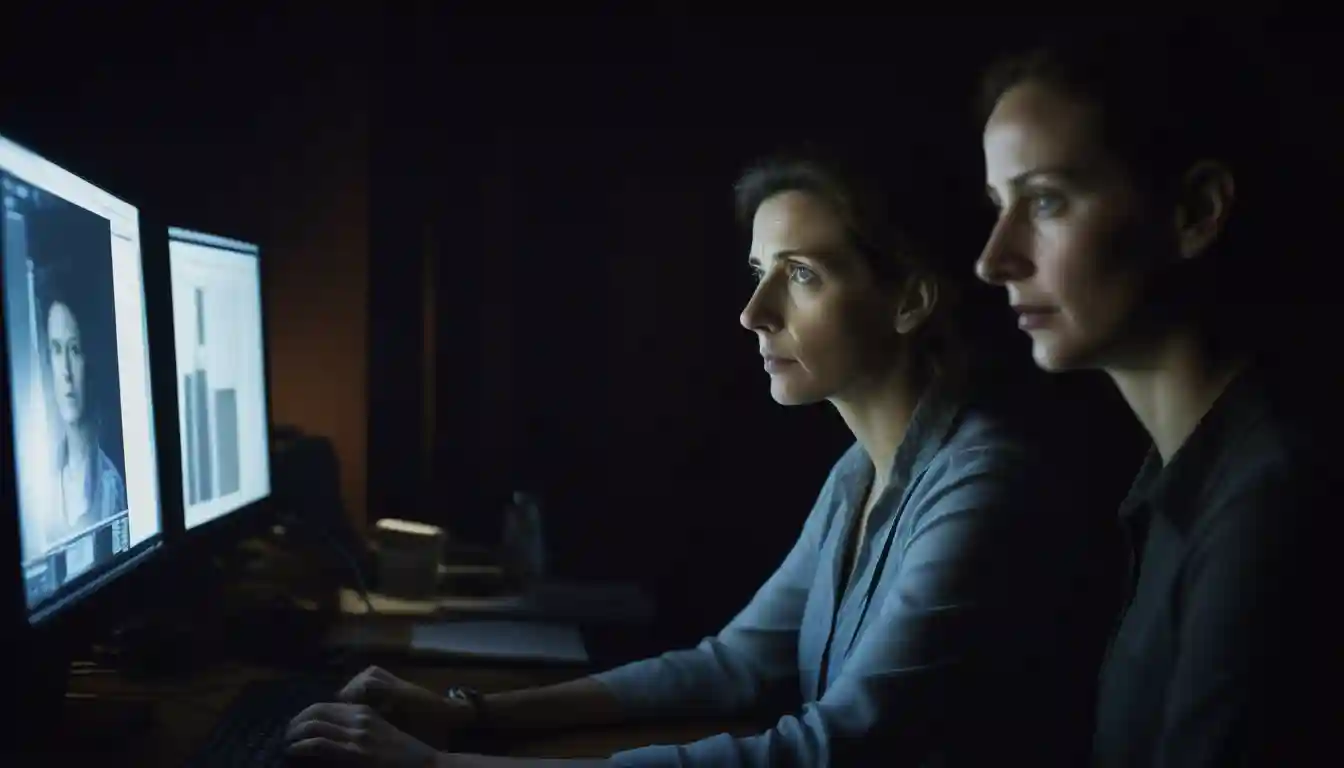
The Potential Pitfalls of AI Safety Tests
As AI technology continues to advance, concerns about its safety and reliability have come to the forefront. Despite ongoing efforts to develop and implement rigorous safety tests, there are growing concerns that our current testing methods may not be sufficient to ensure the safe deployment of AI systems.
The widespread integration of AI technology into various aspects of our lives has sparked conversations about the capabilities and limitations of AI safety tests. While there is a collective desire for AI to be secure and dependable, challenges persist in accurately assessing its safety and reliability.
AI models possess a wide range of functionalities, from generating text and images to composing music. However, these models are not infallible, and their behaviors can be unpredictable. Amid this backdrop, major companies and government entities are grappling with the task of devising novel methods to evaluate and validate the safety of these AI models.
Recently, organizations such as Scale AI and the U.K. AI Safety Institute have spearheaded the development of tools to evaluate the risks associated with AI models. However, a study conducted by the Ada Lovelace Institute has revealed that existing tests may not be sufficiently robust. Experts have highlighted the susceptibility of current tests to manipulation and their inability to accurately reflect real-world AI behavior.
One of the primary shortcomings lies in the fact that several tests primarily assess AI performance in controlled environments, overlooking its behavior in real-world scenarios. Moreover, the issue of "data contamination" looms large, where AI performance in tests may be inflated due to its training on the same dataset as the test data.
Another method known as "red-teaming," which involves individuals uncovering vulnerabilities in AI models, faces challenges stemming from the absence of standardized procedures, making it an expensive and insufficiently regulated process.
In response to these challenges, the Ada Lovelace Institute has advocated for increased involvement from governments and policymakers. They recommend greater public engagement in test development and extended support for third-party evaluations.
Furthermore, there is a pressing need for "context-specific" evaluations that scrutinize the potential impacts of AI models on diverse user groups, along with the identification of potential bypasses for safety measures. However, it is crucial to acknowledge that despite concerted efforts, complete assurance of AI safety may remain elusive, hinging on its application and the end-user.
In essence, the quest for ensuring the safety of AI presents a formidable challenge. Enhanced testing methodologies and widespread participation are imperative in the pursuit of maximizing its safety.
Key Takeaways
- AI safety benchmarks may be inadequate: Current benchmarks may not comprehensively capture real-world AI behaviors, potentially undermining their reliability.
- Data contamination: The reliance on the same dataset for training and testing can lead to inflated performance in AI benchmarks, masking real-world applicability.
- Red-teaming lacks standardized methods: The absence of uniform procedures for red-teaming poses obstacles in assessing its effectiveness in identifying AI vulnerabilities.
- Public-sector involvement is crucial: Governments need to play a more proactive role in enhancing the evaluation of AI safety, necessitating widespread public engagement.
- Context-specific evaluations are essential: Identifying potential impacts on diverse user groups and circumvention of safety measures is critical for comprehensive AI safety assessments.
Analysis
The inadequacies in current AI safety benchmarks, compounded by data contamination and the absence of standardized red-teaming methods, emphasize the urgency of greater public-sector involvement and the need for context-specific evaluations. Organizations and government agencies face critical challenges in ensuring the reliability of AI systems, which could have immediate repercussions in terms of misapplications and public distrust, as well as long-term consequences impacting AI integration and innovation. Strengthening public participation and third-party evaluations is crucial for augmenting both the safety and trustworthiness of AI.
Did You Know?
- AI safety benchmarks may be inadequate: The current benchmarks used for assessing AI safety often fall short of predicting real-world behaviors due to their reliance on controlled environments, leading to potential discrepancies in performance and real-world behavior.
- Data contamination: When an AI model is trained and tested on the same dataset, its performance in benchmarks may not accurately reflect its ability to adapt to new, untested data, undermining its real-world applicability and safety.
- Red-teaming lacks standardized methods: The absence of standardized procedures for red-teaming contributes to inconsistencies in the identification and resolution of AI vulnerabilities, potentially leaving safety gaps unaddressed.