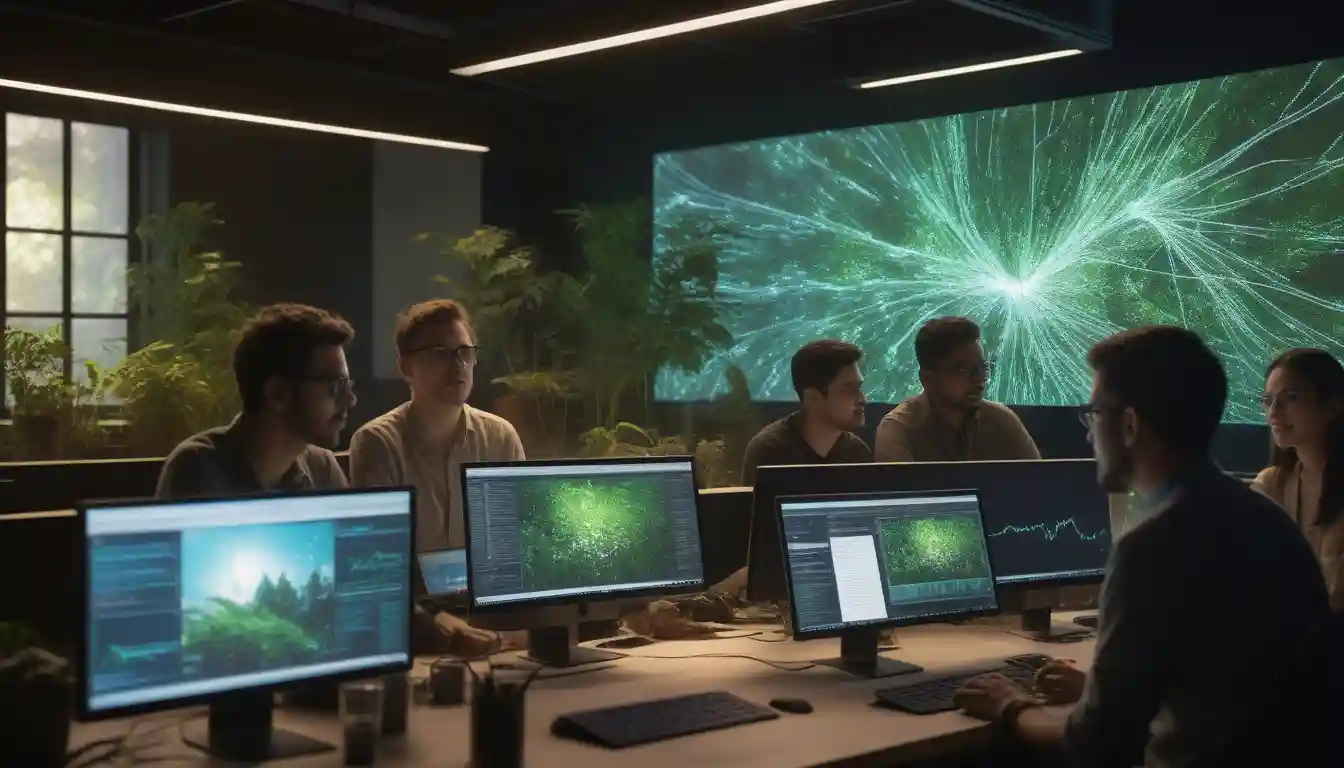
Wikimedia Deutschland's Groundbreaking Collaboration with DataStax and Jina AI to Revolutionize AI Development
Wikimedia Deutschland has joined forces with DataStax and Jina AI to launch a groundbreaking semantic search initiative designed to enhance access to Wikidata's extensive, open-licensed data. This strategic partnership aims to transform the AI development landscape, offering a reliable and freely available information ecosystem. This move challenges the heavy reliance on commercial data sources, paving the way for a more democratized approach to AI development.
