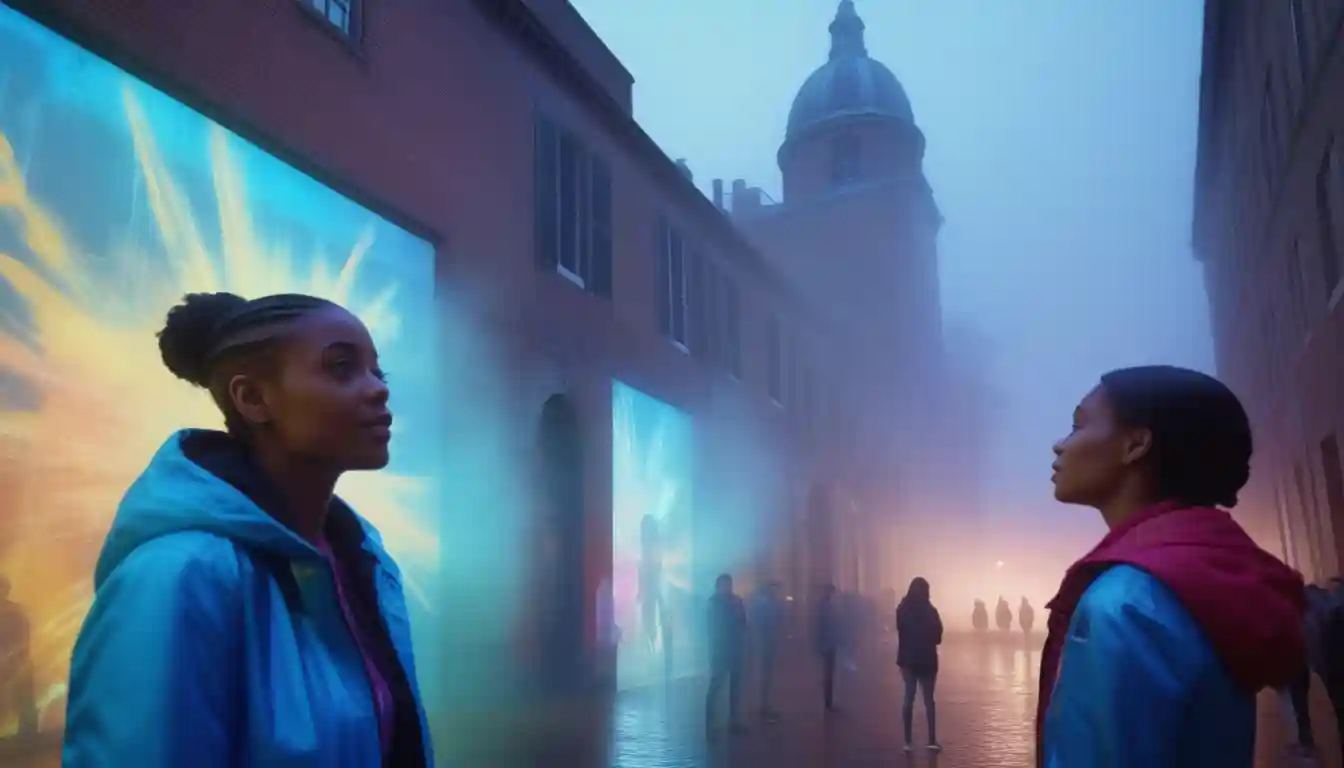
VCs Bet on Young Innovators and Model Breakthroughs Instead of "Engineering Tweaks" as AI Startups Face a Major Shift
New VC Insights for AI Startup Founders: Why Model Innovation and Young Talent Matter More Than Engineering Tweaks
The venture capital landscape for AI startups is undergoing a major shift, with investors prioritizing foundational model breakthroughs and youthful, agile teams over traditional engineering refinements and seasoned tech veterans. According to industry insights, the next two to three years will witness at least two significant technological iterations, driven by advancements in scaling laws, reinforcement learning, and novel AI architectures beyond Transformers.
This shift is already evident in market trends: companies that focus on improving foundational AI models, rather than fine-tuning existing applications, are gaining a competitive edge. The case of Talkie surpassing Character.ai highlights this shift, as Talkie’s continued investment in foundational models outperformed its competitor’s stagnant approach. Additionally, AI startups like DeepSeek, which leverage young, innovative teams, are achieving exceptional results at lower costs, proving that agility and fresh thinking are critical success factors.
For AI startup founders, this insight is game-changing: instead of relying on incremental engineering improvements, the focus should be on pioneering new AI architectures and recruiting young, experimental talent to drive innovation.
