Why SlowFast-LLaVA-1.5 Could Be the Breakthrough for Long-Form Video Understanding—Without the Compute Overhead
The Real Bottleneck in AI Video Processing? Tokens, Not Just Compute
Long-form video content is exploding. From surveillance footage to educational lectures, the need to extract insights from multi-minute—or even multi-hour—videos has never been greater. But for AI, understanding long-range temporal context in video isn’t just a matter of throwing in more frames or fine-tuning a bigger model.
The real challenge? Token efficiency.
Most existing Video Large Language Models (Video LLMs) struggle to scale. They process vast amounts of data but burn through compute and memory, making them impractical for mobile, edge, or even many enterprise deployments.
That’s where SlowFast-LLaVA-1.5 enters the scene. Developed as a token-efficient, reproducible solution for long-form video understanding, it combines a novel two-stream processing approach with a lean training pipeline—delivering state-of-the-art performance even with smaller models (as low as 1B parameters).
For businesses betting on scalable AI, and for investors seeking edge-deployable AI solutions, this model represents a meaningful technical and strategic shift.
The Two-Stream Architecture That Changed the Game
At the core of SlowFast-LLaVA-1.5 is a clever architectural twist borrowed and adapted from prior work in video recognition: a SlowFast mechanism. Here's how it works:
- The Slow stream processes fewer frames at higher resolution, capturing rich spatial details from select keyframes.
- The Fast stream handles many more frames but with less granularity, focusing on motion and temporal flow.
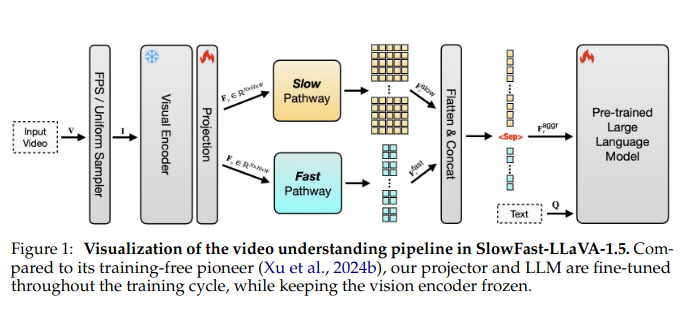
By combining these pathways and aggregating their outputs, the model captures both what’s happening in each frame and how scenes evolve over time—without drowning in tokens.
This is a shift from monolithic video transformers that struggle to balance spatial accuracy and temporal reach. The result? SlowFast-LLaVA-1.5 processes nearly twice as many frames using just 65% of the tokens compared to other leading models.
Leaner Training, Broader Adoption
Training large video models is notoriously messy—often involving proprietary datasets, multi-stage pipelines, and resource-intensive tuning. The authors of SlowFast-LLaVA-1.5 took a more pragmatic route:
- Stage I – Image-Only Fine-Tuning: The model is first trained on publicly available image datasets to establish visual grounding and general reasoning.
- Stage II – Joint Video-Image Training: It’s then exposed to a curated set of video and image data, enabling the model to learn both spatial and temporal dynamics.
The datasets are open-source, and the approach is reproducible—two attributes often missing from recent LLM releases. This emphasis on accessibility isn’t just academic; it lowers the entry barrier for smaller companies or startups looking to integrate advanced video AI.
Benchmarks That Back the Claim
Performance metrics were tested across multiple high-profile benchmarks, including:
- LongVideoBench: 62.5% with the 7B model
- MLVU (Multimodal Long Video Understanding): 71.5% with the same model
Notably, even the 1B and 3B parameter models delivered competitive results, proving that size isn’t always the differentiator. That’s a particularly attractive trait for firms exploring mobile or edge-based deployment.
Additionally, thanks to its joint training strategy, the model didn’t sacrifice image reasoning capabilities. It competes on par with dedicated image-language models—a clear win for unified multimodal processing.
Efficiency Without Compromise
In raw numbers, the efficiency gains are clear:
- Processes up to 2× the number of frames
- Uses ~35% fewer tokens than comparable models
- Reduces compute costs significantly while maintaining or exceeding performance levels
This balance of accuracy and efficiency unlocks a wider range of use cases—from smart cameras and IoT monitoring systems to in-app video assistants or enterprise knowledge mining tools.
For investors, that means stronger ROI and lower barriers to productization.
Strategic Value Across Sectors
Enterprise AI Integration: With its compact size and efficient architecture, SlowFast-LLaVA-1.5 can be deployed in environments where bandwidth, compute, and latency are critical constraints—think on-site surveillance, customer behavior analytics in retail, or in-field machinery diagnostics.
Media & Streaming Platforms: Automated summarization, search indexing, and content moderation for long-form media becomes feasible at lower cost.
Assistive Tech & Accessibility: Real-time interpretation of video for visually impaired users becomes more viable when the processing doesn’t require cloud-scale infrastructure.
Security & Defense: Drones and on-device surveillance systems benefit immensely from smaller models that can analyze live feeds without uplinking gigabytes of video.
Limitations and Open Opportunities
The authors acknowledge two key limitations:
- Frame Sampling Limitations: While frame sampling is FPS-based for most use cases, the model defaults to uniform sampling when video length exceeds a set frame threshold—potentially missing critical moments in ultra-long content.
- Frozen Vision Encoder: To preserve efficiency, the vision encoder remains frozen during training. While effective, fine-tuning this module may unlock higher performance, albeit at greater compute cost.
These constraints point toward logical next steps for future versions: adaptive sampling techniques, selective vision module tuning, and integration of memory-efficient architectures.
Why This Matters for the Industry
The vision-to-language paradigm is evolving fast. Until now, most breakthroughs in video AI have leaned on brute force—bigger models, larger datasets, more tokens.
SlowFast-LLaVA-1.5 offers a strategically leaner approach. It’s not just a new model—it’s a blueprint for how efficient AI can scale video understanding across industries without overloading infrastructure.
It demonstrates that token efficiency can be as powerful as parameter scale—a message both the research community and commercial ecosystem need to take seriously.
Final Takeaway for Investors and Builders
If your roadmap involves intelligent video processing—whether for consumer tech, industrial applications, or real-time analytics—SlowFast-LLaVA-1.5 is a signal that high-performance AI doesn’t have to mean high-cost AI.
For venture capital, this opens the door to supporting startups who previously lacked the resources to train or run massive video models. For enterprise builders, it’s a chance to deploy competitive video AI without rebuilding your entire infrastructure stack.