Transforming AI with Semantic Vectors
At the core of this project is the transformation of Wikidata's entries into semantic vectors stored in a vector database. This process is expected to significantly reduce AI errors and increase the reliability of large language models (LLMs). Jina AI is at the forefront, providing vector embeddings that convert words and themes into a computer-understandable format. DataStax, on the other hand, manages the vector database, ensuring efficient storage and retrieval of this data.
This innovative approach does more than enhance AI response relevance. By enabling developers to access the most current information, it effectively reduces reliance on outdated training data, a common issue in traditional AI models. The immediate access to up-to-date data allows for more accurate and dependable AI responses, directly addressing the challenge of AI hallucinations and misinformation.
Revolutionizing AI with Open-Source Data
Set to commence in December 2023, this project not only aims to streamline AI development but also to democratize it. By simplifying access to Wikidata's 112 million entries, the initiative is poised to empower AI developers, particularly those working on non-profit, open-source applications. Wikimedia Deutschland is committed to the dissemination of freely available knowledge, and this partnership is a testament to that mission.
Beta tests for the prototype are scheduled for 2025, marking a significant milestone in the journey to provide the open-source generative AI communities with high-quality, validated data. This step promises to bring substantial benefits, including aiding the identification of vandalism in Wikidata and enhancing its use in retrieval-augmented generation (RAG) applications.
DataStax's Role in AI Innovation
DataStax's involvement brings cutting-edge technology into the mix, offering enhancements that make AI application development faster, more flexible, and less dependent on commercial data sources. The recent introduction of Langflow 1.0, a tool that facilitates the comparison of large language model providers, and Vectorize, which integrates top embedding providers through a single API, represent significant strides in the industry. These tools align perfectly with Wikimedia's vision, offering a stable and secure environment for AI applications, particularly those in the open-source sphere.
DataStax's advancements extend beyond this partnership. The integration of vector search capabilities in Astra DB is crucial for generative AI applications, enabling context-based similarity searches that go beyond traditional keyword matching. This feature is instrumental in mitigating AI hallucinations, enhancing the accuracy and relevance of AI responses. Additionally, DataStax's Hyper-Converged Data Platform (HCDP) supports AI workloads across various deployment environments, including cloud and on-premises systems, showcasing a significant shift towards integrating advanced AI capabilities with data management platforms.
Pioneering a New Era in AI Development
This collaboration between Wikimedia Deutschland, DataStax, and Jina AI marks a pivotal moment in AI development, introducing a transformative semantic search concept that has the potential to redefine how AI applications are built and utilized. By making high-quality, validated data more accessible, this initiative not only improves the reliability of AI models but also fosters an open-source ecosystem where innovation can thrive without the constraints of commercial data dependencies.
As the industry moves towards more scalable, secure, and efficient AI development, this partnership sets a new standard for how data management and AI capabilities can be integrated to support a more democratized and reliable information ecosystem. With beta tests on the horizon for 2025, the potential impact on the AI community, especially within open-source generative AI, is immense, promising a future where AI applications are more dependable, accessible, and aligned with the principles of freely available knowledge.
Key Takeaways
- Wikimedia Deutschland collaborates with DataStax and Jina AI to simplify access to Wikidata's 112 million entries, aiming to democratize AI development.
- The project seeks to transform Wikidata's data into a format usable by AI, with the objective of reducing mistakes and enhancing reliability of responses.
- Beta tests for the prototype are planned for 2025, potentially impacting open-source generative AI communities.
Analysis
The partnership endeavors to democratize AI development, disrupting the dominance of large commercial entities in AI by providing a reliable, open-source data alternative. Short-term benefits include improved AI accuracy and reduced reliance on outdated data, while long-term impacts could shape future AI standards and regulations.
Did You Know?
- Semantic Search: This technology improves search accuracy by comprehending the intent and contextual meaning of the search query, transforming data into a format that AI can understand, thus facilitating the retrieval and utilization of information.
- Vector Embeddings: These are mathematical representations of data points that capture semantic relationships, aiding AI models in processing language more effectively.
- Retrieval-Augmented Generation (RAG): This technique enhances generated text quality by integrating traditional language models with a retrieval mechanism, simplifying the access to current and accurate data for AI models.