Furthermore, the "killer app" of AI has already emerged—ChatGPT-like UI directly on top of LLMs. With the maturity of large language models , most applications, including agents and domain-specific workflows, will become obsolete as LLMs handle these functions natively. This means startups focusing on application-layer innovations may struggle to find relevance in the long term.
Key Takeaways
- Fundamental Model Innovation Over Engineering Tweaks
- AI startups must prioritize deep foundational model advancements over merely refining existing applications.
- Companies relying solely on application-layer improvements will struggle to stay competitive.
- AI Scaling Laws Are Driving Major Iterations
- The next two to three years will see at least two significant AI breakthroughs, fueled by pre-training and inference scaling laws.
- Research is shifting toward reinforcement learning scaling and test-time scaling to maximize efficiency.
- Young, Agile Teams Drive the Future of AI
- Startups with younger teams are proving to be more innovative, adaptable, and cost-efficient.
- Traditional, large tech experts may slow down decision-making and innovation cycles.
- Transformers Are Not the Only Path Forward
- Alternative architectures beyond Transformers are gaining traction, offering breakthroughs in efficiency and multimodal AI processing.
- ChatGPT-like UI is the Killer App
- LLMs have already reached a point where they can replace most domain-specific applications and agents.
- Future AI applications will likely be direct interfaces to LLMs, reducing the need for specialized agents and tools.
Deep Analysis: The Changing AI Startup Playbook
1. Scaling Laws Are Redefining AI Innovation
Scaling laws have long governed AI development, but recent advancements suggest that merely increasing model size is no longer the best strategy. The AI research community is shifting focus to:
- RL Scaling & Test-time Scaling – Utilizing additional computational power during inference to optimize performance.
- Exploring Non-Transformer Architectures – New AI frameworks promise efficiency gains in processing power, memory, and multimodal capabilities.
With computational costs skyrocketing, startups that find more efficient ways to improve AI capabilities will dominate the market.
2. Foundational Model Innovation: The Key to Market Leadership
Startups that heavily invest in building and improving their own foundational models outperform those focused on application-layer engineering. The case of Talkie overtaking Character.ai demonstrates this: when Character.ai’s founder left to return to Google, the company’s innovation stagnated, whereas Talkie’s commitment to foundational model improvements led to exponential user growth.
This indicates that the future belongs to AI companies that continuously enhance their model architectures, pushing the boundaries of natural language understanding, reasoning, and multimodal AI integration.
3. The Age Factor: Young Minds Drive AI Breakthroughs
In an industry moving at breakneck speed, younger teams have proven to be a major asset. Unlike seasoned professionals who often rely on established methodologies, young engineers and researchers:
- Are more experimental and adaptable to new trends.
- Move quickly from research to implementation, reducing decision-making bottlenecks.
- Challenge traditional AI paradigms, leading to groundbreaking innovations.
DeepSeek exemplifies this trend by assembling a younger, more flexible team that achieved competitive AI performance at a fraction of traditional costs.
4. The Fall of Application-Centric Startups
VCs are increasingly skeptical of startups that simply build applications on top of large language models . The belief that “engineering polish” alone can differentiate a product is becoming obsolete. Instead, startups focusing on proprietary foundational model improvements are the ones securing investment and market dominance.
Moreover, with ChatGPT-like UI becoming the standard interface for AI, domain-specific applications and agents will likely disappear. LLMs will natively handle most workflows, making traditional AI applications redundant. For AI entrepreneurs, this means shifting focus from short-term product iterations to long-term, fundamental AI advancements.
Did You Know?
- Transformers may not be the future of AI – While they have dominated the field, alternative architectures are emerging that may be more efficient and scalable.
- Reinforcement learning is entering a new era – Instead of only being used for training, RL is now being applied during inference, unlocking greater performance gains.
- The average age of AI researchers is dropping – Many of the most groundbreaking AI models are being developed by researchers under 30, reshaping the industry’s workforce dynamics.
- VCs are prioritizing AI model innovation over engineering talent – The era of massive hiring from Big Tech firms may be over as startups realize that fresh, young teams deliver more innovation at lower costs.
- ChatGPT-like UI is making traditional AI applications obsolete – With LLMs becoming increasingly powerful, most domain-specific applications will be redundant in the near future.
Final Thoughts
The AI startup landscape is evolving rapidly, with fundamental model breakthroughs, novel architectures, and young talent emerging as the key drivers of success. Investors are backing startups that push the boundaries of AI research rather than those that merely refine existing technologies.
For AI founders, the message is clear: invest in model innovation, prioritize youthful agility, and embrace technological experimentation. In the next few years, those who adopt this mindset will not just survive but lead the AI revolution.